Five Data Masking Best Practices for Securing Sensitive Data
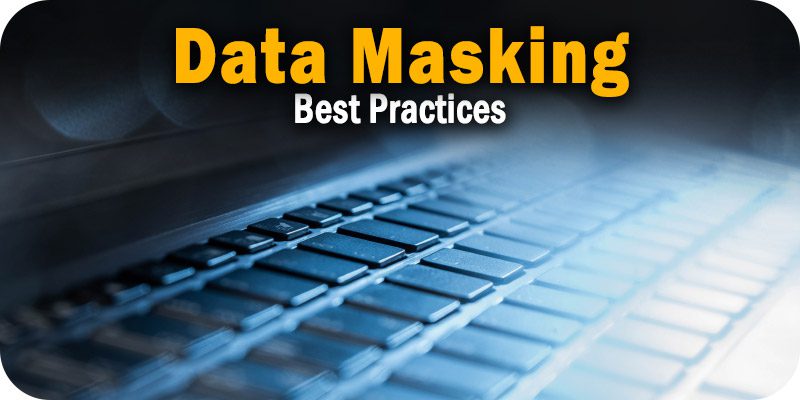
This is part of Solutions Review’s Premium Content Series, a collection of contributed columns written by industry experts in maturing software categories. In this submission, Immuta CTO Steve Touw offers key important data masking best practices for securing sensitive data.
In today’s digitized world, sensitive data is more abundant and available than ever before. Once collected, everything from credit card information to healthcare data and browsing history quickly becomes an organization’s responsibility to protect. Unfortunately, with this increase in the use and sharing of sensitive data comes a rise in data breaches and leaks. In fact, research shows that 83 percent of the 1,862 data breaches in 2021 involved sensitive data.
As a result, data security is top of mind for many organizations. But while protecting sensitive data is critical, it is possible to take it too far. We frequently see IT teams going to the extremes: either leaving their data overly exposed and accessible to risk (high data utility, low security), or completely locking it down, preventing the ability to effectively leverage that information (high security, low data utility). Therefore, finding that sweet spot of safety and accessibility is essential, and can be accomplished by adopting techniques like data masking that allow tradeoffs between the security and utility of sensitive data within storage and compute environments.
Let’s dive further into some of the benefits of data masking and some best practices for allowing sensitive data to be stored and accessed, while maintaining some level of anonymity and safety.
Data Masking Best Practices
It is clear that there is no one-size-fits-all approach to data masking. Understanding what is being protected and why is critical when balancing access and security. With this in mind, here are four best practices that can help organizations roll out an effective data masking strategy:
Identify Your Sensitive Data
This is an important first step in data masking. In order to effectively implement masking techniques, data and governance teams must identify the kind of sensitive data being masked so they can apply policies that ensure the appropriate protection and compliance.
Understand Data Use Cases and Their Risks
After identifying the data, organizations need to understand how it’s going to be used. From there, they can determine different masking techniques and weigh them against potential risks. This is oftentimes referred to as the “security/privacy vs. utility” tradeoff.
Consider Governance Rules & Regulations
Compliance regulations are complex and may apply differently based on the type of data. Take the time to learn and understand any applicable governance requirements, as they may suggest or dictate masking approaches for governed data categories, or in some cases even reduce the compliance burden to allow for broader data sharing and access.
Ensure Repeatability and Scalability
As potentially the most important step in creating a successful and lasting masking strategy, it is vital to implement solutions only if they are scalable long term. As data volumes, analytical users, and privacy regulations continue to grow and evolve, an organization’s data masking policies must be able to keep up. Therefore, the basic foundation of any data masking approach should prioritize repeatability and scalability.
Implementing Secure and Enduring Data Masking
It is clear that sensitive data volumes will continue to expand while the privacy landscape becomes more stringent and security threats grow. There is no silver bullet to navigating these evolving factors, so data teams cannot afford to ignore the need to adopt techniques like data masking, which enables them to conduct effective analytics while ensuring data security. This begins with identifying a scalable masking solution that is best suited for an organization’s current and future business needs and goals.
Benefits of Data Masking
Data masking – also known as data obfuscation – is a form of data access control that takes sensitive information in a data set and makes it unidentifiable, but still available for analytics. This enables organizations to effectively store, access, and derive value from the data while preserving its safety and anonymity. This is key in today’s business environment, where all businesses regardless of size, location, or industry are potential targets for attackers, internal bad actors, and/or privacy regulators.
In addition to the business and safety benefits, data masking also helps from a customer standpoint. Due to growing privacy and governance requirements, today’s customers expect that organizations are taking the necessary steps to secure their sensitive data and use it responsibly. If this trust is broken by misuse or a data breach, it may irreparably damage consumer confidence and brand reputation. Masking techniques reduce the risk of breaches and internal bad actors using data irresponsibly while meeting privacy regulations sweeping the globe. This ultimately protects consumers’ privacy and helps maintain a higher level of trust between an organization and its customers.
Static Data Masking (SDM) vs. Dynamic Data Masking (DDM)
When it comes to implementing a data masking strategy, there are two primary types – Static Data Masking (SDM) and Dynamic Data Masking (DDM). Both have strengths and weaknesses, and thus one may be better suited for a specific data environment than the other. Companies should evaluate which approach best meets their needs by understanding the main differences between them:
Static Data Masking (SDM)
At a high level, static data masking masks data at rest rather than in active use. This is accomplished by creating a copy of an existing data set and hiding or eliminating all sensitive and/or personally identifiable information (PII). This copied data is then free to be stored, shared, and used, free of any sensitive information, and is completely detached from the initial set.
This type of masking is a better fit for environments where data does not change over time and is only used for a single purpose, such as software and application development or training. A large downside to SDM, however, is that it is unable to easily scale when larger data sets and/or combinations of access levels are introduced. Additionally, since the data is static, it is not well suited for analytical use cases. For these reasons, organizations should stay away from SDM for analytical purposes.
Dynamic Data Masking (DDM)
Unlike SDM, DDM applies masking techniques at query-time, and does not involve moving, copying, or separating the data from its original source. This helps teams avoid any confusion and silos around data copies that have been scrubbed and masked for different reasons. It also remains updated and “live,” which is critical for analytics.
Since DDM is not tied to where the data is copied or stored, it is often considered to be the most widely-applicable type of masking. It also easily scales to more complex policy scenarios and use cases, making compliance much easier to manage.