What the AI Impact on Data Management Jobs Looks Like Right Now
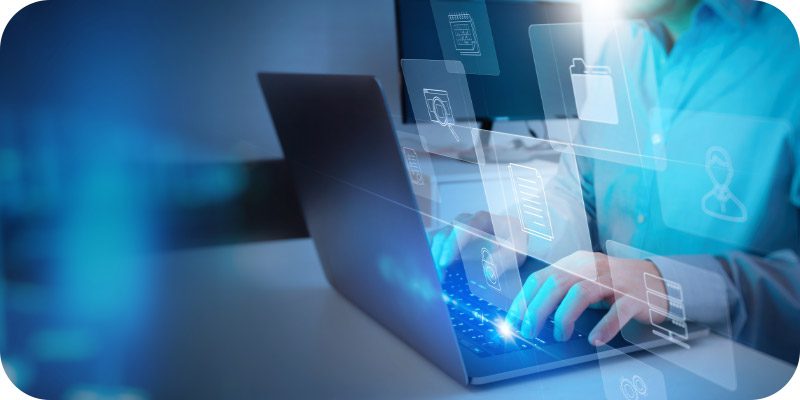
Solutions Review’s Executive Editor Tim King highlights the overarching AI impact on data management jobs, to help keep you on-trend during this AI moment.
One of the least surprising things someone can say in 2025 is that artificial intelligence (AI) has impacted data management jobs. What’s less clear is the specific impact AI has had on those jobs, and whether data management professionals should feel threatened or empowered by the rise of generative AI, LLMs, and automation. With AI being woven into every aspect of how organizations collect, govern, protect, and steward data, the structure and purpose of data management roles are evolving at breakneck speed.
To keep pace with these changes, the Solutions Review editors have mapped out the key ways AI has transformed data management, what data stewards and managers can do to maintain relevance, and what the future may hold for their careers and core responsibilities.
Note: These insights were informed through web research using advanced scraping techniques and generative AI tools. Solutions Review editors use a unique multi-prompt approach to extract targeted knowledge and optimize content for relevance and utility.
AI Impact on Data Management Jobs: How Has AI Changed the Data Management Workforce?
The impact of AI on data management has been both sweeping and paradoxical. AI has taken over huge swathes of tedious manual work—think data cataloging, tagging, lineage tracking, policy enforcement, and metadata management. This has created the freedom for data management pros to focus on strategy and governance rather than grunt work. Yet, it’s also made certain foundational skills and roles—like manual data quality checks, rule-based stewardship, and legacy master data management—less valuable, if not redundant.
Automated Metadata Management and Data Cataloging
For years, data management teams painstakingly cataloged data assets, tagged metadata, and tracked data lineage to enable discoverability, compliance, and trust. Today, AI-driven data catalogs like Collibra, Alation, and Informatica CLAIRE can crawl, classify, and suggest business definitions automatically, keeping pace with data at cloud scale. These platforms not only surface hidden relationships but also recommend privacy tags and policy assignments. The upside: data becomes more discoverable and governed at scale. The downside: the classic “metadata librarian” role—a fixture in traditional IT—has become far less relevant.
Data Quality and Governance
AI-powered data observability platforms now monitor for data drift, outliers, and data integrity issues in real time, auto-generating reports and suggesting remediations. AI agents can trigger corrective actions and even notify stakeholders before flawed data can impact business decisions. This doesn’t mean the human element is obsolete; if anything, oversight becomes more critical, as professionals are needed to interpret, validate, and challenge AI’s judgments—especially as AI can introduce new types of errors, biases, or even hallucinations. Still, entry-level stewardship jobs that revolve around manual review are in decline, and the “craft” of traditional data cleansing is shifting rapidly toward a role of guiding, auditing, and curating AI-driven processes.
