Key Takeaways: 2024 Gartner Magic Quadrant for Data Science and Machine Learning Platforms
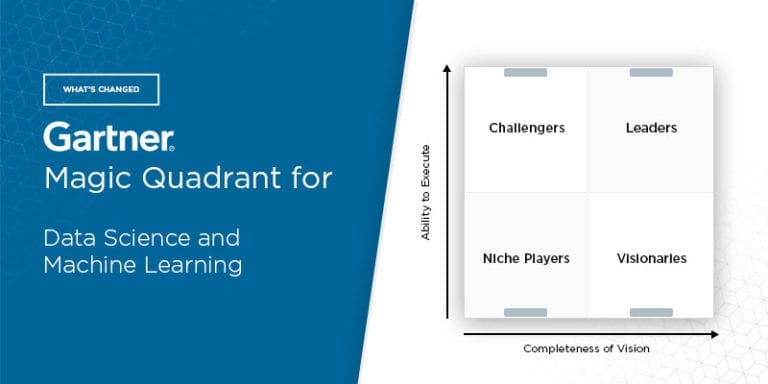
Solutions Review’s Executive Editor Tim King highlights the key takeaways from Gartner’s Magic Quadrant for Data Science and Machine Learning Platforms, providing an analysis of the new report.
Analyst house Gartner, Inc. has released its 2024 Magic Quadrant for Data Science and Machine Learning Platforms. The researcher defines a data science and machine learning platform as “an integrated set of code-based libraries and low-code tooling that support the independent use by, and collaboration between, data scientists and their business and IT counterparts through all stages of the data science life cycle.”
DSML latforms are provided via a desktop app or browser with supporting compute instances and/or as a fully managed cloud offering. They are also being purpose-built these days to, as Gartner puts it: “allow a broad range of users to develop and apply a comprehensive set of predictive and prescriptive analytical techniques.” Included machine learning capabilities vary and range from classic regression and decision trees to much more complex functionality.
In short, enterprises use data science and machine learning platforms to reduce the cycle time and silos typically associated with predictive models. Gartner adds: “They enable collaboration and reuse of assets across multiple teams and departments and orchestration of workloads to handle high volumes of data. They also provide consistent and reproducible training and development environments that provide linkage between data, code, and model assets, enhancing data scientists’ productivity.”
And with recent innovations, low-code, and natural language toolsets enable non-data scientist line-of-business users to do similar work to a trained engineer. Model building, low-code, notebook-based interface, and support for MLOps are all what Gartner refers to as “standard capabilities” while optional ones include automated recommendations, advanced UI toggles, custom SDKs, and GenAI integration.
Innovation among the vendors in this enterprise software space is in a race to enable success with GenAI. One interesting note Gartner makes in a broad context is: “The trajectory of this market, however, is different due to the dual function of the platforms in relation to GenAI.” Another key factor is this, said well by report analysts: “DSML activities within enterprises have grown outside of centralized, core DSML teams.”
It remains true that many of the vendors in this market partner with one another to “share” functionalities that bring joint customers better business value. Gartner says: “AI and analytics leaders should understand how these platforms complement each other, whether for low-code, code-first, data management or operationalization-based functionality, to provide the best means of delivering value.”
How about waiting for the end of the report to proclaim: “The importance of DSML platforms as a strategic enterprise asset has never been greater. The surge in demand for AI solutions, including GenAI, is at its peak, yet the raw materials of data, models, code, and infrastructure have never been more complex to assemble into trusted, scalable products.” As such, the stakes are high when investing in DSML platforms.
GenAI has, and will continue to, hasten and broaden the trend for democratization of data science in the enterprise. Now, the problem that persists most for leaders in this field, is the management and governance over distributed activities.
Gartner adjusts its evaluation and inclusion criteria for Magic Quadrants as software markets evolve. In this Magic Quadrant, Gartner evaluates the strengths and weaknesses of 20 providers that it considers most significant in the marketplace, and provides readers with a graph (the Magic Quadrant) plotting the vendors based on their ability to execute and completeness of vision. The graph is divided into four quadrants: niche players, challengers, visionaries, and leaders.