The Secret to Augmenting AI-Driven Business Value? Clean Data
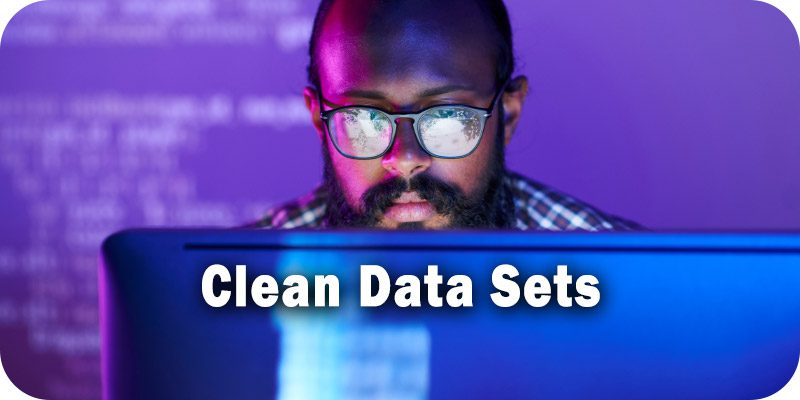
Solutions Review’s Premium Content Series is a collection of contributed articles written by industry experts in enterprise software categories. In this feature, Quantexa Chief Product Officer Dan Higgins offers the secret of augmented AI-driven business value; it’s clean data.
Years of continued global conflict, heightened economic instability, shifting consumer expectations, and accelerate digital transformation have put organizational leaders under magnified pressure to deliver results. But delivering results at speed is getting even harder, and organizations and business leaders alike are under pressure to make faster, more accurate decisions. In a survey conducted by Gartner, 65 percent of respondents noted that they felt they were forced to make significantly more complex decisions today than they were two years ago. Meanwhile, another 53 percent said that they feel more pressure to explain or justify their decision-making – a clear sign of the missing link between rushing to automate and understanding exactly what is being computerized and why.
This is where we often see organizations turn to technologies like artificial intelligence (AI) and machine learning (ML) to support their decision-making. However, the biggest challenge we see is that organizations often implement these technologies without fully considering the importance of context. For AI and ML to make effective predictions and decisions, they need contextual information. It is important to keep in mind that automation challenges stem from more than just complex lines of code; the quality of input data plays a crucial role as well.
Business Value & Clean Data
Solving A Chicken-And-Egg Problem: Data Quality and AI Business Value
Any application of AI and ML will only be as good as the quality of the data that is inputted. This is why to produce higher-performing AI algorithms, data scientists are laser-focused on working with dependable and transparent data. For example, if you build a classifier to distinguish between photos of cubic zirconia and diamonds, data scientists would ideally like an input image dataset certified by a jeweler. If they couldn’t source this, then the obvious next best place to find this might be online. But this is where challenges of entry error and mislabelling come into play.
There is also the challenge of inconsistent data entry, where a single entity may be referred to using different names. For example, if we were to take my name as an example, I Daniel John Higgins may appear as D.J. Higgins, Dan Higgins, Mr. Higgins, or in a like manner. The same applies to businesses, which may be referred to by their full legal name or a shortened version.
It is crucial for the algorithm to be able to recognize and learn from a variety of different names and formats. This becomes particularly challenging when we consider the sheer scale of data and the number of entities with similar names. The challenge is compounded by the number of individuals and organizations that share the same name. Understanding this scenario and its implications is referred to as context. The algorithm must be able to learn from a full range of different names and formats in order to make these sorts of distinctions.
Unlocking the Power of Your Data to Transform Your Business
Fewer than half (42 percent) of global IT decision-makers trust the accuracy of their organization’s data. This according to new research by Quantexa, which also uncovered that one in eight customer records in the U.S. is a duplicate – meaning that a massive number of organizations cannot differentiate between me as D.J Higgins, Dan Higgins and Daniel John Higgins.
Data is crucial to the success of digital transformation initiatives that use data to enhance operational efficiencies, customer value, and to create new avenues for revenue generation. The truth is, we’ve never had more data. So, despite having the potential to act as an organization’s greatest strength, data can also act as the greatest barrier to its transformation efforts.
Recently, enterprises are projected to have invested a staggering $1.3 trillion (USD) towards digital transformation. Unsurprisingly, a whopping 70 percent of these initiatives have fallen short because companies prioritized other technology investments over the data culture necessary to support their intended objectives.
This challenge will only continue to grow as these efforts continue to snowball generating fivefold more datapoints and adding to the complexity problem across industries.
We then find ourselves in a situation whereby in some industries, such as banking and financial services, organizations may fall victim to this data ‘context gap.’ This is as a direct result of having duplicate datasets relating to the same customer spread across various CRM and other management tools and systems. It can be a simple duplication error, but the impact on insight is instrumental. For example, if the customer’s name is spelled with just one letter out of place on one system but not another, there’s a strong chance that the organization will consider these two entries to be two unique entities, even though they refer to the same person. This is the very nature of siloed data. Without context, deploying any sort of significant analysis is next to impossible, stunting the decision-making process altogether.
To gain a 360-degree view of your customers in a scalable way, it requires more than simply combing through archives to try to spot duplicates manually. Manual data management is not only slow and laborious, but also extremely prone to human error. Legacy methods of doing this, such as Master Data Management (MDM), historically have not been effective in identifying these ‘missing links’ and connecting them to an individual customer. To effectively do this, organizations will need to deploy an emerging category of product that does – entity resolution.
Entity Resolution Brings Rich Context into Focus
By leveraging advanced AI and machine learning models, entity resolution effectively connects, standardizes, and parses data to identify like entities in a cohesive manner. This is achieved by grouping related records, thereby creating a set of attributes, and labeled links for each entity. In contrast to the conventional method of record-to-record matching utilized by MDM systems, entity resolution offers organizations the ability to introduce new entity nodes, which act as a crucial connection point for linking real-world data.
This leads to more accurate and efficient data linking, including the ability to match high-value external data sources such as corporate registry information that was previously difficult to link reliably. Quantexa’s same research shows that only 27% of organizations globally currently use entity resolution technology for mastering their data and making informed decisions.
With widespread duplicate data across various databases and data storage systems, entity resolution is critical for decision intelligence, helping companies avoid making decisions based on inaccurate or incomplete data.
All Roads Lead Back to Clean Data
It’s not a secret just how keenly aware organizations are when it comes to the importance of using data to enhance their decision-making capabilities. In order to break down data silos, companies must parse through a bog of duplicate redundant data, which can have a ripple effect on decision-making efficiency
and accuracy. This leads to wasted resources across data, IT, and business teams and hinders a company’s ability to quickly identify risks and provide top-notch customer service. That is why, ultimately, achieving intelligent decision-making all comes down to the foundation of your data.