Data Science vs. Data Analytics; What’s the Difference?
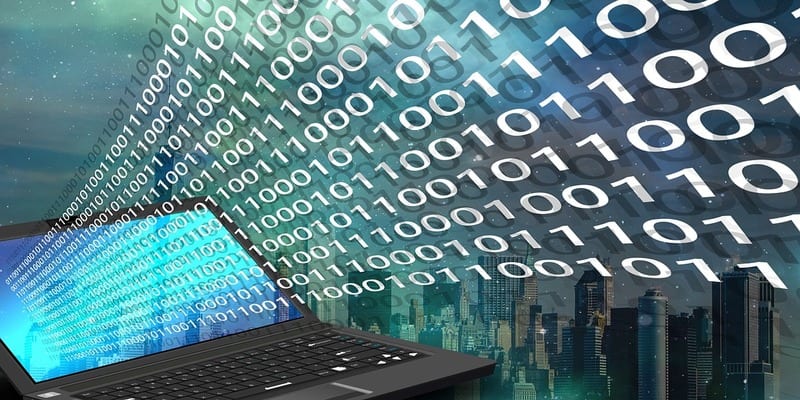
Keeping up with the endless barrage of technology jargon can be a difficult task. Loosely-defined terms and industry-specific vernacular muddy the waters even further. It may seem like a matter of trivialization, but properly defining technology solutions and the data users that handle them has real-world implications.
There is a topical overlap that exists between data analytics and data science. In the same breath, there are also key differences amongst the practitioners of big data in enterprise settings. While data analytics and data science are both important parts of the future of data work, it’s hard to know where one ends and the other begins.
Widget not in any sidebars
That’s where Solutions Review comes in. Our job is to scrub every available inch of relevant information on the web to bring you the leading library of content. It is our hope that these resources help you gain a better understanding of what is becoming an increasingly complex technology environment.
To that end, we’re going to take a deep dive into the world of big data in an attempt to uncover the similarities and differences between data analytics and data science. We’ll also take a look at the business units that perform data analysis. Data workers perform many duties, so defining the strategic impact each has on business is an important task.
Data science
Data science is an umbrella term used to describe how the scientific method can be applied to data in a business setting. In this way, organizations use mathematics, statistics, predictive analytics, and artificial intelligence (including machine learning) to dig into cumbersome data sets in order to reveal trends. Data science is a product of big data through and through, and can be seen as a direct result of increasingly complex data environments.
Data science combines AI-driven tools with advanced analytics. This framework is utilized by data scientists to build connections and plan for the future. Data scientists are largely responsible for formulating overarching questions, not dealing with day-to-day operations. They are also expected to build statistical models, create and work with algorithms, utilize machine learning, and write advanced programming.
Formal training in computer science and statistics is not a must, and this is evident in the rise of the citizen data scientist over the last several years. At the same time, data visualization prowess is an expectation of the job. Data scientists are largely responsible for converting data ‘stories’ into easy-to-digest visuals for business stakeholders. The role is a prestigious one, and data scientists are more likely than their counterparts to lead teams that set the agendas for data projects.
Data analytics
Data analytics is a data science. The purpose of data analytics is to generate insights from data by connecting patterns and trends with organizational goals. Comparing data assets against organizational hypotheses is a common use case of data analytics, and the practice tends to be focused on business and strategy. Data analytics deals less in AI, machine learning, and predictive modeling, and more with viewing historical data in context.
Data analysts are not commonly responsible for building statistical models or deploying machine learning tools. They use basic query expressions (think SQL) to slice and dice data. Data analysts are less likely to be versed in big data settings, and it’s expected that data analysts wrangle data that’s either localized or smaller in footprint.
Its commonplace that data analysts take direction from data scientists, as the former attempts to answer questions posed by the organization as a whole. Data analysts have less freedom in scope and practice, and practice a more focused approach to analyzing data. They’re also much less involved in the culture of data work.
Bottom line
Data science and data analytics are intimately related, but serve different functions in business. Data science plays an increasingly important role in the growth and development of artificial intelligence and machine learning, while data analytics continues to serve as a focused approach to using data in business settings. Organizations utilize analytic tools in slower-moving verticals. Conversely, predictive tools and data science methods are deployed in industries that need access to real-time insight.
The data analyst’s role is business-centric, whereas data scientists tend to work with concepts and ideas. It remains true, however, that both roles are responsible for writing queries, collaborating with engineering departments, source data, and perform various forms of data preparation. Data analytics lives closer to where data resides. The opposite is true for data science and predictive modeling, where the aim is to examine data that lives in disconnected and disparate sources, systems, and applications.
No matter the differences, the two analysis methods provide organizations the ability to learn from data, and the demand for this is huge in today’s marketplace. Data analytics and data science are also two of the hottest career tracks in the big data world. In fact, a recent Glassdoor survey found data science to be the number-one job in America, based on available positions and median starting salary. Harvard Business Review has also referred to data science as ‘the sexiest job of the 21st century‘.