Navigating the Pitfalls of Self-Service Analytics: Insights from Qrvey CEO Arman Eshraghi
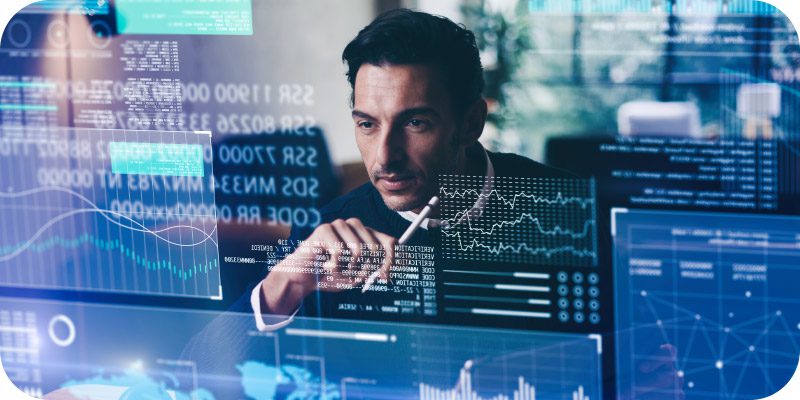
This exclusive Q&A with Arman Eshraghi, CEO of Qrvey, dives into the common mistakes organizations make in self-service analytics and the best practices to avoid them.
Self-service analytics seems like a win-win proposition for business and IT, but it can quickly go awry without sufficient governance, according to international thought leader Wayne Eckerson.
In an article on How to Succeed with Self-Service Analytics, Eckeron says: “Newly empowered data analysts create a multitude of conflicting reports and dashboards with unique business logic and data. The data doesn’t add up when shared, and business users no longer know which report to use.”
He adds: “At organizations awash in conflicting reports, data analysts refuse to trust any except those they create themselves. Also, business users revert to requesting custom reports from IT, or worse, they stop using data altogether to make decisions.”
Likewise, datazuum’s Principle Samir Sharma confirms these self-service analytics in his article Self-Service Analytics: A Value-Driven Perspective for Executives: “Expecting every employee to become a data analyst is unrealistic. While SSA provides powerful tools, many business users need ongoing support and training to derive accurate insights from the data.”
In this guide, Qrvey CEO Arman Eshraghi offers expert insights drawn from his point of view, highlighting how Qrvey’s offerings help organizations harness the full potential of their data. Through a series of questions curated by Solutions Review Executive Editor Tim King, the interview explores Qrvey’s approach to user-friendly analytics, data governance, and the integration of AI to enhance decision-making processes.
This conversation aims to equip leaders and analytics professionals with the knowledge to implement self-service analytics tools effectively, ensuring they add value without compromising on data integrity or security.
For more insights, check out Arman Eshraghi on a recent episode of the Insight Jam Podcast, where he gets into the importance of data visualization, the challenges of AI adoption, and more.
Self-Service Analytics Pitfalls
Question 1: Can you describe Qrvey’s core philosophy regarding self-service analytics? How does this philosophy help your clients avoid common pitfalls?
Answer: In general, people like self-service because it liberates them. For example, think of self-service banking via ATM and mobile phone versus going to a branch. Self-service banking allows people to do it anytime, anywhere, in any way they like without waiting in line for someone to help them. That’s liberty.
Self-service analytics liberates business users to access their information, personalize it in a way that is most intuitive to them, massage their data to make it easier to understand, visually analyze it, automate their data-related tasks, share and collaborate with other people or AI engines, publish their findings, and more – all without waiting for someone to help them. That’s liberty!
Question 2: What are the most common mistakes you see organizations make when they first implement self-service analytics tools?
Answer: Self-service analytics starts with a vision. Every organization should form a vision that is aligned with its goal. For example, a SaaS company’s vision might be to monetize self-service analytics and increase revenue, enrich its product to outshine the competition, or sometimes increase customer retention.
Then, they need to define their strategy, and finally, it comes down to planning and execution.
The lack of a pragmatic approach backed by a clear vision is the most common mistake among self-service implementations.
Another common pitfall in self-service analytics is limiting the scope to charts and visualizations. Without extending self-service capabilities to data and automation, the result will be too limited to be widely adopted by users. In our view, making the data self-service is the prerequisite for having a user-friendly self-service analytics experience.
Question 3: Could you share an example where Qrvey helped a client successfully navigate or correct a significant misstep in their analytics strategy?
Answer: Recently, a SaaS company bought Qrvey to modernize its analytics. They provide financial solutions to nonprofit organizations. Self-service was the main objective for them. About 10 percent of the data were custom-defined fields that were specific to each of the customers. Their traditional analytics was not designed for multi-tenancy, and they could only make the standard set of data available to their customers and users.
How much would people pay for 90 percent of a car? Probably nothing! Using Qrvey they allowed each customer to add their custom-defined data into the data lake without impacting others and the overall data. It made a huge difference and users started to adopt the self-service analytics and benefit from it.
