What the AI Impact on Data Analytics Jobs Looks Like Right Now
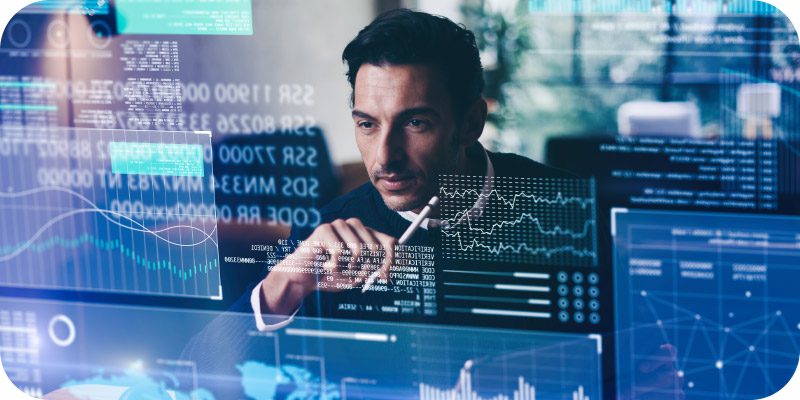
Solutions Review’s Executive Editor Tim King highlights the overarching AI impact on data analytics jobs, to help keep you on-trend during this AI moment.
One of the least surprising things someone can say in 2025 is that artificial intelligence (AI) has impacted data analytics jobs. What is less clear is the specific impact AI has had on those jobs and whether data analysts, data engineers, and business intelligence professionals have cause for concern. As we see AI integrated into data analytics operations at unprecedented levels, the form and function of a company’s data team will inevitably continue changing and evolving.
To keep track of those changes, the Solutions Review editors have outlined some of the primary ways AI has changed data analytics, what analytics professionals can do to remain agile during those changes, and what the future may hold for them and the technologies they use.
Note: These insights were informed through web research using advanced scraping techniques and generative AI tools. Solutions Review editors use a unique multi-prompt approach to employ targeted prompts to extract critical knowledge and optimize content for relevance and utility.
AI Impact on Data Analytics Jobs: How Has AI Changed the Data Analytics Workforce?
In just a few years, the integration of AI into data analytics has dramatically restructured the roles, responsibilities, and required skill sets in the industry. This transformation has been liberating for many, as AI has automated routine reporting, empowered self-service analytics, and shifted teams from rote number crunching to higher-order interpretation and business impact. At the same time, it’s understandable for many professionals to feel a sense of unease about just how fast AI is moving: entire segments of classic analytics work—think ETL scripting, dashboard maintenance, and even exploratory data analysis—are being subsumed by advanced AI agents and copilots. Here are some of the job roles that have been impacted the most by AI:
Data Preparation and Cleansing
Historically, data analysts and engineers spent the bulk of their time cleaning and preparing data for analysis—tasks often viewed as the “janitorial” work of analytics. With the emergence of AI-powered data wrangling tools, a process that could consume up to 80% of a professional’s day is now handled by automated analytic agents that deduplicate, impute missing values, and detect anomalies without human intervention. The upside is clear: more time for analysis, modeling, and communication. The downside? Many entry-level data roles—often the “foot in the door” for aspiring analysts—are at risk of being fully automated out of existence. If you’re early in your career, betting on the future of manual data prep is a losing strategy.
Predictive Modeling and Advanced Analytics
Traditional data scientists once held exclusive domain over predictive modeling and statistical forecasting. Today, LLM-based AI tools and AutoML platforms can build, evaluate, and deploy complex models faster than most teams of humans—and, crucially, without deep coding expertise. What does this mean in practical terms? Data analysts now act more as model stewards and explainers, translating algorithmic output into actionable business insight. Yet, as these tools improve, the gap between “real” data science and automated analytics continues to narrow. This increases productivity and democratizes access, but it also means a growing wedge between those who can interpret AI output (and understand its limitations) and those who simply press buttons. The professionals who thrive will be the ones who combine technical acumen with business domain knowledge and the ability to question AI-driven results.
