AIOps: Finding Relief from Alert Fatigue
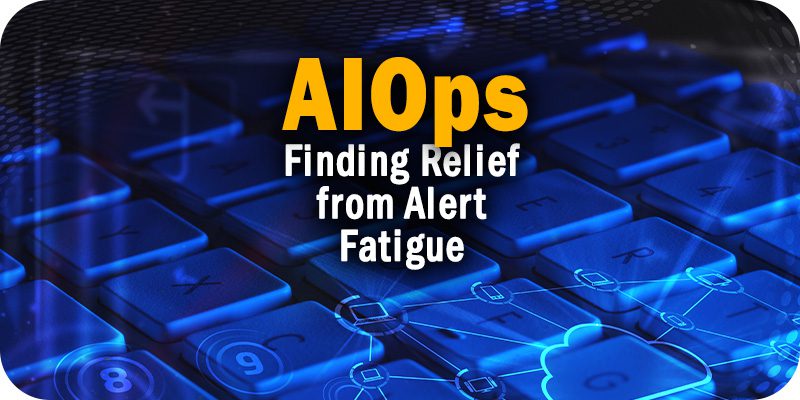
Solutions Review’s Expert Insights Series is a collection of contributed articles written by industry experts in enterprise software categories. Nitin Kumar of Selector examines AIOps network monitoring, and what it takes to find relief from alert fatigue.
When you run a large-scale business infrastructure, diagnosing connectivity problems can feel like trying to find a needle in a haystack. Except it’s not just one haystack, it’s dozens. And the tools you use to search one haystack won’t work for others; each requires a specialized approach. Also, you don’t own many of those haystacks, other people do, and there’s no way to know ahead of time if it’s your job to find the needle or someone else’s. For that matter, most of the time, you don’t know you’re looking for a needle until after it’s found.
Sound frustrating? Then you can understand why so many businesses struggle to maintain reliable, high-performing infrastructures. But the problem goes deeper. In many network operations centers (NOCs), outdated monitoring strategies add to the challenge. Too often, they function as if all that’s needed to uncover problems is more information, and still more, until engineers get flooded with a firehose of data. Understanding which alerts among thousands require further investigation becomes almost impossible. To the point that NOC engineers get “alert fatigue” and start tuning them out.
Now, a new generation of solutions known as Artificial Intelligence for Operations (AIOps) offers a machine learning (ML)-based approach to monitoring and alerting. Algorithmic approaches can be incredibly powerful, turning mountains of telemetry data into actionable insights to help engineers understand, prioritize, and fix problems more quickly. But ML is not magic. If AIOps platforms don’t collect the right data — or correlate the right signals, or surface the right alerts — they can end up perpetuating problems instead of solving them.
In the market for network monitoring solutions? Check out our free Solutions Buyer’s Guide!
AIOps and Finding Relief from Alert Fatigue
Anatomy of an Alert Storm
Modern networks incorporate more components and functions than ever before, making them exponentially more complex. These sprawling, highly dynamic infrastructures enable amazing new capabilities, but they also introduce countless new interdependencies— and new opportunities for connectivity issues to degrade or knock out services. And monitoring approaches that worked in simpler environments just can’t keep up.
The problem isn’t the lack of telemetry data, but the opposite. With so many metrics, logs, and alarms to interpret in real-time, engineers find themselves in an almost impossible position. Somehow, they need to analyze mountains of data while navigating “alert storms” and correlating anomalies. And they need to do it quickly enough to take action before connectivity issues disrupt users and customers. It’s a tall order, requiring engineers to overcome multiple barriers, including:
- Fragmented tools: Organizations typically maintain specialized toolsets to monitor each infrastructure domain (applications, networking, Kubernetes, etc.), and often each vendor within that domain, with each generating its own domain-specific information. As businesses grow, this “tool sprawl” creates a deluge of data. The more information engineers have to synthesize, the more likely they’ll become unable to efficiently parse or prioritize issues.
- Fragmented teams: Organizations themselves also typically fragment across domains. The cloud team monitors the public cloud, the data center team monitors the server fabric, etc., each using specialized tooling. When a service-impacting issue crops up, determining which domain is causing the problem can be a monumental effort. Often, that requires a chaotic scramble to bring all teams together, each with its own toolset and perspective, to try to make sense of the data.
- Siloed data: Modern infrastructures generate vast amounts of telemetry in the form of metrics, logs, and events, and signals can be derived from any of them. But since each must be analyzed in its own way, information often ends up in siloed data stores. Without a way to holistically analyze all data, correlating signals becomes incredibly difficult. And the more an organization grows, the more silos they create.
- Siloed communications: It’s not just data that gets siloed; human insights do too. Solutions to complex issues might never be shared beyond a certain team or Zoom call, even when they might apply to other incidents or domains. Some organizations have spent days trying to diagnose a problem when the solution was already in an email—complete with screenshots and remediation steps—that never went beyond two or three engineers.
As organizations grow, approaches that worked fine with a smaller customer base or simpler environment become increasingly dysfunctional. The results: diminishing visibility, growing frustration, and frequent, expensive fire drills for teams tasked with keeping the business running. And for customers? Unreliable services and poor experiences.
Cutting Through the Noise
Modern AIOps tools can provide real-time analysis and actionable alerting to overcome these issues. After all, detecting patterns across vast datasets is a task that’s tailor-made for ML. Algorithmic analysis can isolate meaningful signals and surface urgent issues in seconds. At the same time, ML tools are only as good as the data they analyze and the knowledge baked into their design. If an AIOps platform misses important metrics or focuses on the wrong signals, it will just provide bad information more quickly.
If you’re evaluating AIOps platforms for your business, look for solutions that:
- Break up data silos: When signals are trapped in data stores, your platform remains blind to them, impeding accurate correlation. AIOps tools should be able to collect all types of data — logs, metrics, alarms — across all vendors and infrastructure areas in your organization, and consolidate them within a common analysis domain.
- Uncover hidden insights: Effective correlation requires deep domain expertise. To separate meaningful signals from noise, ML algorithms must be designed with an exhaustive understanding of each domain under analysis, including each vendor within that domain. At the same time, no third-party tool can go as deep as a vendor’s own instrumentation. AIOps solutions must strike a delicate balance to surface the relevant signals and only those signals— design decisions that only come from extensive technical expertise. Make sure the team behind your AIOps platform has real-world experience with the vendors and tooling in your environment.
- Democratize data: You can’t optimize operations if engineers have to solve the same problems over and over again. An effective AIOps platform should not only provide a cross-domain data repository, but enable cross-team distribution of insights. It should integrate natively into ticketing systems, log management tools, and enterprise communication tools like Slack and Teams, so that information can be shared across the organization.
Effective monitoring can mean the difference between delivering consistently great experiences as your business scales up, or waiting to learn from customers that you’ve outgrown your infrastructure. Make sure you’re giving your infrastructure teams the tools they need to help your business thrive.