Harnessing Industry-Specific AI Models to Improve AI Accuracy
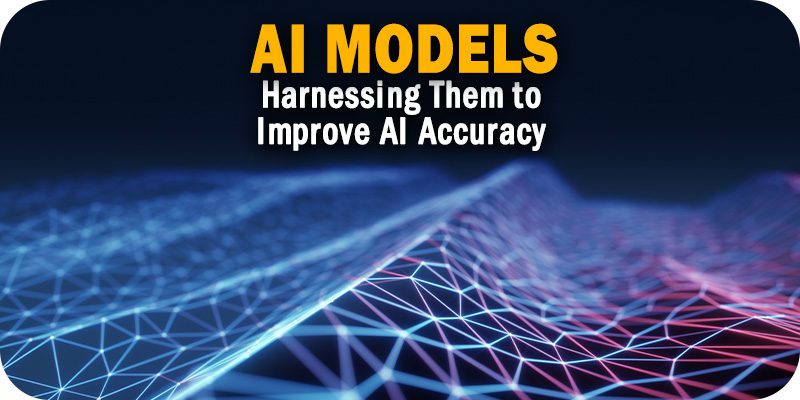
As part of Solutions Review’s Contributed Content Series—a collection of articles written by industry thought leaders in maturing software categories—Assaf Melochna, the President & Co-Founder of Aquant, explains how companies can use industry-specific AI models to improve the accuracy of their AI efforts.
Artificial Intelligence has permeated various industries over the last decade. But powerful open foundational models, like ChatGPT, have recently increased the adoption of the technology, encouraging more business leaders and CIOs to invest and revolutionize how businesses operate. However, as AI systems gain popularity, there is a growing concern about AI hallucinations, where AI models generate inaccurate or misleading outputs. To mitigate these challenges, enterprises and vendors combine generative AI technology with industry-specific AI programs.
Over the next few years, AI is expected to become a commodity with two distinct categories: horizontal and vertical. The former, horizontal, will be more ubiquitous and generalized in its applications (like ChatGPT or Bard AI). And the latter, vertical, will be more specialized and tailored to specific industries (like Service Co-Pilot or 6sense). Eventually, all organizations will need to use some form of AI to stay competitive.
These vertical, purpose-built AI models are a bit more sophisticated and equipped to learn, adapt, and leverage a wide range of data sources, including insights from subject matter experts. When leveraged alongside generative AI models, they can help businesses generate content and automate tasks quickly and accurately. We’ll explore the pivotal role played by industry-specific AI models in mitigating the risks of AI hallucinations, focusing on their learning capabilities, adaptability, data integration, and utilization of expert knowledge.
Learning Over Time
Industry-specific AI models possess the capacity to learn continuously, refining their understanding and performance over time. Unlike traditional models with fixed rules and predefined workflows, these AI programs are designed to evolve through iterative learning processes. By ingesting vast amounts of data, they become adept at identifying patterns, correlations, and contextual nuances specific to their respective industries. This continuous learning enables AI systems to improve accuracy and make more informed predictions.
Adaptability and Real-World Feedback
One key advantage of industry-specific AI models is their ability to adapt and adjust based on real-world feedback and company-specific information. These models are not limited to rigid algorithms or predefined rules but can incorporate new data and insights into their decision-making processes. By embracing feedback from users, customers, and employees, these AI systems can identify areas of improvement and refine their algorithms accordingly. This adaptability ensures that the models remain relevant, up-to-date, and aligned with the dynamic needs of the industry.
Integration of Multiple Data Sources
Hallucinations occur because of the amount and quality of the data leveraged in LLM models. To combat hallucinations effectively, the AI model must consume the appropriate data and information specific to the use case or problem you’re trying to solve. Industry-specific AI models use generative AI to pull information from the correct structured and unstructured data sources to gain a comprehensive understanding of the company’s intricacies.
These AI programs obtain a holistic view of the industry landscape by assimilating data from critical, nonpublic sources such as customer feedback, employee notes, sales records, market trends, and research publications. This integration of data sources helps minimize biases, enhance prediction accuracy, and reduce the risk of hallucinations caused by the abundance of data.
Leveraging Subject Matter Expertise
Recognizing the critical role of human expertise, industry-specific AI models actively seek to convert the knowledge of subject matter experts into actionable data. Aquant’s internal research reveals that incorporating human expertise is indispensable, as 30 percent of solutions to service problems are derived from expert-provided data rather than historical service records.
By tapping into the insights and experience of domain experts, AI models achieve more personalized and reliable results. This collaboration between AI and human expertise helps improve accuracy by grounding the models in real-world understanding, addressing context-specific challenges, and ensuring the AI’s outputs align with industry standards and best practices.
Industry-specific AI programs have emerged as powerful tools to optimize how workforces interact with AI technology and counteract the occurrence of AI hallucinations. ChatGPT and other generative models elevate domain-specific AI by generating easily understood and actionable outputs. Its ability to learn over time, adapt based on real-world feedback, integrate multiple data sources, and leverage subject matter expertise contributes to producing more accurate and reliable outputs. These AI models offer more comprehensive and nuanced results by mining structured and unstructured data and incorporating human insights. As businesses continue to harness AI’s potential, industry-specific AI programs are crucial in ensuring the trustworthy and effective deployment of AI technologies across various sectors.