62 Analytics & Data Science Predictions from 35 Experts for 2023
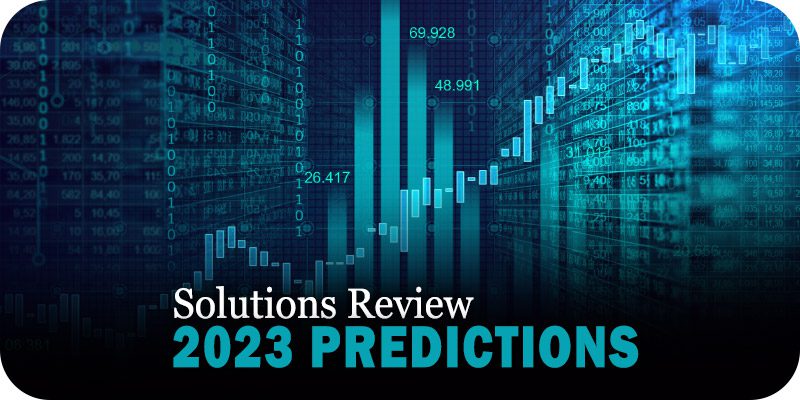
Solutions Review editors received analytics and data science predictions from experts for 2023, part of the 4th-annual BI Insight Jam.
As part of Solutions Review’s third-annual #BIInsightJam, we called for the industry’s best and brightest to share their data protection predictions for 2022. The experts featured here represent the top business intelligence and data analytics providers with experience in this niche. Analytics and data science predictions have been vetted for relevance and ability to add business value as well. These are the best predictions from the dozens we received. We believe these are actionable and may impact a number of verticals, regions, and organization sizes.
Note: Analytics and data science predictions are listed in the order we received them.
Analytics and Data Science Predictions from Experts for 2023
Christian Buckner, SVP Data Analytics and IoT at Altair
The rise of generative AI startups
“Generative artificial intelligence exploded in 2022. In this next year, we will see text processing and visual art using generative AI continue to improve. Entrepreneurs will look to get in on the action (and the $$) and many startups will emerge that create simple experiences for non-technical people based on generative AI. This could range from advertising copy, SQL queries, documentation copy, blog title ideas, code comments, instructional material, and even deepfake video content. Increasingly the creative output from these models is indistinguishable from – and in many cases superior to – human output.”
Rosemary Francis, Chief Scientist at Altair
Deep learning is here
“The next step for artificial intelligence in 2023 is deep learning. While AI so far has mostly been a mix of supervised machine learning and data analytics, the rise of deep learning will usher in a new era where computers are able to learn without supervision. Advancements in deep learning will lead to innovations in robotics, generative AI, natural language processing and speech recognition, and scientific breakthroughs in health, sustainability, and more. Of course, as with any AI models, the key for organizations to ensure the results are accurate and comply with new regulations emerging is to make sure there is still a human element for routine monitoring and trusted accuracy of the ML models.”
Mamdouh Refaat, Data Scientist at Altair
AI and machine learning models should be explainable
“Building AI models is very complex. For AI to really take off, data science and business professionals need to better understand the technology and have access to tools that are easy to use and efficient in building models and gathering data.”
Ryan Welsh, Founder and CEO at Kyndi
The world reached the era of “peak data scientist”
“The shortfall of data scientists and machine learning engineers has always been a bottleneck in companies realizing value from AI. Two things have happened as result: (1) more people have pursued data science degrees and accreditation, increasing the number of data scientists; and (2) vendors have come up with novel ways to minimize the involvement of data scientists in the AI production roll out.
The coincident interference of these two waves yields “peak data scientist”, because with the advent of foundational models, companies can build their own applications on top of these models rather than requiring every company to train their own models from scratch. Less bespoke model training requires fewer data scientists and MLEs at the same time that more are graduating. In 2023, expect the market to react accordingly resulting in data science oversaturation.”
The AI industry will offer more tools that can be operated directly by business users
“Companies have been hiring more and more data scientists and MLEs but net AI adoption in production has not increased at the same rate. While a lot of research and trials are being executed, companies are not benefiting from production AI solutions that can be scaled and managed easily as the business climate evolves.