Question 4: How does Qrvey ensure its analytics tools are user-friendly for non-technical users while still powerful enough for data experts?
Answer: We understand that different types of users have different needs. For example, super users or developers need ultimate power and flexibility. Developers love Qrvey’s API-first approach, which applies to data engineers as well as DevOps. These users can leverage all the functionalities through APIs and widgets. For example, DevOps engineers can programmatically deploy content to as many instances and tenants as they wish and automate their processes.
The other group of users are power users; they are spreadsheet wizards, can write formulas, and have a good understanding of the data, but they don’t code. Qrvey offers No-Code and Low-Code environments to these creators. Plenty of capabilities are available to power users with minimal or no coding.
Last but not least are end-users. In general, they tend to be less analytics-savvy than power users. However, end-users vary from organization to organization, and unlike developers and power users, there isn’t a universal and clear definition for all end-users, and so do the self-service capabilities they need. That’s why Qrvey empowers developers and power users to create a sandbox for their end-users. After all, they know their own end-users best, and they can include fewer or more options available in the sandbox. They can even define different groups of end-users and configure different levels of sandboxes.
Another important aspect of being user-friendly to non-technical users is bringing analytics to them instead of pushing them toward analytics. What I mean by that is embedding analytics inside the applications the end-users are familiar with and regularly work with. Making analytics in-app and a natural extension of the applications will help user adoption and simplify usage.
Qrvey does an exceptional job of embedding analytics because it allows all the functionalities, without exception, to be embedded seamlessly and tightly within the web applications with full flexibility for the customizing look and feel in addition to integrating with different data sources and security models.
Question 5: Data governance is crucial in self-service analytics. What are your recommendations for organizations to maintain data integrity and security?
Answer: As organizations gain maturity with their data and analytics, they better understand the importance of data governance.
Qrvey solely serves SaaS product teams by enriching their self-service analytics capabilities to keep their customers happy and highly-engaged. We offer several capabilities to ensure that data is effectively managed, secure, accurate, and accessible throughout its lifecycle.
Each of our customers has a different set of processes for managing data quality, compliance with regulatory requirements, access control, data privacy, and data security. Qrvey acts as a platform and accommodates their preferred style of data governance.
Question 6: What role does training and support play in preventing user errors in self-service analytics platforms? How does Qrvey approach these aspects?
Answer: Qrvey is a train the trainer model – given our use-case for embedding into SaaS product companies. So, we train the SaaS product team, and they create an experience to match their product and customer requirements.
With our background in hundreds of analytics product deployments, we have helped our customers create a phased approach to launching their software that creates the most intuitive end-user experiences. So, from a support standpoint, it starts by creating the best possible self-service UX that can be highly customized to tailor the correct outcomes to match the skill sets of the end users.
Question 7: From your perspective, how important is the customization of analytics tools in preventing user errors and enhancing data-driven decision-making?
Answer: For SaaS companies – customization is an absolute necessity. Each tenant in a multi-tenant SaaS environment may have slightly different analytic requirements. Whether it means they’re operating off of their own custom data assets or they have tenant-specific metrics they need to track – giving users the ability to customize means that you can optimize the value that the analytics offer within your product.
So, customization doesn’t just prevent user error, it means that user experience can be tailored to specific requirements more easily to match the customer data and their outcomes.
Question 8: Can you discuss any upcoming features or innovations at Qrvey that aim to further minimize common mistakes in self-service analytics?
Answer: I think the most important features for this would be Automation and GenAI / AI assisted outcomes
For automation; most application analytics are still heavily reliant on user actions – Log-in, run the report, click around, drag /drop to create and share some additional content with other users, and then go off into some other system and take an action or complete a different task. With the Automation feature, you can not only eliminate most of those steps, but you can also use those same business rules to create repeatable actions that don’t require user interactions or create more user tasks.
Gen AI takes this even further by leveraging AI models to help interpret the data and provide additional insights.
Question 10: What advice would you give SaaS CEOs and Product leaders that want to offer self-service analytics?
Answer: Logically, there are three possible ways to add self-service analytics. The first is to build it. Second, you can buy a tool or technology from a vendor and embed it within your product. This will potentially save you time and money. Before I explain the third option, I should mention that both of these cases are risky and there is no shortage of failed projects in either case. Especially if you are serving multiple groups, customers, or tenants, the complexity of self-service capabilities will multiply, and so will the risk of failure.
The third option – and the one I recommend – is to buy from an OEM partner who can lead you to successfully infuse self-service analytics into your product and business. On the technical side you will need more than just reactive tech support and on the business side you will need well-baked advice to embed it correctly into your sales and marketing machinery.
Final Thoughts
Consider those options from the lens of your vision and goal of offering self-service analytics in your SaaS product. If the goal is to increase retention, outshine the competition, or add new revenue streams, then time to market will be critical.
The third option gets self-service analytics to market quickly and with less risk, and will ultimately set you up for continued success.