How Insurers Can Tackle the Challenges of Unstructured Data with AI-Powered Solutions
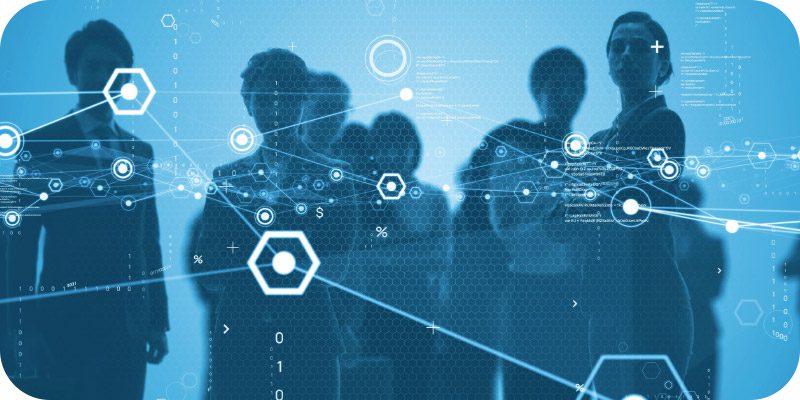
Chaz Perera, the co-founder and Chief Executive Officer (CEO) of Roots Automation, explains how intelligent automation can address the unstructured data challenges insurance companies face. This article originally appeared in Insight Jam, an enterprise IT community that enables human conversation on AI.
Unstructured data—such as the contents of emails, forms, invoices, and contracts, where data is fragmented, siloed, or of insufficient quality—lacks a consistent organization, making it difficult to review and analyze using traditional methods (i.e., human power, robotic process automation, intelligent document processing, optical character recognition). In the insurance industry, critical information is locked in these formats, with much of the work still handled manually by personnel in underwriting and claims.
By leveraging AI-powered intelligent automation, insurers can generate billions of dollars in operational cost savings by enhancing underwriting and claims management efficiency. Faster quoting leads to increased business acquisition, while improved claims handling reduces costs and minimizes claims leakage.
Identifying Data Bottlenecks: A Crucial First Step
To effectively use AI-powered automation, insurers must first identify data bottlenecks hindering efficiency:
- Conduct a Data Audit: Perform a comprehensive audit of data sources and workflows. Identify where data comes from, how it is being processed, and where delays or inefficiencies occur. This will help to map out the entire data flow and spot areas that need improvement.
- Analyze Data Quality: Assess the quality of the data. Look for inconsistencies, inaccuracies, and gaps. Poor data quality can significantly slow down processes and lead to incorrect insights.
- Evaluate Data Accessibility: Determine how easily different departments and systems can access the data. Data silos, where information is isolated within specific departments, create bottlenecks. Ensure that data is stored in a centralized, accessible location.
- Monitor Data Processing Times: Track how long it takes to process data at various stages. Identify steps that take longer than expected and investigate the reasons behind these delays. This could involve manual data entry, outdated software, or inefficient workflows.
- Engage with Stakeholders: Involve key stakeholders from different departments in the identification process. They can provide valuable insights into where they experience data delays or issues.
Addressing these bottlenecks streamlines workflows and enables insurers to leverage AI for improved operational performance.
Unlocking Actionable Insights: Underwriting and Claims Use Cases
AI-powered automation enhances both underwriting and claims management.
6 Ways AI Can Help Underwriting
Use cases where AI-powered intelligent automation empowers underwriting success include:
- Submission Intake and Data Extraction: Intelligent automation can improve speed and accuracy by reading coverage requests, statements of value (SOV), endorsements, and other documents and automatically passing structured data to an underwriting platform. This can accelerate quote times and drive more premium revenue.
- Submissions Triage: Insurers can deploy AI agents to assign submissions and automatically optimize workflow and resource utilization. Using AI in submission processing delivers 99 percent accuracy in a fraction of the time.
