2018 Gartner Critical Capabilities for Data Science and Machine Learning Platforms: Key Takeaways
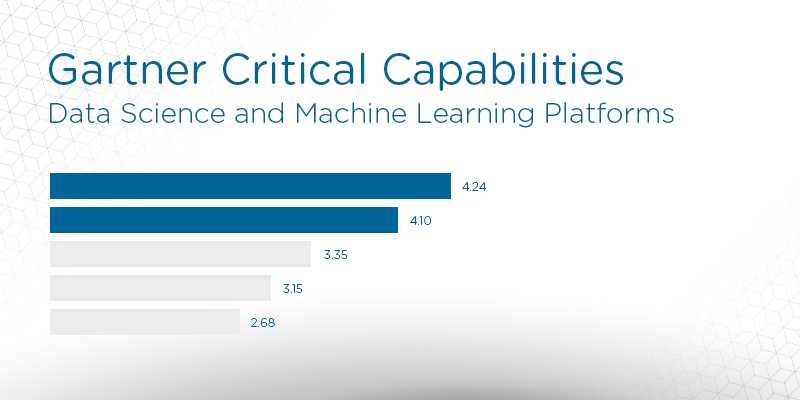
Analyst house Gartner, Inc. recently released its 2018 Critical Capabilities for Data Science and Machine Learning Platforms, a companion resource to the popular Magic Quadrant report. Used in conjunction with the Magic Quadrant, Critical Capabilities is an additional resource which can assist buyers of data management solutions in finding the products that fit best in their organizations.
Gartner defines Critical capabilities as “attributes that differentiate products/services in a class in terms of their quality and performance.” Gartner rates each vendor’s product or service on a five-point (five points being best) scale in terms of how well it delivers each capability. Critical Capabilities reports include comparison graphs for each use case, along with in-depth descriptions of each solution based on the various points of comparison.
The study highlights 17 vendors Gartner considers most significant in this software sector and evaluates them against 15 critical capabilities and three use cases prevalent in the space, including:
- Business exploration
- Advanced prototyping
- Production refinement
The editors at Solutions Review have read the report, available here, and pulled out three key takeaways.
There are a wide range of data science and machine learning providers
Gartner believes there to be more than 70 viable data science and machine learning solution providers, making this a vibrant marketplace. These providers offer cohesive ‘building blocks’ for the development of solutions that are demanded by current conditions. Speaking to the technical capabilities of the 17 providers included in the report, Gartner says: “Each platform is capable of delivering data science solutions into business processes, surrounding infrastructure, applications and products.”
Gartner recommends avoiding long-term commitments
Not only are the solution providers innovating at a rapid pace, but a robust developer community is constantly releasing new open source tools. Gartner recommends paying close attention to these trends, as automation, deep learning and hybrid cloud technologies are pushing the limits of traditional data science frameworks. Organizations would be wise to favor more flexible tools that support and integrate open-source capabilities. However, this requires technological skill that is not widely available in the marketplace.
Data science will soon be a major part of overall analytics spending
As a strategic planning assumption, Gartner believes that predictive and prescriptive analytics will make up roughly 40 percent of enterprises’ spending in BI and analytics by 2020. We’re already seeing the proliferation of more advanced capabilities into ‘modern’ BI and analytics software. Over the last few years, this process has accelerated to the point where data science tools have become commonplace in the enterprise, and more specifically amongst the more forward-thinking solution providers.
Read Gartner’s Critical Capabilities to see how all the top providers scored.