Privacy, Security, and Compliance
One of the most profound AI impacts is in privacy and compliance. AI tools now scan for sensitive data, automatically classify PII, and apply (or recommend) policies for GDPR, CCPA, HIPAA, and other regulatory mandates. While this makes compliance faster and more comprehensive, it also means the compliance professional’s job is less about hunting for issues and more about defining ethical frameworks, auditing AI systems, and handling edge cases. AI is good at enforcing rules but still poor at understanding nuance. As regulatory and ethical scrutiny increases, human oversight is more crucial than ever. In the next wave, expect to see new roles like “AI compliance analyst” or “ethical data steward” emerge as organizations grapple with risks unique to machine-driven automation.
Master Data Management and Data Integration
AI-driven MDM tools can reconcile entities, resolve duplicates, and stitch together fragmented records from disparate sources at machine speed. They’re great at recognizing patterns and performing fuzzy matching that would have taken humans weeks or months. But this also means classic MDM administrators—once prized for their meticulousness and tribal system knowledge—are being retooled or redeployed. Instead, the value now is in designing matching strategies, validating results, and integrating MDM processes into AI-augmented data pipelines. The “glue work” that kept MDM viable is dissolving, and those who rely on old-school MDM scripting are facing a shrinking field.
A 2024 IDC report found that 68% of organizations with AI-powered data management platforms reduced time spent on data cataloging and quality tasks by over 50%, while 72% reported improved compliance and audit readiness. But 57% said they were struggling to retrain legacy staff for the new AI-centric tools, and 48% expressed concern about over-reliance on automated recommendations.
The Emergence of AI-Centric Data Management Roles
The decline of some legacy roles is matched by the rise of new, AI-focused positions. Data management teams increasingly need “data product owners,” “AI policy architects,” and “AI trust leads”—people who understand both the technical and ethical dimensions of managing data in an AI-rich environment. LinkedIn’s 2025 “Skills on the Rise” report lists AI governance, explainability, and data privacy as the fastest-growing requirements for data management professionals.
Still, don’t mistake this influx of new roles as permanent. There’s every reason to believe that, as AI gets smarter, even some of these hybrid jobs could become ephemeral. The safest strategy: cultivate deep business acumen, critical judgment, and the ability to question automated outcomes—skills AI can’t easily replicate. The professionals who will thrive are those who can translate between the business, the technologists, and the AI systems, ensuring that automation aligns with organizational values and regulatory boundaries.
Upskilling for the Future
Upskilling is not just advisable—it’s existential. The mantra “learn AI or be replaced by someone who has” is no longer speculative. For data management pros, this means focusing on:
-
AI literacy and tool fluency: Get hands-on with AI-driven governance, cataloging, and data quality tools.
-
Policy, ethics, and regulatory intelligence: Stay ahead of privacy law, bias detection, and automated policy enforcement.
-
Communication and leadership: Guide multidisciplinary teams and bridge the gap between business and technical stakeholders.
-
Critical thinking and oversight: Develop the instincts to question, audit, and intervene in automated processes.
For organizations, data management can’t be seen as a “back-office” or merely IT discipline any longer. The best teams are evolving into cross-functional “data enablement” units that empower self-service analytics, support compliance, and drive value from data assets—powered by AI, but grounded in strong human judgment.
AI Will Augment Data Management Jobs, Not Replace Them—But the Role Is Evolving
Ultimately, AI is automating away the tedious and the routine in data management, but it can’t automate accountability, ethical decision-making, or business alignment. The professionals who are proactive about learning new tools and frameworks, who can interpret and guide AI outputs, and who see the bigger picture will always be in demand. The future for data management is not about clinging to legacy processes, but about becoming the trusted steward and strategic enabler for the AI-powered enterprise.
Bottom line: AI is transforming data management from the ground up, eliminating the old “janitorial” tasks and creating a new class of high-impact, high-responsibility work. To thrive, data management professionals must embrace change, develop AI fluency, and double down on the human skills that machines can’t (yet) replace. The future is wide open—but only for those willing to reinvent themselves.