In the coming year, AI will start to become more democratized such that less technical people can directly leverage tools that abstract all the machine learning complexity. Knowledge workers and citizen “data scientists” without formal training in advanced statistics and/or mathematics will be extracting high-value insights from data using these self-service tools allowing them to perform advanced analytics and solve specific business problems at the speed of the business.”
Ravi Mayuram, CTO at Couchbase
DevOps will adopt blockchain and newer programming languages
“With the emergence of Web 3.0, working with blockchain (specifically Ethereum) will be in every DevOps pro’s playbook—mirroring the boom of adopting machine learning. Newer programming languages, such as Solidity, that are designed for developing smart contracts will enter the toolkits of developers.”
In an increasingly data-centric world, edge computing will fuel the evolution of reliable AI
“AI is ubiquitous in our everyday lives. It suggests what to buy and the news we read. It could determine the emails we receive and augment the cars we drive. Moving forward, AI will be even more embedded in our world. It’ll go through a maturation phase that enables us to rely on it more. Predictability and explainability of AI will improve dramatically as we move forward. Moreover, AI will evolve from being algorithm driven to being more data driven. In order for this to be effective, more and more computation will happen at the edge for AI to be reliable, responsive and cost-effective. This trend of more data influencing the algorithms will determine how AI will evolve to be a tool that is relied upon heavily in this data centric future.”
Zohra Ladha, Senior Director of Data Science at Tredence
Augmented intelligence
“To date, AI and ML have chiefly been used in predicting outcomes and as standalone applications. In the next year, machine learning and natural language processing will both be used to better automate processes and process data while also deriving insights from them as a task that would otherwise be handled by humans, thus increasing workflow efficiencies. Augmented intelligence can transform data analytics with intelligent automation and actionable insights.”
Technology proliferation and personalization through quantum ML
“Experimentation of quantum computing to build more powerful ML models will grow in 2023. With big players like Microsoft and Amazon enabling quantum computing resources via the cloud, this may soon become a reality.”
Technology proliferation and personalization through consolidation of MLOps
“In 2022, enterprise adoption of MLOPs—which deliver scale, speed, and production diagnostics to improve existing models—took shape in a big way. In the coming year, companies are expected to increase their ML budgets fourfold, with a substantial part of it dedicated to MLOps to support enhanced real-time collaboration between teams. While downstream integrations will continue to be a challenge, additional processes and frameworks will be put in place at the initial stage of development to address this issue.”
Finding the right talent (crunch)
“The gap between supply and demand regarding data science talent will continue to widen in 2023. Companies must spend a wealth of time, money, and resources to find the best available data scientists. They should focus on organizing Hackathons, bootcamps, and meetups to target new-age skillsets in AI and data science. Identifying niche skillsets through conventional hiring channels could be challenging. For example, full-stack data science skillsets will now include business domain, machine learning, software engineering, ML engineering, and infrastructure engineering to build end-to-end assets.”
Citizen data scientists
“The one-two punch of the data scientist talent crunch and the increase in no-code/low-code machine learning platforms will strengthen and grow the citizen data scientist community to deliver self-service ML as a business user. Citizen data scientists can bolster organizational value, solve a host of business-specific issues, and deliver meaningful prescriptive analytics.”
Scott Francis, Technology Evangelist at PFU America
AI gets smarter in 2023
“AI will not only grow smarter in 2023, it will become more affordable, simple, and accessible. Companies will automate many processes done manually before, such as invoicing, transcriptions, medical charting, and resume processing. As companies digitize their document archives and historic data, they will use Natural Language Processing (NLP) to make it searchable in real-time. This will increase employee productivity and accelerate the availability of information to business units, delivering richer insights and stronger ROI.”
Companies will leverage AI to shed some light on dark data
“The lack of insight caused by the “black hole” of dark data will continue to plague companies in 2023, including the loss of “tribal” knowledge every time an employee leaves the company. This knowledge gap will lead organizations to leverage AI to classify employee knowledge, making sure everything is captured and searchable, thus enabling team members and new employees to quickly ramp up rather than starting from ground zero.”
