4 Trending & Industry-Specific Confidential Computing Use Cases
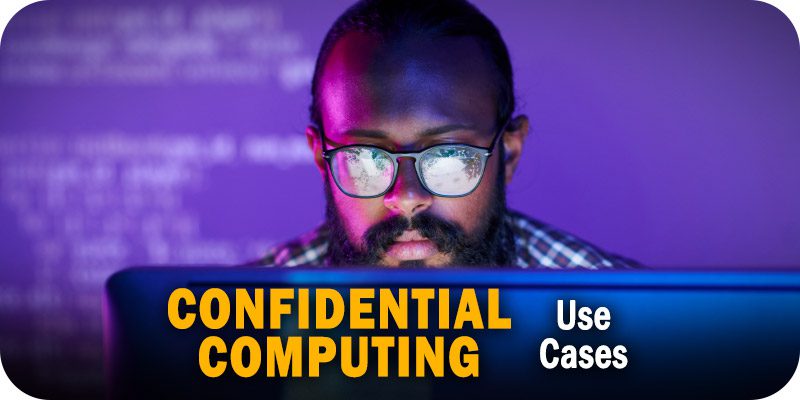
Solutions Review’s Expert Insights Series is a collection of contributed articles written by industry experts in enterprise software categories. In this feature, Opaque Systems Co-Founder and CEO Rishabh Poddar offers his thoughts on some forward-thinking and industry-specific confidential computing use cases to know.
More data means a higher potential for breakthrough advancements in every industry. Effective data collaboration can unlock key patterns across institutions, unearthing never-before-seen insights. Yet, ensuring massive data sets are secure and meet privacy regulations is difficult and have been a roadblock to effective execution.
Confidential computing is a new and mighty player in data security. This technology isolates data within a secure, hardware-protected environment, called an enclave, while it is being processed. The enclave keeps the data encrypted even when in use – while in memory and during computation. With a secure enclave environment, multiple parties can combine their encrypted data and collaborate on analytics and AI use cases, without compromising the confidentiality of their individual data and exposing it to other parties.
This approach is a game-changer in sensitive data collaboration. Not only can data be shared across departments, but institutions around the globe, all without compromising privacy. Confidential computing offers major benefits to organizations across all industries with a need for sensitive data collaboration. In order to understand these benefits more specifically, let’s explore how it can be applied in the following core industries.
Forward-Thinking Confidential Computing Use Cases
Informing Treatment for Rare Cancers
There is an undeniable need for sensitive data collaboration among healthcare institutions. The amount of data produced in this industry is also astronomical – healthcare data providers hold large-scale health care and consumer data from many sources, and provide that data to their customers such as clinical laboratories, EMR suppliers, pharma manufacturers, health IT companies, insurers, health service providers, etc. What’s more, customers want to combine their own data on the platform with the data providers’ data for patient insights. The challenge lies in combining all of this data together to analyze and collaborate on. Many companies and customers are unable and or unwilling to combine their own datasets with information available from data providers for fear of patient re-identification. As a result, the industry is missing out on insights driven by the large mass of healthcare and consumer data in combination with internal data.
With confidential computing, organizations can run machine learning (ML) and complex analytics directly on encrypted data at scale, unlocking model training and rich analytics on the joined dataset while complying with all regulations. Combining HIPAA protected healthcare data with highly specific demographic and psychographic information available from data platforms in a secure and private fashion enables creation of patient profiling models, disease prediction, audience targeting and messaging, and improved risk models.
Identifying Fraud and Combating Money Laundering
It is estimated that up to $2 trillion is laundered globally each year, roughly $300 billion of that takes place in the U.S. alone. These numbers continue to increase, and with it the need for data collaboration across financial institutions. However, data surrounding fraud is especially sensitive and carries detrimental
consequences if susceptible to breaches. The challenge lies in the fact that lines of business (LOBs) often maintain different repositories of their own data and there is often a need for data to be available in a centralized data repository which can enable better computational insights. However, given that these tables often contain confidential personally identifiable information PII data, this slows down data access and increases the entitlement cycle to 2 months or more due to governance around PII data.
Confidential computing enables secure collaboration on data containing PII across LOBs, keeping all the data encrypted in use. Organizations also benefit from faster access to data, obviating the need for human intervention to identify and preprocess PII data, streamlining governance. With this faster access to data, and higher quality of insights available as a result of analysis of encrypted data fields, organizations gain better insights into customer behavior leading to more effective business decisions.
Improving Supply Chain Tracking
Every year, manufacturers lose billions of dollars due to the lack of visibility into crucial shipment data. The ability to share confidential data allows manufacturers to visualize the structure and dynamics of the entire supply chain, letting its players make joint decisions to improve processes or respond to crisis situations. Collaborative data analytics also allow manufacturers to ensure production conditions or raw materials meet quality, safety and ethical customer requirements.
With confidential computing this collaboration is protected as data is tracked throughout all stages of its lifecycle, while providing technical assurances. This ensures that this data can’t be misused, a reality that could result in system downtime, monetary loss, and reputational damage. With 36% of companies reported that cyberattacks significantly disrupted their supply chain, according to The Economist, ensuring data is secure and remains encrypted is especially crucial.
Uncovering Environmental Changes
Data collaboration is a vital and promising solution to combating the primary contributors to climate change. Access to more data helps to advance the efficiency and accuracy of many climate- focused technologies. For example, real-time ocean data monitoring systems allow experts to predict tsunamis, so more access to this data can more quickly uncover patterns to help predict these events sooner. Other use cases include smart diagnostics within water treatment facilities to detect leaks and allow for water savings, and predictive models of wind turbines to determine how much wind power is produced at a particular site. All of which can be improved with a wider network of data from similar machinery.
However, even this sort of data is sensitive in nature. Private organizations want to collaborate on particular data sets for stronger results, but security concerns also arise, as they want to ensure detachment from any company-exclusive data. Confidential computing enables climate data to be collected in a host cloud enclave that can support deep analytics without sharing any of the underlying sensitive data across organizations. This ensures secure collaboration to ease the mind of companies, while also adhering to general data privacy regulations.
Confidential computing ensures sensitive data is secure– preserving the effectiveness and integrity of data collaboration. As utilization of the cloud continues to be the standard for data flow, confidential computing allows organizations to securely collaborate on their sensitive data. Data collaboration is a revolutionary tool to help drive nearly industry forward, as it enables exponential growth in key insights and advancements.