Close Data Quality Gaps, Minimize Downtime
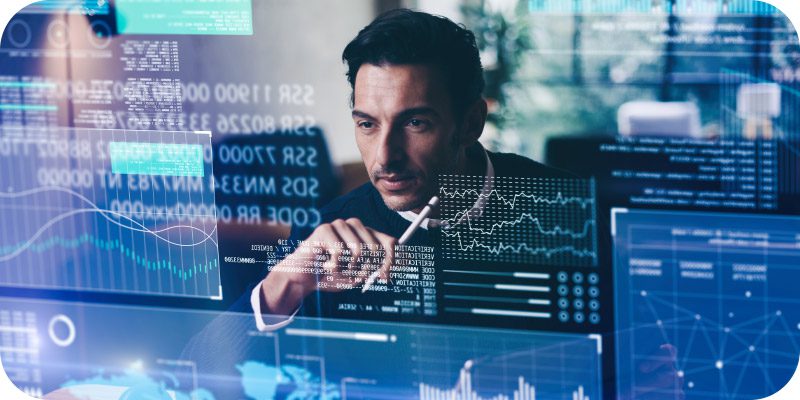
Pantomath’s Somesh Saxena offers insight on closing data quality gaps and minimizing downtime. This article originally appeared on Solutions Review’s Insight Jam, an enterprise IT community enabling the human conversation on AI.
As you’re likely aware, poor data quality costs enterprises millions each year, creating a domino effect that impacts data downtime, decision-making, and resource allocation. These challenges arise because data environments have grown exponentially more complex than the technologies used to manage them.
Data observability has emerged as a modern solution to close this gap. but it only goes so far. Data observability tends to monitor data at rest, while most pipelines are actually filled with data in motion. This leaves room for errors and oversights like data latency and pipeline failure.
Here are some ways your team can close data quality gaps—not just in theory, but in practice—along with some metrics and KPIs you can use to pulse-check whether your data quality methods are actually working.
Strategies and Metrics for Closing the Quality Gap
To tackle data quality gaps head-on and reduce costly downtime, organizations need actionable strategies backed by concrete metrics. It’s not enough to theorize, or unscientifically pursue a haphazard suite of tools in the modern data stack and hope for a miracle.Instead, go into your current setup and figure out where the real issues lie. With this in mind, here are essential strategies for achieving robust data observability.
Pipeline Traceability
Pipeline traceability allows you to view and track data as it moves through your system, from its source to its destination. It’s like having a camera film a birds’ eye view of where your data originates, how it changes, and where it ends up. Through that visibility, pipeline traceability tells the full story of your data, including current weak spots and vulnerabilities. In other words, pipeline traceability is a data quality strategy that tells you how much of the pipeline is being adequately monitored.
Key Metrics:
- End-to-end visibility score:% of data pipeline steps with complete traceability
- Time to identify pipeline bottlenecks: average time to locate performance issues
- Data transformation accuracy: % of correctly tracked data transformations across the pipeline
Operational Observability
When considering comprehensive data quality, it’s essential to incorporate operational observability. This involves monitoring data while it moves in real-time . In dynamic data environments, nothing stays the same for long. Operational observability allows you to gain real-time insight into the health and performance of your data ecosystem. With operational observability, you’re not just looking at static reports—you’re actively watching and understanding your data’s journey and behavior as it happens.
Key Metrics:
- Mean Time to Detect (MTTD): average time to identify operational issues
- Mean Time to Resolve (MTTR): average time to resolve identified issues
- System health score: composite metric of various operational indicators (e.g., latency, throughput, error rates)
Data Validation at Ingestion
Robust data validation at ingestion prevents bad data from entering your systems. Imagine a large e-commerce company that receives product data from multiple suppliers. Each day, thousands of new products are added to the catalog, and existing product information is updated. Without proper validation at ingestion, data errors lead to pricing mistakes, inventory discrepancies, and incorrect product descriptions. Preventing bad data from entering your system will significantly reduce downstream issues and close the data quality gap.
Key Metrics:
- Ingestion error rate: % of records flagged with errors during ingestion
- Data schema compliance: % of incoming data adhering to predefined schemas
- Validation processing time: average time taken to validate each record
Automated Data Profiling
Automated data profiling is the systematic analysis of datasets using specialized software tools. These tools examine data to identify patterns, anomalies, and potential quality issues without manual intervention. The outcomes of this analysis include statistical summaries, structure analyses, and content overviews of data assets, among others. Ongoing profiling enables quick identification of deviations from expected norms. Ideally, teams should maintain a comprehensive understanding of their data landscape, so that deviations raise red flags right away.
Key Metrics:
- Data completeness score: % of required fields populated across datasets
- Anomaly detection rate: % of data points flagged as potential anomalies
- Profile refresh frequency: how often data profiles are updated and analyzed
Continuous Data Testing
A continuous data testing framework acts as a vigilant guardian. By spot-checking for data anomalies, over time you set up a consistent standard of excellence. Data testing is usually performed in a non-production environment before the deployment process kicks off. Testing activities include things like validating schema, columns, triggers, and validations. It transforms your data management from reactive firefighting into proactive quality assurance. Ultimately, you save your teams countless hours of debugging.
Key Metrics:
- Test coverage: % of critical data elements covered by automated tests
- Test pass rate: % of data quality tests passed in each run
- Time to test: average time taken to complete a full suite of data quality tests
Data Quality SLAs and Monitoring
To truly close the quality gap, make “quality” a central focus of your data strategy with clear intent. Establish and monitor data quality Service Level Agreements (SLAs) to align data quality efforts with business objectives and ensure cross-team accountability.
What does this look like in practice? Imagine a global retail chain implements data quality SLAs for its inventory management system, setting a 99.9 percent accuracy target for stock levels across all stores. They establish real-time monitoring alerts for any discrepancies exceeding 0.5 percent and mandate resolution within two hours. After three months, inventory accuracy improves from 97 percent to 99.8 percent, resulting in a 15 percent reduction in stockouts and a 10 percent increase in customer satisfaction.
Key Metrics:
- SLA compliance rate: % of data quality SLAs met over a given period
- Quality incident frequency: number of data quality incidents reported per month
- Time to SLA breach resolution: average time taken to resolve SLA breaches
These strategies and metrics are a good starting point for closing the data quality gap and avoiding data management pitfalls. However, data quality isn’t just a technical challenge—it’s also a cultural one. In a data-driven culture, quality is everyone’s responsibility, from the C-suite to frontline teams.
Looking ahead, the role of artificial intelligence and machine learning in maintaining data quality cannot be overstated. These technologies can automate processes and uncover insights the human eye might miss. That doesn’t mean data quality can be solved overnight with one or two automation hacks or a silver-bullet AI algorithm.
Closing data quality gaps is an ongoing journey, not a destination. As data volumes grow and complexities increase, our approaches must evolve in kind.