- Extract Loss Histories: Insurers using AI experience 80%+ straight-through processing rates for underwriting documents, significantly reducing human effort. AI-powered processing enables faster extraction of claim dates, types, descriptions, costs paid, claim status, payroll history, and other key data from detailed Loss Runs and exposure data reports.
- Request Documents: Streamline underwriting processes and eliminate back-and-forth communication with brokers and agents by using AI to identify missing information based on custom-configured business rules.
- Analyze Risk Thresholds: Automate the classification of submission risks and match them to predefined thresholds—or even decline uninsurable risks based on risk appetite.
- Premium Audits: AI can automate requesting, collecting, and extracting data from employer payroll records, job classifications, 1099 reports, tax forms, and other premium data to increase the speed and accuracy of auditor workflows.
5 Ways AI Can Help Claims Management
Use cases where AI-powered intelligent automation can yield significant gains for claims management include:
- Claims Indexing: Insurers deploying AI to read, understand, and classify demand letters, medical records, and other document types are seeing up to 99 percent improvement in claims indexing accuracy. This reduction in time and human effort offers a fast track to improved capacity during CAT season and other peak demand periods.
- FNOL/FNOI Setup: Another area where you can reduce claims handling time by 90 percent is streamlining First Notice of Loss (FNOL) and First Report of Injury (FROI) processing with AI to automate data capture, coverage verification, duplicate claim lookup, and immediate claim creation in systems of record.
- Surface Legal Demands: AI augments claim adjusters’ capacity by finding and tracking time-sensitive requests buried in multiple-page demand packages. This ensures prompt action to prevent missed deadlines and improve overall compliance.
- Send EOB: AI improves operations, reduces human intervention, and improves the customer experience by automatically forwarding explanations of benefits (EOBs) and policy ID information to claimants.
- Identify Subrogation: Intelligent automation analyzes claim data to help boost profitability by identifying accounts for recovery or subrogation.
Integrating AI into underwriting and claims management offers insurers significant opportunities to enhance efficiency, accuracy, and customer satisfaction. However, it’s crucial to recognize that implementing AI solutions requires substantial investment in technology and expertise.
Implementing Intelligent Automation: Build or Buy
To integrate AI, insurers can:
Build In-House
Developing and maintaining AI models is a substantial investment, often reaching into the millions of dollars. This significant expenditure encompasses various stages, including gathering and preparing data for model training, creating and refining AI models, establishing the necessary computational resources, and implementing regular updates to keep models accurate and relevant.
Training large-scale AI models can require extensive computational resources, leading to high costs. The need for specialized expertise and continuous updates to keep models accurate and relevant further contributes to the overall investment. Modern insurers are tech-driven, but they’re not technology companies. Does the organization have the right mix of people and data resources? If not, consider how to source them. Building and maintaining models and an in-house data store will lengthen the AI project timeline.
Partner with an Insurance solution provider
AI solution providers often have pre-built models and tools tailored for the insurance industry. This means insurers can leverage the provider’s existing technology without starting from scratch, reaching the production stage much faster and with higher-quality results. Providers bring expertise in AI and machine learning and a deep understanding of insurance processes. They also easily align with specific business objectives and grow with the insurer. This ensures that the solutions are technically sound and aligned with industry-specific requirements and compliance.
By partnering with an AI solution provider, insurers can effectively tackle the challenges of unstructured data, streamline operations, and receive actionable insights to improve decision-making and operational efficiency and remain competitive in a rapidly evolving market.
Every piece of information hidden inside unstructured data is an opportunity to enhance underwriting and claims operational efficiency, drive bottom-line results, and delight customers. Success in AI transformation largely depends on meeting the organization’s skills and resource needs. Building insurance AI solutions in-house can yield benefits, but only if the insurer is positioned to make a significant investment in time, talent, and resources. For most businesses, finding a strategic partner is often the straightest and fastest path to ROI from AI.