Vamsi Paladugu, Senior Director Lyve Cloud Analytics at Seagate Technology
Data analytics are increasingly being asked to drive greater data freedom in the multicloud
“Data freedom is data’s ability to be accessed and to move, and therefore the freedom to deliver insights and the business value that comes from these insights. It means a frictionless flow—unobstructed by structural, economic, and other barriers. One way in which analytics can drive data freedom is via open data lake architectures that leverage open-source frameworks. “
Data democratization will be the number one competitive advantage in 2023
“As companies prepare for economic softening, leaders are leaning on their (often downsized) teams now more than ever. When companies provide teams with unrestricted access to data, employees are empowered to unlock the rich insights deep customer data analysis offers. The companies that can ground individuals throughout the organization in data that helps them to better solve for customer pain points will achieve higher levels of customer satisfaction and retention.”
Daniel Fallman, CEO at Mindbreeze
Technology innovation and investment will not slow down; insight engines will be key
“Even though companies are scaling back given the looming recession, teams are still going to be expected to produce at the same – or even accelerated – pace. Companies need to be prepared to produce the same output, or potentially more, with less human capital. Insight engines will be key to aggregate knowledge and increase efficiency.”
AI-driven question answering will come to the forefront
“It’s what everyone has been waiting for – really making a proper intelligent model to answer questions from inside the organization, with direct extraction of the answer from the source content. Like having a personal assistant, the technology will allow anyone within the organization to ask a question and find the answer from the knowledge base.”
Customization & AI democratization will open doors
“2023 will see a massive boost in roll out of highly specific applications for information insights and apps in low-to-no code fashion to open the door to more use of product among less technically trained individuals. Companies like Mindbreeze are making AI simpler to use so that companies can more easily leverage technology’s capabilities with ease and see more ROI.”
Yali Sassoon, Co-Founder at Snowplow
Data creation as an approach
“One of the most exciting developments in 2022 was the introduction of Data Creation as an approach. This is the process of intentionally creating data to power AI and advanced data applications, as opposed to data exhaust, which is the byproduct of data emitted from existing systems. Data Contracts have been a much-discussed topic in the data community this year. In the context of the Modern Data Stack, this sensible idea has been controversial primarily because practitioners are stuck in the ‘data is oil’ paradigm, in which they assume that data is extracted and not intentionally created. We expect the ‘data product manager’ role and ‘data product management’ skill set to continue to gain traction in 2023. They are instrumental for organizations to successfully treat data as a product, design and implement the right data contracts (as part of data products) and enable them to build out a ‘data mesh.”
Chris Lubasch, Chief Data Officer & RVP DACH at Snowplow
Artificial intelligence
“As much as we love AI, a lot of companies have burned their fingers with huge investments into silo use cases, without seeing their huge, anticipated returns. We will see a shift from ‘AI will solve all my problems; let’s just hire enough data scientists’ to a more thorough approach. AI will still be extremely valuable, but major issues are grounded on the foundations not being ready. Data quality is an issue more often than not. With data cleaning/transforming can take up 80 percent of the data scientist’s time, there is frequently little time left to the real work around AI. More companies will realize that investing in AI isn’t a shortcut to bypass 10 data maturity steps at once.”
Data ethics
“Data Ethics is a topic that’s not yet mainstream, but it should be. With more and more technical capabilities on the rise, in particular, in the field of AI, we need to talk more about how to use data, our algorithms, and our findings in an ethically bearable manner. There’s more than one story of machine-learning trained models that discriminate certain groups of people (e.g. because the training data was already reflecting a certain amount of bias), algorithms denying credits based on questionable correlations, or companies sending out sensitive messages to customers, entering a very delicate field of intimacy and privacy.”
Modern data stack
“It was a year of fast-moving discussions around the modern data stack. Lots of new vendors popped up, and major ones like Snowflake and Databricks continue their journey to take over many technical components, despite the challenging economic situation. But at the same time, voices emerged who questioned the modern data stack as such, whose decoupled approach often leads to many tools and high costs, let alone the complexity of getting it all together. The discussions around the ‘postmodern data stack’ (as just one out of many terms) were started, and we’re all eager to see where this will lead us in the coming years.”
Tech-driven investments
“For years, we’ve seen a lot of siloed and very tech-driven investments in data. Yet, there’s regularly no coherent data strategy in place, that ties all data efforts together. More importantly, it’s absolutely crucial to connect the data strategy properly to the Business Strategy and desired outcomes. Many companies will upgrade their existing strategic and operational efforts to clearly show how data helps to create business value and contribute to concrete goals.”
Chris Gladwin, Co-Founder and CEO at Ocient
Data analysis will be continuous
“Data warehouses are becoming “always on” analytics environments. In the years ahead, the flow of data into and out of data warehouses will be not only faster, but continuous.
