Growth Strategies Get an Upgrade: AI Use Cases in Pricing, Selling and Incentives
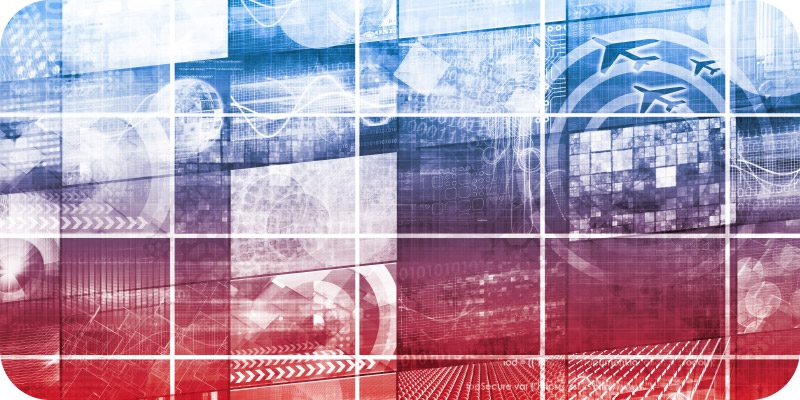
Alex Hoff, the Chief Profit Evangelist at Vendavo, explains how growth strategies are upgrading and shares some AI-centric use cases. This article originally appeared in Insight Jam, an enterprise IT community that enables human conversation on AI.
Manufacturers and distributors are adopting a combination of financial strategies to drive growth in 2025 amid a complicated global economy. They have found higher revenues come with a unified approach to pricing, selling, and rebate management initiatives, but execution isn’t always straightforward. AI brings a new level of intelligence and efficiency to each approach, but most don’t use it yet.
Finance and sales leaders rank AI implementation as their organization’s greatest challenge in the 2024-2025 Top Growth Drivers Report for Manufacturing and Distribution. But it’s also their biggest growth opportunity.
The Need for Buyer Insights
The new study reveals top strategies for driving growth and profitability in 2025 are enhancing channel/partner programs (60 percent), rebate programs (56 percent), price optimization (54 percent), and advanced RevOps tools and processes (54 percent).
Effective execution of each requires in-depth knowledge of buyer sentiment and behavior, but the challenge lies in compiling and analyzing inevitably large data sets from disparate systems. Manually, this requires hours of tedious, error-prone work, and scaling across customer and prospective customer lists is nearly impossible. Adopting purpose-built technology solves some of this heavy lifting, yet 35 percent of manufacturers and distributors say they rarely or never use technology to improve their company’s pricing and incentive strategies, let alone AI.
AI can rapidly and more accurately analyze the data necessary for each strategy, predict future customer actions, and provide timely pricing and selling guidance that maximizes competitive advantage. According to the study, one-third of manufacturers and distributors say they don’t use AI in their price optimization and rebate management efforts or do so only on an ad hoc basis.
Sales, service, and support-driven communication will reveal important customer and partner insights and help strengthen relationships. But there is no substitute for technology-driven fact-finding, automated updates, and AI-powered analysis.
AI Use Cases
How can AI level up growth strategies? Process automation is an essential first step. AI-fueled intelligence with final calculations approved by users is where you gain even more value. Here are some current AI use cases:
- Automated data analysis: AI can process and analyze vast amounts of data from various sources—such as sales transactions, contracts, and invoices—to identify variances and quantify their effects.
- Diagnostic analytics: Within incentive programs, AI algorithms can detect situations where customers are getting large-volume discounts even though they’re buying at medium-volume levels. These customers can be flagged for further review and validation and could even show up as an alert the next time the account manager reviews their contract for renewal.
- Predictive analytics: AI can use historical and commercial telemetry data, and you already have to predict which customers are at risk of decreasing their spending or churning completely.
- Anomaly detection: One of AI’s strengths is its ability to identify outliers and anomalies in data that may indicate a problem. For example, AI can analyze sales data to detect unusual discounting patterns or even changes in revenue or order frequency that may be correlated with past account churn or defections.
Cautions to Consider
AI is uniquely valuable for quickly understanding and extracting large volumes of unstructured or semi-structured data. It also surpasses human capabilities in data analysis and identifying patterns. Generative AI is adept at creating new content that will likely be quickly relevant and helpful. However, predictions can be risky, particularly for financial strategy decisions. With bad data or an improperly trained model, AI can fabricate or hallucinate answers, leading to little understanding of how results were derived or, worse, incorrect or misleading outputs.
Unexplainable black box numbers and false positives in the context of business decisions are risky and have proven to be harmful to business results and the general trust and adoption of technologies in the future. Users must always have the final word.
Finding time to navigate an AI implementation and review the technology’s outputs isn’t easy for busy finance and sales teams. Manufacturers and distributors consider AI their top challenge, but interestingly, the second thing on the challenge list is understanding/meeting customer expectations (14 percent). Imagine how much easier that would be with the help of AI.
A Look Ahead
Despite technology cautions and the added bandwidth required for learning and adopting something new, leveraging AI innovation is a top priority for most C-suites. The upside of driving bottom-line growth with greater efficiency is irresistible.