Blurring the Lines Between Data Science and Optimization
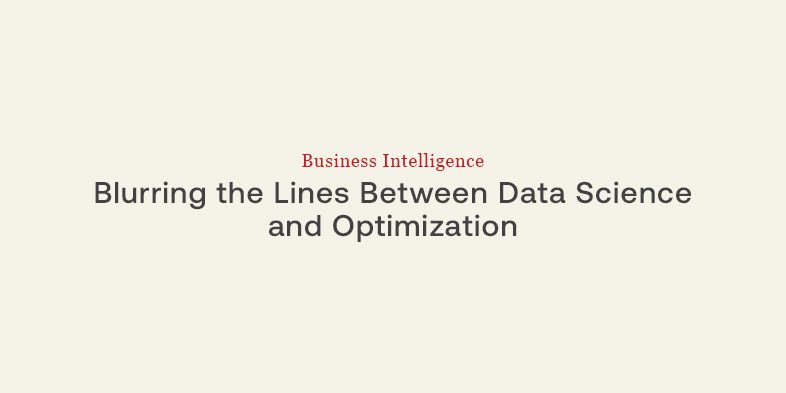
Gurobi Optimization’s Jerry Yurchisin offers insights on blurring the lines between data science and optimization. This article originally appeared on Solutions Review’s Insight Jam, an enterprise IT community enabling the human conversation on AI.
Research suggests that the average person makes somewhere in the ballpark of 33,000-35,000 decisions every single day. Some of these are simple — what shirt should I wear, do I want a hot or iced coffee — but others are much more complex.
Consider, for example, the myriad choices that businesses need to make on a regular basis. What is a reasonable timeline for this project? How can we best appeal to this customer base? How can I use my budget effectively for this effort? These kinds of decisions — ones that can impact your team’s performance and business objectives — are likely the result of more deliberation, discussion, and data analysis than whatever you decided to eat for breakfast.
The more complex the question, the more factors may impact the final decision. When unchecked, these various moving parts can quickly gridlock your team’s capacity to make timely and effective choices. This is why as data sources, analytics tools, and business roles continue to evolve, the future of decision-making is becoming increasingly data-driven.
An Increase in Decision-Making Roles
One way to examine this claim is to observe the types of employees that play a key role in making data-based business decisions at modern organizations. Consider the two following roles that are present across industries:
- Data Scientists: These are problem solvers who leverage statistical models and machine learning (ML) to derive important insights from complex forms of data.
- Operations Researchers: These are problem solvers who leverage various mathematical and analytical techniques like data mining, optimization modeling, simulation, and statistical analysis in order to help businesses make informed and efficient decisions.
On their own, each of these roles is becoming increasingly sought-after by employers. In fact, the U.S. Bureau of Labor Statistics estimates that U.S. employers will need an additional 24,200 operations researchers and an additional 40,500 data scientists by 2031, making these two of the top 30 fastest-growing jobs of this decade.
The roles of data scientists and operations researchers are also starting to become increasingly intertwined. In fact, 55 percent of respondents to one survey noted that they experience operations research and data science team collaboration on a weekly basis. This comes as businesses look for ways to ensure that their decisions are informed from all possible angles.
Combining Decision-Making Technologies
Beyond the closer alignment of decision-making staff, businesses are also up leveling the joint capabilities of their decision-making tools and technologies. Some popular examples of these tools include:
- Machine Learning (ML): A branch of artificial intelligence (AI) that enables computers to ingest, analyze, and learn from data autonomously in order to share predictive insights.
- Mathematical Optimization (MO): A process that leverages a mathematical model of your problem and advanced algorithms to examine complex problems and generate prescriptive solutions.
While ML has flourished in the spotlight of the artificial intelligence boom, 94 percent of survey respondents indicated that MO was gaining traction or remaining steady with decision-makers at their organization. This demonstrates that both ML and MO are crucial to contemporary decision making in their own manner.
Even so, teams are beginning to lean more into the combined capabilities of these tools in order to drive the most informed, prescriptive choices possible. In 2020, less than half of survey respondents (46 percent) claimed that their organization combined ML and MO capabilities. This number has nearly doubled since then, with 81 percent of 2024 survey respondents indicating that their organization combined the capabilities of ML and MO for at least one project. The combination of ML and MO enables decision-makers to automate data analysis, gain predictive insights, and feed these into algorithms that output informed prescriptive suggestions.
What Does This Mean for Your Business?
Growing your ranks of decision-oriented staff and combining capable tools is great, but what does it mean for your company’s decision-making bottom line? By recognizing and acting on opportunities to combine technical and operational capabilities and drive improved decision-making, organizations can:
- Improve Efficiency: Gone are the days of getting bogged down in lengthy strategy discussions or debates. Matching predictive insights from ML with prescriptive solutions from MO helps remove the guesswork from decisions and streamline your processes, making your operations and staff more efficient.
- Optimize Employee Capabilities: Make sure that your skilled employees have the best possible chance to leverage their skills and work cross-functionally to drive improved insights. This can include collaboration between teams and/or upskilling of existing employees to add new capabilities (MO or ML) to their existing training.
- Minimize Costs and Maximize Profits: Improved decision-making can help teams spend less and make more. When fewer resources like time, tools, and employee focus need to be dedicated to analyzing data and deliberating over complex decisions, your team saves on the related costs. Similarly, when company decisions can be made at the speed of the market instead of the speed of internal deliberation, it becomes easier to jump on opportunities and achieve more profit-driving business goals.
A streamlined, efficient, and low-cost business model is ultimately better suited to keep pace with the speed of business and achieve more lasting and concrete results.
The Future of Decision Making
Taking into account the growth of operations research and data scientist roles, their increasing overlap, and the synergistic relationship between ML and MO, it’s clear that businesses are committed to making the most informed and reliable decisions possible.
As teams advance their decision-making capacity, it’s crucial that they understand how each piece of the puzzle — employee capabilities, predictive analytics tools (ML), and prescriptive analytics tools (MO) — can play its critical role in the streamlining of these processes. By continuing to invest in skilled employees and capable tools, these teams will be empowered to solve whichever complex problems come their way.