Technology strategists have long sought to utilize real-time data for business decision-making, but architectural and system limitations have made that a challenge, if not impossible. Also, consumption-based pricing could make continuous data cost prohibitive.
Increasingly, however, data warehouses and other infrastructure are offering new ways to stream data for real-time applications and use cases.
Popular examples of real-time data in action include stock-ticker feeds, ATM transactions, and interactive games. Now, emerging use cases such as IoT sensor networks, robotic automation, and self-driving vehicles are generating ever more real-time data, which needs to be monitored, analyzed, and utilized.”
Dhruba Borthakur, Co-Founder and CTO at Rockset
2023 will be the year of the data app
“In the past ten years we’ve seen the rise of the web app and the phone app, but 2023 is the year of the data app. Reliable, high performing data applications will prove to be a critical tool for success as businesses seek new solutions to improve customer facing applications and internal business operations. With on-demand data apps like Uber, Lyft and Doordash available at our fingertips, there’s nothing worse for a customer than to be stuck with the spinning wheel of doom and a request not going through. Powered by a foundation of real-time analytics, we will see increased pressure on data applications to not only be real-time, but to be fail safe.”
Real-time data goes mainstream
“In 2022, we saw strong growth of real-time data analytics as more enterprises realized just how valuable it is, which was just a foretaste of its true potential. 2023 is the year that real-time analytics goes mainstream. We are seeing more and more instances of organizations realizing tremendous benefits of real-time spanning across industries. For example, sporting event organizers are using ticket booking data in real-time to adjust prices and local governments are using real-time traffic patterns to dynamically tune traffic signals. With the current bearish market economy, it’s the efficiency and performance of these data systems that will prove to be the key to success. “
Cloud Efficiency Benchmark Wars
“Real-time data analytics has become a necessity in 2023, and the efficiency and performance of these cloud data systems is the key to adoption and success. With the current bearish market economy, every business is feeling the need to reassess the cost of these real-time data analytics systems to better understand price-performance. We are seeing more benchmarks competition from data vendors like Snowflake and Databricks to prove its value to customers, and the data systems that can do more with less are the clear winners. In 2023, we will see benchmark wars between cloud data vendors showing one system being more efficient compared to the other.”
Rise of Real-time Machine Learning
“With all the real-time data being collected, stored, and constantly changing, the demand for real-time ML will be on the rise in 2023. The shortcomings of batch predictions are apparent in the user experience and engagement metrics for recommendation engines, but become more pronounced in the case of online systems that do fraud detection, since catching fraud 3 hours later introduces very high risk for the business. In addition real-time ML is proving to be more efficient both in terms of cost and complexity of ML operations. While some companies are still debating whether there’s value in online inference, those who have already embraced it are seeing the return on their investment and surging ahead of their competitors.”
Haoyuan Li, CEO at Alluxio
More large-scale analytics and AI workloads will be containerized, but the talent pool is a bottleneck
“In the cloud-native era, Kubernetes has become the de facto standard, with a variety of commercial platforms available on the market. Organizations are increasingly deploying large-scale analytics and AI workloads in containerized environments. While containers provide many benefits, the transition to containers is very complex. As a result, in 2023 the main bottleneck to container adoption will be the shortage of talent with the necessary skill set for tools like Kubernetes.”
Big models for AI are driving innovations in specialized infrastructure and solutions
“Over the past few years, AI and deep learning have become mainstream and reached the same maturity level as data analytics. Big models, from OpenAI’s DALL-E 2 image generation model to Google’s LaMDA conversation agent, are expected to dominate the landscape in 2023. Billions of files will be used to train big models for longer periods of time, requiring more specialized infrastructure and solutions. Next-generation AI infrastructure will be developed to handle the scale.”
Demand for simplified data access and data sharing is one on the rise
“Data has become increasingly distributed as the amount of data grows. In 2023, organizations will have an ever-increasing need to manage their scattered data wherever it exists. Furthermore, data sharing across organizations and platforms will become more critical. It will be necessary for organizations to develop and implement a data strategy for managing and sharing distributed data across regions, organizations, clouds and platforms.”
