4 Essential Pillars for a Successful Data as a Product Strategy
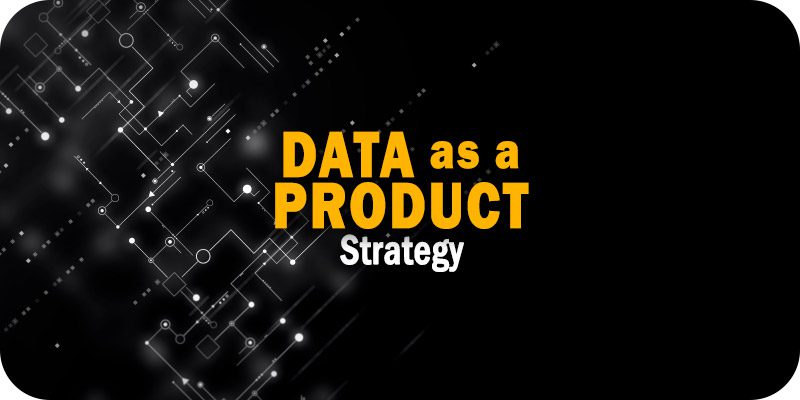
Solutions Review’s Expert Insights Series is a collection of contributed articles written by industry experts in enterprise software categories. In this feature, Starburst Head of Data Products Vishal Singh offers the essential pillars for a successful Data as a Product strategy.
Treating data as a product is at the foundation of modern analytics. With the growth of cloud adoption and the managed service movement, organizations no longer need to consider the complexities of managing software. They can now take advantage of that optionality and focus on the business value of the data. Extracting that value relies on treating data as first-class products or architectural and functional groups of closely related data sets, shortening time to insight and effectively closing the gap between those who have access to and understand the data and those who know the business questions to ask.
It focuses on empowering domains to act as “data producers” servicing the enterprise with “data products.” That’s not data products, as in technology products that use data, but rather data products, as in discoverable, analyzed and curated datasets that inform important and timely business decisions. While this may seem like a complex shift, to make this more attainable and help organizations better benefit from adopting this model, here’s an explanation of the four key pillars of data products and what treating data as a product means for the enterprise.
Data as a Product Strategy
Pillar 1: Data
At its core, a “data product” is just data. This data is curated, analyzed, and combined with business knowledge to inform decisions. Without data, there is no data product. This comes in the form of different files, tables, views, or streams, and it all needs to be identified to ensure that it is the right type of data that will benefit different facets of the organization.
Pillar 2: Metadata
For that data to be useful to an end-user, such as a data analyst, they need to understand the data. This means they need its metadata – columns, definitions, number of rows, refresh patterns, etc. Metadata enrich raw data with context, ultimately shortening the gap in realizing its business value. This also includes access patterns, a specific type of metadata useful in instructing end-users how to query the data and what engine to use.
Metadata helps data consumers and producers to understand how data is being used in the organization and what business context is being driven by the data product. Data consumers can quickly understand the value of insights that data products have already provided, as well as the adoption and success metrics of a data product itself.
Pillar 3: Code
When treating data as a product, it is also important to understand the governance around data policies and utilize APIs or code to enforce the policies, compliance, and provenance. Teams need the proper APIs to access the data, metrics, definitions, and metadata of the data product. They subsequently need code to consume, transform, and best serve the data as a product. A vital component to treating data as a product, code manages and automates these governance policies and ensures teams are abiding by the leading standards without spoiling the agility data provides teams.
Pillar 4: Infrastructure
Finally, the infrastructure used to create the data product is another meta-part of the data product. The essential feature of data products is that they do not require ownership or even approval from a central authority. They thus avoid the agility and scalability problems of centralized data management. However, data products can still be physically stored and accessed in a single software system or across several different systems, as directed by the underlying governance code to maintain global security and tracking of data usage.
When treating data as a product, data product developers and owners aren’t necessarily focused on managing the infrastructure itself but rather on molding the data within it to make it useful and valuable to others. This speaks to the necessity of flexibility and optionality regarding infrastructure. While managing infrastructure may not be the key component of successfully treating data as a product, it is still necessary to have the right infrastructure in place from the start.
It’s the combination of all of these — data, metadata, code, and infrastructure — that make up the data product. By incorporating a unified understanding of and agreement upon what constitutes a data product cross-functionally, businesses can adopt a framework that enables domains to produce data within that structure.
With a data products framework, data producers can trust but also verify the trustworthiness of the data product. That treatment of data as a top-line product of business domains provides a cultural and functional standard across the company, which informs all data producers and consumers that data is a precious commodity. When this principle is executed well, the business is poised to unlock an enormous amount of value from its data – something that will continue to be a necessity for organizations in the future.
Final Thoughts
As organizations continue their data journey, treating data as a product will be a vital component of unifying their data strategy in a highly distributed cloud environment. Understanding these four pillars will help teams make better use of their data at scale and become truly data-driven.