Reporting and Dashboarding
The legacy model of “analyst as dashboard creator” is under siege. AI copilots now generate real-time dashboards, surface insights with natural language queries, and proactively alert teams to outliers or trends—often before a human even knows to ask. For example, Microsoft Copilot in Power BI or Tableau Pulse can summarize key findings, recommend visualizations, and even generate written executive summaries from raw data. The upside: business users can self-serve, and analysts are freed from repetitive requests. The con: the “middle layer” of dashboard assembly and routine reporting—a career bedrock for thousands—risks becoming obsolete, or at least dramatically less relevant.
A 2024 survey from NewVantage Partners found that 67% of data leaders had already implemented AI copilots for analytics in some form, with 79% reporting significant time savings and increased end-user satisfaction. However, 61% said they’re now struggling to retrain or repurpose analysts whose core tasks have been automated.
Data Governance and Quality
AI also changes the very nature of how organizations manage and govern data. AI-powered data catalogs and observability tools can scan, tag, and assess data quality in real time, reducing the manual burden on data stewards. But as the data landscape gets more complex—and as generative AI creates synthetic or hallucinated data—the demand for professionals who understand not just the mechanics, but the ethics and risks of automated decision-making, grows. Expect a premium on roles focused on AI governance, bias detection, and transparency—especially as regulatory scrutiny tightens.
The Emergence of AI-Centric Data Roles
If the impact of AI on traditional analytics roles is substantial, its effect in creating new, AI-centric jobs is even more dramatic. We’re already seeing demand spike for prompt engineers, AI system trainers, and data product managers—roles that barely existed five years ago. LinkedIn’s 2025 “Jobs on the Rise” report lists AI literacy and prompt engineering among the top ten fastest-growing skills for data professionals, outpacing even classic programming languages.
Yet there’s a twist: as AI tools mature, there’s a real possibility that the “AI specialist” role will be a temporary phase. In the near future, configuring, prompting, and maintaining AI-driven analytics could itself be automated, leaving only the most complex or strategic tasks in human hands. If you’re a data professional betting your future on prompt engineering, recognize this is an arbitrage window, not a permanent moat.
For the next 5-7 years, expect hybrid roles that blend classic analytics skills with AI fluency to be in highest demand. But after that, as generative AI becomes the default layer in every analytics workflow, those who can’t add unique value—whether through domain expertise, critical thinking, or creative insight—will face a far tougher market.
Upskilling for the Future
The need to upskill is not just a cliché for data professionals; it’s an existential imperative. As Christina Inge wrote in her marketing analytics book, “AI might not take your job, but it will be taken by a person who knows how to use AI.” This is doubly true in analytics, where the ability to interrogate an AI’s methods, spot errors in synthetic data, and ensure compliance with new AI regulations will become baseline requirements.
Key skills to focus on now:
-
Algorithmic literacy: Understand how generative AI and AutoML platforms make decisions and where they might fail.
-
Data storytelling: Communicate complex insights in business-friendly language, especially as “raw” analysis is increasingly machine-generated.
-
AI governance and ethics: Master frameworks for data privacy, bias mitigation, and explainability.
-
Domain expertise: Marry technical skill with deep understanding of the business context—the one thing AI cannot automate.
For organizations, treating AI as a mere “feature” is a recipe for stagnation. The best data teams are already becoming learning organizations—places where every professional, from junior analyst to Chief Data Officer, is encouraged to experiment, fail, and adapt as the technology evolves.
AI Will Augment Data Analytics Jobs, Not Replace Them—For Now
The most sophisticated data teams see AI not as a replacement, but as an amplifier of human capability. As Narine Galstian put it for marketers, “To truly harness AI’s potential, professionals must adopt a human-centric approach.” For analytics, this means wielding AI as a tool for scale, speed, and depth—while always retaining a critical eye. AI can spot patterns no human would see, but only a person can ask if those patterns matter, if they’re ethical, or if the data was even valid in the first place.
Let’s be clear: the AI impact on data analytics jobs is a moving target, and its effects will only accelerate. There’s a real risk that mid-tier analytics roles will shrink or vanish entirely, and that the new “bar” will be far higher than it was even five years ago. But for those who embrace the change—who learn to leverage AI, build new skills, and apply critical thinking—the opportunities are enormous.
The bottom line: AI is automating the mundane, but it can’t automate human judgment, curiosity, or business acumen. If you’re a data professional, the safest bet is to treat AI as a partner, not a rival, and to constantly evolve your skillset to stay one step ahead of the machines. The future of analytics isn’t “no humans”—it’s better, more creative humans empowered by the best tools ever invented.