Angel Viña, CEO and Founder at Denodo
Ethical AI becomes paramount as commercial adoption of AI-based decision-making increases
“Companies across industries are accelerating the usage of AI for their data-based decision making. Whether it’s about social media platforms suppressing posts, connecting healthcare professionals with patients, or large wealth management banks granting credits to their end consumers; However, when artificial intelligence decides the end result, currently there is no way to suppress the inherent bias in the algorithm. That is why emerging regulations such as the proposed EU Artificial Intelligence Act, and Canada’s Bill C-27 (which may become the Artificial Intelligence and Data Act if enacted) are starting to put a regulatory framework around the use of AI in commercial organizations. These new regulations classify the risk of AI applications as unacceptable, high, medium, or low risk and prohibit or manage the use of these applications accordingly.
In 2023, Organizations will need to be able to comply with these proposed regulations, including ensuring privacy and data governance, algorithmic transparency, fairness and non-discrimination, accountability, and auditability. With this in mind, organizations have to implement their own frameworks to support ethical AI e.g. guidelines for trustworthy AI, peer review frameworks, and AI Ethics committees. As more and more companies put AI to work, ethical AI is bound to become more important than ever in the coming year.”
Luke Han, CEO at Kyligence
Data & analytics ROI is key metric
“Data and analytics continue to be the focus of IT transformation for many organizations, but as the market evolves–and in an environment of economic uncertainty–most will demand that their investments in analytics show a path to a clear return on investment in the near-term.
Lessons learned during the pandemic and from the experiences of organizations that are already a good distance down the analytics road, show that producing faster, more accurate business intelligence is an attainable goal. That puts an emphasis on production rather than proof-of-concept, and ROI is a key metric for enterprises to consider before adopting any analytics platform or product.”
Analytics as a Service & BYOD
“As the power of analytics becomes more obvious, SaaS analytics applications will emerge as a powerful option to get in on the action, especially for organizations without the human capital or data infrastructure to build and manage their own data operations. Analytics as a Service tools will allow organizations to operationalize their own data on sophisticated tools in the cloud, avoiding costly and time-consuming DevOps cycles.
As a result, Analytics as a Service will further democratize the power of data by delivering a faster time-to-insight while allowing organizations, departments, and even individuals to bring their own data to the application and focus on extracting its value rather than fussing with technology.”
Re-emergence of KPIs
“As more enterprises focus on IT operations and data efficiency, refined management has emerged as an important element of enterprise management. In turn, that has placed an emphasis on measuring and managing performance based on quantitative results. Because of this, key performance indicators (KPIs) will overtake objectives and key results (OKR) as a means of evaluating performance, and for driving decisions at the operations level.
That means goals must be translated and progress tracked using measurable data, including leading metrics that take lag into account so that adjustments can be made in near real-time as performance tracks to established KPIs.”
Dashboards are dead
“Experienced business intelligence and data engineers have long been aware that dashboards–often counted as a business asset–tend to generate a great deal of technical debt that accrues over time, hampering performance. That’s because, for all the ease-of-use glitz and democratization acclaim, dashboards are just dirty tools for connecting data silos.
With enterprises rushing to adopt public cloud applications and services, using business intelligence dashboards for managing processes and reviewing results will create more problems than they solve. But while BI dashboards die off, the metrics store concept will displace them as the preferred method for efficiently managing data on one platform across the entire enterprise.”
AI-augmented analytics will enable democratization
“The democratization of analytics means unskilled data consumers across the enterprise are joining the data party. That’s a great thing… provided your data operations are prepared to manage the chaos.
Using artificial intelligence, data consumers can quickly discover insights like anomalies, root causes, and trends without the skills of a data scientist or engineer, adding value to your organization at every level. The secret to extending analytics to everyone without creating headaches is to augment your organization’s analytics processes with AI to empower users on the front-end, while driving efficiencies on the back-end.”
Balaji Ganesan, Co-Founder and CEO at Privacera
Data analytics
“Adoption of data analytics, privacy and security infrastructure tools assure both a company’s data and that of its customers are safe and data governance also plays a big role. Today’s companies need to be smart, not only about security and investing for long-term growth and business success, but they must adhere to the ever-shifting regulatory environment.”
Brad Smith PhD, Chief Science Officer at meQuilibrium
You can’t measure what you don’t improve
“Data analytics are used by forward-thinking organizations to identify patterns and risks, and ultimately utilize the results in decision-making. In the realm of workplace technology, HR population analytics will continue to grow as a tool for forward-thinking organizations to identify workforce patterns and risks, and then take action to address burnout risk, stress and turnover intent in real time.
