Building Data Trust: Why Observability Matters
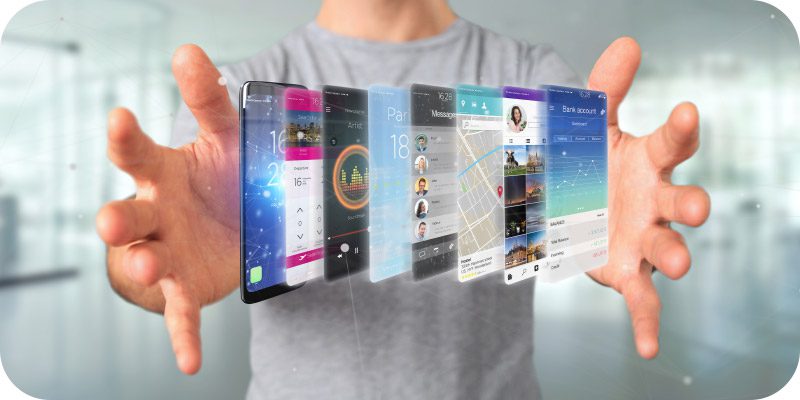
Tredence’s Devang Pandya offers insight on building data trust and why observability matters. This article originally appeared on Solutions Review’s Insight Jam, an enterprise IT community enabling the human conversation on AI.
Modern enterprises have complex data and analytics footprints. They utilize multiple best-in- breed, purpose-built systems to harness data effectively for various use cases across business functions such as marketing, supply chain, finance, and HR.
Further, many companies are leveraging AI/ML to accelerate their business impact and differentiate themselves from the competition. Building effective AI/ML solutions requires trustworthy, high-quality data, which a best-in-class accelerator-led approach can achieve by streamlining and unifying diverse data streams onto one platform. The platform serves as a single source of truth to quickly unlock cross-functional analytics and insights that are the unique drivers of business success.
A quietly expanding challenge that underlies these journeys is the health of the exponentially growing volumes of enterprise data. An earlier study discovered that a mere 3% of companies1 met basic data quality standards. Well-designed DataOps streamline the current data management lifecycle, from source systems to rich insights, addressing the concern to an extent. However, the study’s authors continue to find that2 quality standards have remained the same. Even today, business users come across data that is not fit for purpose during critical moments, from daily forecasting to strategic meetings. Gartner estimates that over the years, the impact on revenue due to poor data quality has ranged from USD 12.9 million3 to USD 15 million4.
Hence, there is a need for data observability frameworks to monitor both the DataOps and the massive data volumes that flow through the enterprise in real-time.
The Pillars of Data Observability
The first, but not necessarily the most important, pillar of observability is data freshness. Are you sure the information captured in your source system flows into your analytics platforms without delay? The next critical pillar is data reliability. Data observability must ensure that the data behind analytics, reports, and dashboards is reliable and accurate on every occasion so business users can depend on it without worry. The third pillar is data completeness. Does the end-user have everything they need for decision-making?
Here is an example of how these pillars effectively triage data quality. Consider a flat file that has to be loaded overnight onto a data lake at a large CPG firm as the input for daily planning. The file may not arrive, it may have too many errors, or it may not have all the information needed, such as data from every market or every product variant. The existing batch data refresh process may not recognize the inconsistencies and deliver reports as usual to business users the next morning. The unusual numbers will throw teams into a tizzy for a few hours or an entire day, scrambling to identify the operational causes. A data observability framework would have proactively flagged the data inconsistencies and initiated workflows to fix them rapidly, reducing anxiety and saving valuable time and money.
The fourth and more subtle and dynamic pillar of observability is data drift. For instance, the lower and upper inventory thresholds for a certain material could shift through business cycles. A good observability tool will deploy self-learning algorithms through AI /ML to determine these changing thresholds and apply them to all the related data sets on the go so they are ready for use.
Running through this entire philosophy is a matter of the cost of data (re) processing, especially on modern cloud data platforms. The primary goal for the business is to have consistent, trustable, and reliable data availability along with optimized cloud compute costs for delivering last-mile adoption of the data insights. A robust observability framework guarantees that your data operations run efficiently, leading to optimized spending across the board. As we have seen, it also minimizes the negative financial impacts of data mismanagement. With companies moving exploding data volumes to multi-cloud environments, the costs of data mismanagement have ballooned, making cost optimization a key consideration.
Building an Observability Strategy
Several tools and solutions in the market provide connectors to monitor your data lifecycle from the source systems and through the complex network of pipelines. Many offer a cost optimization component as well. The build-vs.-buy debate remains. A criterion to keep in mind when choosing a partner for one or a combination of these approaches is to identify which can help you consolidate your observability efforts to avoid further complexity. Also, are they willing to have skin in the game? For example, does the partner bring a degree of accountability that says – we know you have spent a fortune setting up your enterprise data architecture. So don’t pay us now. Let us implement the observability capabilities you need and train your teams. Then, pay us a percentage of the money you save due to streamlined efficiencies and improved strategies. The gain share model is creating a win-win scenario for customers and implementation partners to deliver value quickly.
However, technology alone cannot ensure your data is fit for purpose, irrespective of the use case. This can be achieved when the triumvirate of people, processes, and technology is complete. A checklist for people and processes would look like this: How literate are your core data teams and end-users in the language of data observability? Are best practices for monitoring, logging, and alerting ingrained across the lifecycle? Is your process streamlined from a core deployment perspective? Does your code have guardrails in place to maximize the data quality in your pipelines?
Observability best practices for scientific and business processes have long been around, including for enterprise technology. Data adoption is picking up, which is not surprising. The time to ensure the health of your enterprise data is now.