Data Warehouse vs. Data Lake; What’s the Difference?
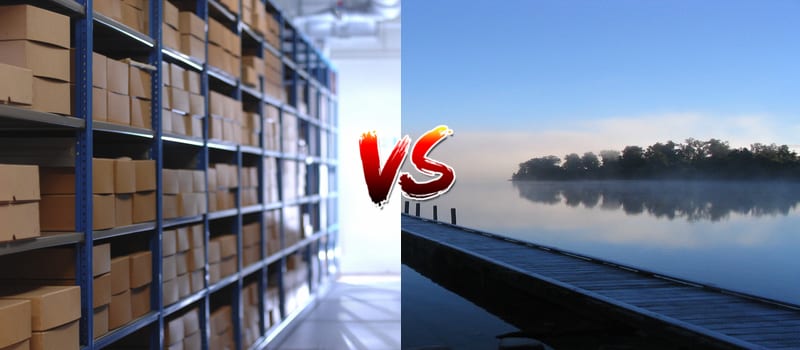
The data warehouse model has always been the foundation for organizations looking to uncover insights from their data. However, there’s a new technology on the block, and it’s making many organizations uncomfortable, forcing them to ask the question as to whether or not their Data Management techniques are outdated. That technology is the Data Lake, which to many in tech is the natural progression of the data warehouse given the sheer volumes of data that enterprises now need to run analytics against. As a result of the Data Lake’s emergence, data-driven enterprises all over are wondering if they need to update their approach to Information Management.
The fact of the matter is, the Data Lake isn’t going to drive data warehousing to extinction any time soon. Enterprises should develop a unified view of their data environments to decide which direction they should take their Information Management framework in. Although it is becoming more of a rarity, there are still some enterprises that collect only transactional data or that already have an expansive data warehouse environment in place, which would make adopting a new Data Management technology costly and labor intensive. The Data Lake can absolutely solve modern problems that warehousing cannot, but some organizations simply don’t need to make the switch.
Data warehouses typically play host to relational database technologies, referred to many forward-thinking vendors as “legacy” tools. This approach to Data Management is highly governed, as warehouses store data in a structured manner, segmenting data into stores based on specific data types. Data warehouses are made up of data that has already been integrated, but they are limited in that they have trouble hosting data from unstructured sources, such as data collected from product sensors, social media and other non-traditional sources.
In using the data warehouse model, data isn’t loaded until users have a defined use for it. This of course is a positive for data architects in that the data is easy to understand and formatted in a way that helps them easily answer the questions they need answers to, allowing for queries on structured data. Data warehouse technology transforms data as it is being injected into the database, a process known as schema on-write.
Enterprises usually have to have multiple data warehouses set up to run analysis on different types of data because once a database is in place it takes a considerable amount of time and energy to change them. For that reason, enterprises that want to analyze a wide variety of data types are coming to the realization that the data warehouse model is slowing them down. Given the rise in self-service Business Intelligence and ad-hoc analytics, many organizations are now considering the Data Lake.
The Data Lake is similar to traditional data warehousing in that they are both repositories for data, but that’s really where the comparison ends. Unlike the data warehouse, Data Lakes are schema on-read, meaning that data is only transformed once it is ready for use. That is, once the user selects a certain piece of information as something they want to use inside an analytics tool. Data Lakes have no regulatory functions, so any amount of data from any data source can be dumped into them. This allows enterprises that want to collect data from any source to simply connect those sources to their Data Lake.
In this way, the Data Lake enables the storage of data in its raw form, with virtually no limit as to how much data can sit in the waters at any one time. The data can be historical or streaming in real-time. This allows organizations to use data as it is funneled into the Data Lake or very far in the future. This technology gives companies the ability to store and use Big Data, allowing them to embrace non-traditional data types. Hadoop, an open source Big Data framework that has grown exponentially over the last decade, aligns well with the Data Lake based on the pure amount of data that these environments allow enterprises to stash away.
Data Lakes enable enterprises to look past the type and structure of data, giving them the chance to collect as much data as they desire. Since data is collected straight from the source systems, companies don’t have to put resources aside to regulate it. Of course, this can sometimes create a logjam when users want to run a query since sifting through large amounts of data can be time consuming. The Data Lake enables digital businesses to go beyond the capabilities of data warehouses, but it does not come without added responsibility.
Though Data Lakes allow users to go beyond the structure of the data warehouse to explore data in unconventional ways, security concerns remain. Since the technology is largely open source and so vaguely structured, it is possible for sensitive data to be compromised. In addition, many companies lack users with the necessary skills to take control of this emerging Data Management technology. And finally, an organization’s hardware environment has to be vastly different than a legacy environment to deploy a Data Lake with any success. This means that in the end, the debate as to which framework is more suitable for a specific environment depends entirely on the enterprise in question.
Widget not in any sidebars