Predictive workforce behavioral health population analytics are increasingly essential to successfully operate and grow in uncertain times. With the latest workplace technology, HR and business leaders have on-demand insights about their people. These insights identify the factors contributing to what is making employees feel and perform the way they do, suggest actions to address these needs, and ultimately measure the results.”
Kay Nair, VP of Global Cloud and Strategic Sales at Neo4j
Increased adoption of open-source
“Open-source adoption will accelerate in 2023, as developers and data scientists require more cost-effective solutions to solve existing and new data and analytics problems.”
Peter Wang, Co-Founder and CEO at Anaconda
Machine learning will empower employees to lean further into decision-making roles
“With the new age of work upon us, lingering fears around emerging technologies like artificial intelligence and machine learning will show signs of slowing. Over the last few years, organizations and technologists have placed a concerted effort on educating the majority on how the technology interacts with us in the workplace—the reality being its ability to support decision-making roles. We can expect machine learning to increasingly allow employers to identify areas that can be fully augmented, like transcription, to increase efficiency across organizations. This will be viewed as a positive step forward as we widely embrace machine learning’s ability to minimize busy work rather than fear it will take over, opening the door to furthering employee involvement in more impactful business decisions.”
Stijn Christiaens, Co-Founder and Chief Data Citizen at Collibra
Companies embracing data culture will see the biggest ROI
“As the datasphere continues to explode and companies more clearly understand the value of data, 2023 will be all about the people behind it. In other words, the companies that see the greatest success in 2023 will be those who fully embrace the building of a data culture, giving more people access to data and prioritizing data literacy across their organizations. We will start to see more organizations move towards a decentralized and democratized approach to data.”
Kirk Haslbeck, VP of Data Quality at Collibra
More training makes everyone a data scientist
“As more and more companies begin to treat data as a product, we will start to see an emphasis on data democratization, giving everyone in an organization access to data to help streamline processes. For this reason, companies will start to more formally train employees on data observability, putting a heavy focus on data quality to ensure data is up-to-date and relevant across the organization.”
Lori Witzel, Director of Thought Leadership at TIBCO
“sensing organizations” will outperform “intelligent” ones
“Sensing organizations sense, respond, and seamlessly take advantage of changing conditions using a combination of new technologies including AR and IoT data streams. Since the past is no longer reliably predictive of the future, organizations using only foundational business intelligence (BI) will fall behind. By evolving from foundational BI to a sensing organization, enterprises will gain the agility needed to outperform competitors and yield a massive competitive advantage.”
Data literacy will become essential to accelerating business value
“The struggle to turn data into business value is real, and enterprises that can’t make the shift will lag further behind their competition. Without at least a baseline level of data literacy across business users, investments in robust data management solutions won’t provide full value from enterprise data for the organization. In 2023, data literacy will become essential in accelerating business value for enterprises, and those organizations that embrace data literacy for all business users will have an edge in rapidly changing, competitive markets.”
Ali Ahmed, GM of Enterprise Applications at Clo
2023 will see the shift from traditional business intelligence to modern business intelligence
“The data analytics space is advancing with AI/ML, driving faster decision making and allowing companies to focus on their success through business intelligence. As the implementation of automation increases, business intelligence will be furthered as well. For example, to scale and democratize automation, robotic process automation will evolve into an as-a-service offering, allowing enterprises and small businesses alike to take advantage and automate business processes to move the business forward.”
Shireesh Thota, SVP of Engineering at SingleStore
Embedded AI expands the potential of operational applications
“In 2023, IDC is forecasting, AI spending will go past half-trillion-dollar mark. However, this will only be possible if AI is generally available to customers and organizations across all industries.
One of the most significant barriers to the wide adoption of AI has been the skills gap. Now, AI has become more accessible with the ever-growing number of apps that include AI functionality, like predictive text suggestions. This, combined with the ease of use that stems from the growth of AI capabilities, will bring the utility of AI within reach for all. Operationalizing ML algorithms within databases has been a harder challenge and that will see a change in 2023, and finally don’t worry, GPT-4 is not going to create skynet.”
