GenAI & AIOps Integration Augments Capabilities, Drives the Move to Autonomous Enterprises
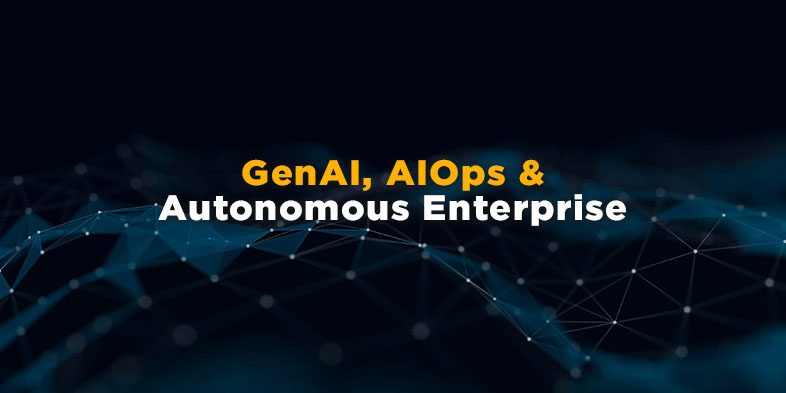
Digitate’s Dr. Maitreya Natu offers insights on how GenAI and AIOps integration augments capabilities and drives the move to autonomous enterprise. This article originally appeared on Solutions Review’s Insight Jam, an enterprise IT community enabling the human conversation on AI.
2023 was truly the year of generative artificial intelligence (GenAI), as its presence permeated the landscape of technology, fueled by its user-friendly interface and its remarkable capacity to rapidly generate high-quality content. As the accessibility of generative AI unlocks new possibilities for creativity through the synergy between technology and human ingenuity, enterprises across industries experimented with GenAI, seeking to boost operational efficiency and provide value to customers.
Defining Generative AI vs. Other Types of AI
GenAI refers to AI algorithms designed to produce novel content by drawing upon existing data, including text, audio, images, and more. These models undergo training on extensive datasets, enabling them to generate innovative content. In contrast to traditional AI, which primarily focuses on utilizing historical data for classification or prediction purposes, GenAI takes a transformative approach.
By way of illustration, consider a straightforward example involving labeled data of cats and dogs, as shown in Figure 1. Traditional AI would answer whether an image depicts a cat, or a dog based on the provided data. In contrast, GenAI elevates its intelligence so that it is capable not only of identifying images but also of creating entirely new images of cats and even crafting narratives to accompany them.
Effective Applications for GenAI
Since its introduction, GenAI has been deployed in applications such as creative content development, where it has emerged as a potent and highly versatile tool for generating imaginative content such as articles, blogs, advertisements, and even songwriting and screenplay development. A decade ago, the idea of AI-generated vocals earning Grammy nominations for artists such as Drake and The Weeknd would have seemed improbable. Yet, as technology advances, users are becoming increasingly innovative in leveraging generative AI in unprecedented ways.
For businesses, one area where GenAI is making waves is to enhance efficiency, especially in the automation of repetitive tasks such as summarizing documents or composing emails. GenAI’s comprehension of programming languages also opens avenues for automatic code generation, where it streamlines basic coding tasks, allowing human experts to focus on more complex scenarios and personalized customizations.
The personalization of user experiences (UX) is a third application where GenAI has taken a foothold. Whether that is through the creation of customized chatbots, advertisements, or personalized emails and notifications, GenAI UX is a significant milestone in the acceptance of AI among end users.
GenAI and AIOps
Within closed-loop autonomous AI Operations (AIOps), there are three established pillars and five areas of activity, as shown in Figure 2. The three pillars laddering up to AIOps are unified observability, closed-loop automation, and intelligent insights. Feeding into those pillars are the five areas of activity: Learning context, managing alerts, handling incidents, performing actions, and optimizing proactively. Introducing GenAI to AIOps enables the development of applications that pair the two, further empowering organizations to accelerate their evolution toward becoming autonomous enterprises. Figure 3 shows how the integration of GenAI can change the game in the five areas of activity. For example, learning context activity can be enhanced with a context-aware “knowledge accelerator” that leverages large language models (LLMs) to extract information from various sources. This enables the creation of a comprehensive enterprise context, gaining a deep understanding of each organization’s unique environment, challenges, and objectives. This context-aware approach allows the AIOps platform to optimize automation through situation and technology-specific actions, delivering tailored and efficient solutions that fuel enterprise agility, resiliency, and innovation, and optimize performance and efficiency.
In addition, looking at the optimize proactively activities, integrated AI-powered virtual assistants have been developed that maximize user experience by leveraging GenAI’s ability to understand language, capture context, and learn from feedback, presenting analytics insights in a much simpler and intuitive way. Guiding users through intricate processes in this way and helping them sift through countless analytics insights enables them to make data-driven decisions with confidence and ease. These are just two applications of course, and can be augmented by others, such as performing code acceleration, and the introduction of collaborative resolution to manage alerts and resolve incidents autonomously.
