Data Quality Trumps Data Visualization
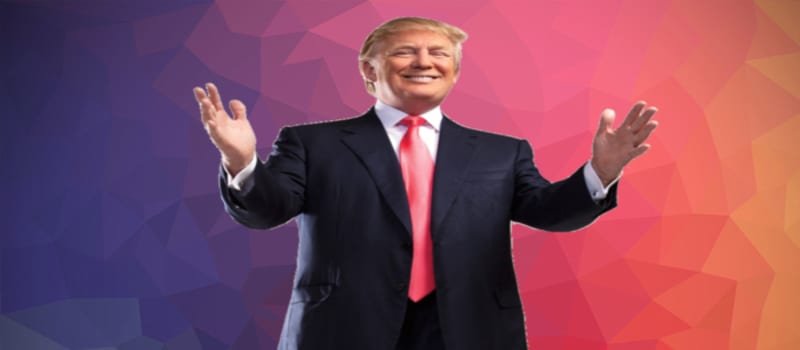
By Raghu Thiagarajan
Data is messy — always has been, always will be. Visualization has become the poster child for addressing complex data problems that could be easily overlooked and simplifies it using design. Patterns or insights may go unnoticed in a data spreadsheet, but if we put the same information on a bubble chart, the insights become obvious. However, it seems that often times data quality is being overlooked in favor of visualization.
With the plethora of data sources that are popping up – structured, unstructured and semi-structured – platforms like Hadoop and NoSQL databases are becoming popular because they can accommodate messiness by not forcing you to normalize everything into predefined data models with consistent dimensions of data. However, this can often delay the problem of data cleanliness. Too often, business analysts arrive at the visualization of their analysis, only to find missing, partial or incorrect information.
Data Aggregation Improves Accuracy
Businesses have lots of data, in lots of formats, in lots of locations. Some of it is in the cloud, some of it is in legacy databases and some of it is in spreadsheets saved to desktops. With so many sources, it’s common that some information is left out, and only partial data makes its way to the final visualization stage. You need to make sure that you are aggregating data from every source. Combined data helps provide context and can lead to insights that wouldn’t be noticed with only limited data access. Indeed, accuracy improves with scale, and trends and exceptions stand out more clearly.
Data Quality at Every Stage
Data quality must be maintained by inspecting for dirty, invalid or inconsistent data at any stage in the complex analytics pipeline. Everything from minor errors to blatant mistakes must be able to be tracked and viewed. For example, if you are importing and analyzing gender and do not check for data quality you may overlook inputs of “M/F” which do not compute. There need to be easy access points and stop checks throughout the entire analytics process so users can make sure that all of the data is clean and going through correctly.
With access to viewing data lineage you can check at any point who was responsible for manipulating data and exactly what they did to it, and in turn maintain data quality throughout. Data quality and consistency are imperative when it comes to ultimately extracting value from Big Data. If at any point in the data pipeline there is a question about data validity, the overall value of the resulting insights is in question.
While some may gloss over the importance of data quality, it is still absolutely crucial to success.
As Big Data tools and solutions aim to be fully enterprise-ready, they must look at combating the data quality issue head on and not wait until the last step. The challenge for analytics tools is to build in data quality protocols, and for end users to think beyond their visualization needs for a solution that tackles the more difficult problems.
Raghu Thiagarajan is responsible for directing Datameer’s Big Data analytics products across the company’s platform, cloud and application portfolio. He has over 20 years of experience in the software industry, and has previously held leadership positions in engineering, product management and product strategy at Sybase, CrossWorlds Software, IBM, Tibco and Hortonworks. He is interested in the convergence of transactional, event-oriented and analytic workloads and easing Big Data consumption for business users. Connect with him on LinkedIn.