Security and Governance of Edge AI Devices
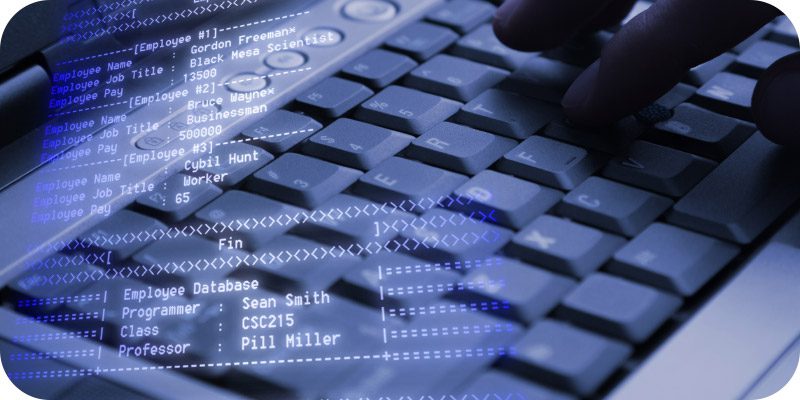
Aparna Achanta, a Principal Security Architect and independent cybersecurity expert, shares her insights into the role security and governance can (and should) play in Edge AI devices. This article originally appeared in Insight Jam, an enterprise IT community that enables human conversation on AI.
Industries relying on real-time data are under growing pressure to deliver speed and efficiency. Traditional cloud computation causes delay, thus making it unsuitable for real-time executing applications. Edge AI revolutionizes real-time analytics by shifting intelligence to edge devices. This eliminates the need for cloud lag times, delivering real-time intelligence where needed.
Consider how this innovation impacts industries like healthcare. In healthcare, patients are monitored by devices with artificial intelligence that continuously monitor vital signs. If an abnormality is found, the device immediately sends an alert, thus removing the delay in sending information to the cloud. This swift response can save lives.
Self-driving automobiles rely on similar real-time decision-making. The cars scan large amounts of sensor data within an instant and make quick decisions to move along the roadway safely to avoid accidents. In high-speed travel, there is not enough time to wait for feedback from a cloud server.
Factories are also being upgraded. With the use of Edge AI, manufacturing lines can detect defects and foresee machine failures in real-time. This system does not rely on servers, as it identifies issues in real-time to avoid the expense incurred during downtime and maintain the continuity of operations. This shift isn’t just about making things faster—it’s about making them more intelligent, efficient, and reliable. Edge AI isn’t the future. It’s already here, changing how industries operate and redefining what real-time means.
The Growing Need for Edge AI
According to a Gartner Report, over 75 percent of enterprise data will be processed outside traditional data centers by 2027. This highlights the need for edge-AI-driven insights since companies recognize that latency from cloud-based systems is increasingly unacceptable in high-speed environments.
The progression in edge deployment of AI models has gathered pace using lightweight neural networks that can directly run on Internet of Things hardware. This approach ensures low-power inference on embedded hardware and high accuracy. With on-device processing, companies can reduce bandwidth costs and improve security by lowering their exposure to cloud computing threats. Edge AI is not just a leap but a fundamental revolution in real-time analytics, hence changing how companies use IoT data to enable faster and better decision-making.
How Does this Affect the Security of IoT Devices
Edge AI is getting more popular, but with this popularity comes bigger safety worries. Instead of having everything in one place like cloud systems, Edge AI spreads data handling and storage far and wide. This difference brings new dangers, some even worse in some ways. Because these devices are everywhere, they can be easy targets for meddling hands and online threats. Many of them are used in places without much control or rules, which only adds to their risk of being attacked.
In simple terms, this means many devices could be physically messed with as they are exposed in locations where they can be stolen or tampered with. This can cause leaks of important information and make networks weak against cyber-attacks. There are also risks from harmful computer programs like malware and ransomware that hackers use to exploit the weaker security measures found in edge systems, leading to a ripple effect of compromising these connected devices.
Stronger Regulations and Governance Strengthen IoT Security
Strengthened regulations and governance frameworks for AI at the Edge are key factors in increasing IoT security. They ensure that data handling on-site adheres to tough compliance standards. Plus, these governance measures can confirm that all AI models set up on edge devices meet audit requirements, aren’t skewed or biased towards any one side, and tie in with ethical notions of artificial intelligence, stopping misuse and avoiding weak points when making decisions.
Adhering to regulations also doubles down on responsibility management while moderating risks tied to rolling out new features in Edge AI. Specific guidelines tailored to every sector help corporations determine the best ways of securing their edge networks and ensure privacy risk management strategies to alleviate risks associated with decentralized information processing techniques. Governance and compliance frameworks call for greater control over who has access to protect against unauthorized access.
The Critical Need for Edge AI
It is a well-known fact that in real-time systems, a slight lag can have serious repercussions. Although cloud analytics can be useful for data storage and processing over a long span of time, in operational environments, the need for timely responses makes server response time a luxury. Thus, Edge AI is seen as a better option for real-time decision-making. An autonomous car, for example, does not have the luxury of sending sensor data to the cloud and then waiting for instructions. It has to process traffic, objects in front, and traffic signals in real-time to navigate and avoid collisions. Local AI processing ensures all decisions—whether to accelerate, brake, or turn lanes—are made in real-time.
Healthcare is another field in which AI can be a lifeline in real-time. Wearable sensors continuously monitor vital signs like heart rate and blood saturation. Unlike sending information to a cloud for processing, such devices can detect real-time anomalies and alert healthcare workers immediately when they occur. This timely response can significantly assist in avoiding serious health complications.