5 Key Data Science Questions to Ask Solution Providers for 2021
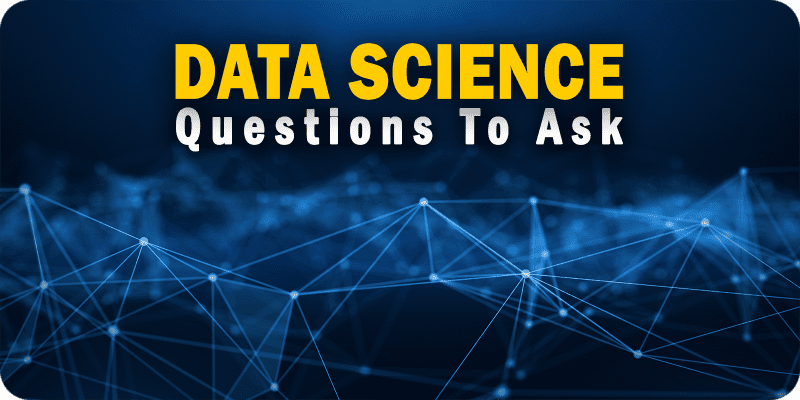
Data science questions to ask solution providers is an annual sneak peak at the top-of-mind concepts to consider during product evaluation. For five key data science questions to ask yourself, consult our Buyer’s Guide for Data Science and Machine Learning Platforms.
Cue the process of seeking out, evaluating, choosing, purchasing, and deploying a data science and machine learning solution. Solutions come in a variety of flavors—ranging from self-service to augmented predictive analytic options. Each features a particular set of capabilities, strengths, and drawbacks. Choosing the right vendor and solution is a complicated process—one that requires in-depth research and often comes down to more than just the solution and its technical capabilities.
To help you evaluate prospective data science and machine learning platforms, these are five data science questions to ask solution providers during product evaluation. If you find these questions helpful, check out our Buyer’s Guide which features five more questions to ask yourself, a comprehensive overview of the market, and full, one-page profiles of the top-16 offerings, as well as our ‘Bottom Line’ analysis.
Who are my data science and machine learning users?
Are your users mainly non-technical business users that require self-service functionality, or experienced data scientists that require advanced tools to do cutting-edge work? The platform or product(s) you ultimately decide to go with should serve all your users as best as possible, or provide training modules to help different employees get up to speed in the shortest possible time.
Which key features are most important?
This will be largely dependent on who your users will be and what your organization already has for other data technologies within its environment. For example, many data science and machine learning tools offer capabilities commonly included in data integration and data management software. If you already have a way of preparing data for example, then you will want to consider that during vendor evaluations.
Do I require augmented data science?
You probably don’t require augmented data science if this is your organization’s first go at evaluating data science and machine learning software. However, if you plan to analyze large data volumes or have expert data science users running a variety of machine learning models, this may be an important consideration.
Is BI and data analytics enough?
Data science and machine learning are popular buzzwords in the technology sphere, and oftentimes organizations bite off a bit more than they can chew. It may be that an advanced analytics tool or platform will work better given you current needs. Don’t buy into the hype to the point where you wind up with a tool that is too complex for your environment or users. If you don’t need specialization in a specific area or for a particular use case, don’t overpay for the functionality.
Do I need a unified platform?
The answer to this question will largely depend on what other data technologies you currently have in your environment. As we’ve noted, there is a great deal of overlap between data science and machine learning platforms and their more traditional BI counterparts. A unified platform is more of an investment and will require buy-in across various departments and users. There are also plenty of great open-source technologies in the space that can help you deal with common data science use cases.