Jeff Tao, Founder, CEO and Core Developer at TDngine
Open-source digital transformation of traditional industries
“Open source and systems will become even more critical over the next year. In particular, traditional industries like manufacturing in the United States will look to open systems to rebuild infrastructure to become more modern, cost-effective, and globally competitive. Open systems will allow traditional industries not to be locked in by legacy vendors and allow them to be on the pulse of cutting-edge tools and technologies like AI, ML, AR, and more. It will remove the data silos allowing data to be shared easily internally or with outside partners for better analysis.
I predict that there will be two ways that this transformation will happen. First, through embracing the cloud. Cloud-based open systems engineers will be able to share data easier and take full advantage of modern data processing, analytics tools, and the elasticity of the cloud to reduce operating costs. Additionally, democratizing infrastructure by embracing open-source projects will open traditional industries like manufacturing and automation to a larger developer community ecosystem.”
Chris McDermott, VP Platform Engineering at Wallaroo.AI
Businesses will continue to invest in operationalizing their ML models and deploying them at scale
“Just as the DevOps movement revolutionized how system administers managed fleets of thousands of servers using the maxim of “cattle not pets”, AI practitioners will require tools that facilitate experimenting, deploying, observing, and continuously retraining large fleets of ML models in production.”
Edge machine learning will be fastest area of growth in AI
“The cloud continues to provide unparalleled resources and flexibility, but enterprises are seeing the benefits of running machine learning close to the source of the data and where decisioning occurs. This is happening for a variety of reasons such as the need to reduce latency for autonomous equipment; or else to reduce cloud ingest and storage costs by performing computations at the edge; or even due to the lack of connectivity in remote locations like for Oil & Gas or for highly secure systems that can’t be connected to the open internet.”
Marinela Profi, Data Scientist at SAS Software
The generalist data scientist becomes specialized
“Recent focus has been on training data scientists as generalists in coding, algorithm development, and open source on the most dispersed (and sometime with low business value) topics. However, we are seeing that when faced with a real-world business problem, data scientists are lacking industry-specific knowledge – an understanding of the dynamics, trends, challenges of a specific industry, and the ultimate goals that everyone is looking to achieve in that niche market – stalling projects at the onset. In 2023, data scientists who have industry specific knowledge will be the most successful in meeting business demand and expectations and we will see data scientists seek out specialized training.”
Data science democratizes to address skills shortage
“The demand for data scientists has grown but organizations are struggling with finding the best candidates, leading to difficulty in putting models into production and operationalizing AI. At the same time, citizen data scientists are emerging as organizations have recognized that data science is no longer a skill for only the technical few and that everyone should be enabled to work with data comfortably. In 2023, look for organizations to consolidate diverse AI and analytics tools around modern, open, multi-language tools that will increase data science productivity, empower end users to do basic analytics tasks, and allow data scientists to focus on core tasks. By democratizing analytics, more people can join the field.”
Sophie Stalla-Bourdillon, Senior Privacy Counsel and Legal Engineer at Immuta
Cloud-based data analytics become mainstream
“And data-driven organizations free up resources to set up trusted or secure analytics environments to prevent data breaches and mitigate compliance risks. While the COVID-19 pandemic has accelerated the ingestion of healthcare data into secure analytics environments, other verticals will quickly follow a similar path. Organizations at the forefront of this move will build multi-cloud data analytics environments and will have to abstract or federate their governance layer to adequately govern data in multiple locations.”
Nick Elprin, CEO at Domino Data Lab
Early adopters will use hybrid and multi-cloud capabilities to leapfrog competitors
“For global enterprises, achieving breakthrough data science innovation across distributed teams while adhering to growing data governance and privacy regulations may seem impossible. Today’s skyrocketing cloud costs show us that unleashing data science at scale is not feasible without a mix of on-prem and multi-cloud infrastructure, and they’re pushing AI/ML frontrunners to repatriate costly cloud workloads back to the datacenter.
Entering 2023, the result is a rising urgency to give data scientists ubiquitous access to data, compute and reusable code without sacrificing cost efficiency or compliance. Rather than lock-in with a single provider, 2023’s data science standouts will be those who now wisely choose to mix their data center’s AI capabilities with the best ones from each cloud.”
Economic uncertainty will expand the divide between model-driven enterprises and all others
“Every company needs to be model-driven, but most are still “data science dabblers,” treating data science as discretionary projects rather than core business strategy. Without the right talent, tools or infrastructure, data scientists spend too much time on engineering or admin tasks instead of building breakthroughs.