Advancing AIOps/GenAI Applications
Popular LLMs offer application programming interfaces (API) that emphasize simplicity for all users while allowing customization and fine-tuning in specific domains. The introduction of GenAI can transform the “first mile” and “last mile” of autonomous IT Operations (ITOps), greatly reducing the reliance on human input. Before the integration of GenAI, the first mile required knowledge of the domain, technology, and situation to generate models for automation. This meant significant reliance on natural human intelligence. The last mile required human input to provide guidance in cases of exceptions and also to review insights to proactively optimize operations. This is illustrated in Figure 4.
Applying GenAI advances the first and last mile significantly. As shown in Figure 5, augmenting natural intelligence with, in the first mile, accelerated coding, testing, and domain knowledge, for example. In the last mile, that too is reimagined, with AI-powered virtual assistance leveraging GenAI applications such as intelligent conversation engines, plain language summaries of problems, and collaborative resolution, augmenting natural intelligence to improve ITOps.
Illustrated below are three use cases where GenAI is already making a difference within AIOps:
- Code Generation, Testing, and Documentation: LLMs facilitate efficient code development in collaboration with an AIOps platform’s library of automation operators. Models can proficiently handle multiple programming languages, enabling automated code generation. While LLMs enhance efficiency and accuracy in coding, they require creative prompt engineering for customization and handling complex logic. LLMs are also employed for code documentation and testing, generating test cases in human-readable format and providing detailed documentation.
- Product Q&A: Traditional product assistance is being reimagined using LLMs for intelligent conversation engines. By training LLMs on existing knowledge articles and user guides within AIOps, they can answer user queries with a combination of general understanding and custom knowledge. This approach allows for precise and user-friendly responses, turning LLMs into an effective support tool.
- Deriving Insights through Conversations:By fine-tuning LLMs with AIOps platform data, an intelligent conversation engine can be created for users to access insights seamlessly. This includes analyzing metrics, events, logs, and relationships. The trained model interprets plain language queries, extracting insights such as changes, trends, anomalies, and forecasts. The conversation engine goes beyond answering questions; it learns user preferences, forms viewpoints, and proactively leads conversations, enhancing the overall user experience.
Challenges in Using GenAI
GenAI clearly shows immense potential, prompting business leaders to explore its integration into their operations. That said, the full impact of GenAI models is yet to be realized, and some challenges need to be addressed when using GenAI. Executives remain skeptical about adopting GenAI due to uncertainties surrounding its long-term effects.
One significant challenge arises from the potential for copyright infringement associated with both the input data used to train these models and the generated output. Reproducing or incorporating copyrighted portions from the training dataset without proper authorization poses a considerable infringement risk. It is crucial to acknowledge that the content generated by GenAI may lack accuracy and timeliness. GenAI models often exhibit hallucinations, fabricating information that does not directly align with the provided input.
Another concern revolves around the lack of explainability, traceability, and reproducibility in GenAI outcomes. The models generate outputs without providing explanations of their decision-making process or disclosing the data sources used. This opacity poses a risk of incorrect decision-making.
Despite these challenges, efforts are underway to address them. Restricting the knowledge base of GenAI models to specific custom datasets has proven effective in enhancing reproducibility. Transparent practices, such as detailed recording of data sources, pre-processing steps, and configurations, help establish accountability. Prompt engineering techniques are being creatively employed to minimize hallucinations, while secure ecosystems with well-defined guardrails are being established to foster the responsible development of GenAI.
Critical operationalization aspects for GenAI include:
- Evaluating Suitability: Thoughtfully select use cases, determining the necessity of Generative AI or whether traditional AI solutions are more appropriate. Avoid unnecessary complexity and arbitrary digital interventions.
- Identifying Source Knowledge: Assess data requirements, deciding between generic datasets and specialized custom datasets. Ensure data quality and compliance with legal standards.
- Choosing the Correct GenAI Tool: Choose a model based on considerations like hosting, performance, customization, and privacy requirements. Consider factors such as local hosting, infrastructure needs, and fine-tuning capabilities.
- Crafting Effective Prompts: The engineering of prompts is pivotal for successful GenAI solutions. Experiment with different prompts to constrain responses within specific contexts.
- Evaluating Trustworthiness: Assess the quality of output and establish measures for completeness, correctness, and overall quality. Develop strategies to mitigate errors and consider human-in-the-loop usage, especially for critical applications.
- Weighing Costs and Benefits: Consider the associated costs of GenAI, such as querying Language Model APIs or hosting local models, against potential benefits.
- Integrating Machinery with Generative AI: Embed GenAI into the broader operational process, encompassing data engineering, traditional AI solutions, user experience, last-mile automation, and learning engines.
- Maintaining Human Involvement: Given the evolving nature of GenAI, it is advisable to keep humans in the loop for monitoring, validation, and improvement until the solution matures.
The AI Revolution is Now
There’s no doubt that there’s a pronounced surge of interest and investment in GenAI, revolutionizing the application of technology just as the internet did 30 years ago and social media did, starting in the late 2000s.
Every revolutionary technological advancement requires careful consideration, yet it also warrants exploration and financial support. While there remain uncertainties regarding transparency and trustworthiness, unlocking the genuine potential of GenAI necessitates responsible development, ethical application, and ongoing exploration. The emergence of GenAI brings us to a captivating crossroads, where machine intelligence converges with human creativity. This convergence not only allows us to address the open questions but also empowers us to surpass the limits of what we once thought possible.