When economic uncertainty tightens budgets, these dabblers will inevitably shut down their data science projects to conserve cash. In 2023 and beyond, this dynamic will significantly widen the gap between those who try and those who succeed as model-driven enterprises.”
Increased opportunities for deep learning will boost demand for GPUs
“The biggest source of improvement in AI has been the deployment of deep learning—and especially transformer models—in training systems, which are meant to mimic the action of a brain’s neurons and the tasks of humans. These breakthroughs require tremendous compute power to analyze vast structured and unstructured data sets. Unlike CPUs, graphics processing units (GPUs) can support the parallel processing that deep learning workloads require. That means in 2023, as more applications founded on deep learning technology emerge to do everything from translating menus to curing disease, demand for GPUs will continue to soar.”
Vishal Singh, Head of Data Products at Starburst
The future is decentralized and agility and collaboration will be key
“Despite attempts to house data in a central location over the past few decades, the reality is that data remains distributed, and it will continue to exist across different regions, clouds, domains, and/or business units. So, how do organizations succeed in an environment where centralized strategies are being advocated for but distributed data is the reality?
In 2023, agility and collaboration will be the keys to successful decentralized data management. The organizations that will succeed will do so by enabling data driven insights to be easily curated and shared across the enterprise. A foundational component of this will be the widespread adoption of the concept of treating data as a product.
Rather than needing to go elsewhere to answer questions they may have about the data they are working with, Data Products provide a self-service component that enables them to fill in the gaps between data creation and consumption. In the coming year, we’ll see more organizations laying these foundations of democratizing data access and/or strengthening their Data Mesh framework through Data Products.”
Chris Gladwin, Co-Founder and CEO at Ocient
Data complexity will increase, while data analysis will be continuous
“Next-generation cloud data warehouses must be versatile—able to support multimodal data natively, to ensure performance and flexibility in the workloads they handle. The need to analyze new and more complex data types, including semi-structured data. Technology strategists have long sought to utilize real-time data for business decision-making, but architectural and system limitations have made that a challenge, if not impossible. Emerging use cases such as IoT sensor networks, robotic automation, and self-driving vehicles are generating ever more real-time data, which needs to be monitored, analyzed, and utilized.”
Clément Stenac, Co-Founder and CTO at Dataiku
2023 will be a year of acceleration for the operationalisation of widespread usage of analytics and ML in all functions of enterprises
“For years, early adopters have already been building out systems to automate a host of mundane tasks and to focus on higher-value activities: this has included everything from financial reporting, to data cleansing and document parsing.
They’ve also combined automation with traditional analytics and AI or ML activities. The benefits can be significant, with companies reporting greater efficiencies and improved quality control, with time to focus on developing the next great ideas and products. Moving on to more profound work also delivers a higher sense of accomplishment: it makes people feel that their job has more value and sense.
All of this together creates a strong incentive for more conservative companies to heavily invest in these practices, which are more often than not accelerated by employees eager for more automation, more analytics, and more insight. When it’s grassroots-driven like this, you get buy-in from across the organization. The success of these initiatives relies on appropriate tooling and standard processes (MLOps, data ops, sometimes called XOps) in order to disseminate such power across organizations, while retaining appropriate controls and governance.”
Iddo Gino, CEO at Rapid
APIs will drive democratization of data
“APIs make it easy to adjust, transform, enrich and consume data – traditionally there was a need for hundreds of highly paid engineers to manage the data and data scientists were needed to understand algorithms. In 2023, we will see a shift towards APIs technologies managing data as a way to gain insights and also control data related costs which means people will no longer need to have highly developed engineering skills to harness the power of data.”
Michael Krause, Data Science Director at Beyond Limits
DALL-E and generative AI in 2023
“Generative models, like the one used to create DALL-E, analyze data and interpolate to create something brand new. But they’re not just good at creating weird art — generative models can be used to discover new materials for battery design, carbon capture, and loads of other innovations.
Generative models will reach new heights in 2023 as solutions like DALL-E are adapted to the younger generation’s desire for video over audio and pictures. We can also expect to see generative models continue to infiltrate the healthcare space for vaccine modeling, drug discovery, and even personalized medicine supported by training data generated from electronic medical records.”