Data Management Predictions from Experts for 2025
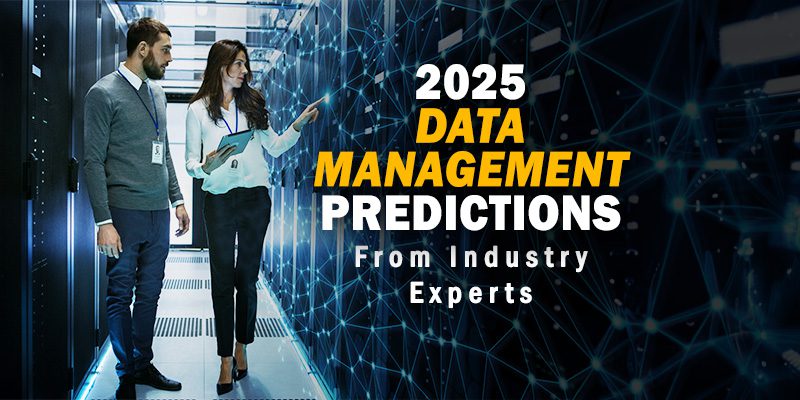
For our 6th annual Insight Jam LIVE!: Strategies for AI Impact. Solutions Review editors sourced this resource guide of data management predictions for 2025 from Insight Jam, its community of enterprise tech and AI builders, implementors, and experts. Join Insight Jam free for exclusive expert insights and much more.
As enterprises gear up for 2025, the importance of effective data management has never been more critical. With the exponential growth of data, the increasing complexity of hybrid and multi-cloud environments, and the expanding role of artificial intelligence, organizations face a pivotal moment in managing their most valuable asset.
This curation brings together expert predictions from our enterprise tech and AI community—builders, implementors, and strategists who are shaping the future of data management. Their insights illuminate the trends, tools, and best practices that will define the next generation of data-driven success.
From advances in automation and governance to innovative approaches for ensuring data quality and accessibility, these predictions provide a roadmap for navigating the challenges and opportunities of the year ahead. Dive into this collection to uncover the strategies and perspectives of those leading the charge in transforming how enterprises manage their data in 2025.
Data Management Predictions from Experts for 2025
Ashwin Rajeeva, Acceldata
Data Governance, Bolstered by Advanced Data Observability, Will Evolve Into a Strategic Asset
Data governance, bolstered by advanced data observability, will evolve into a strategic asset, driving both compliance and innovation in the AI-driven business landscape of 2025. Organizations will enhance governance frameworks with observability tools focused on data reliability, compliance, and ethical use. This evolution will provide visibility into data lineage and metadata, enabling organizations to meet regulatory standards with operational agility.
To Ensure Ethical and Transparent AI Practices in 2025, Organizations Will Utilize Data Observability
To ensure ethical and transparent AI practices in 2025, organizations will utilize data observability to monitor and validate the data underpinning AI systems, thereby mitigating bias and fostering trust with stakeholders. Data observability will play a key role in supporting responsible AI development, aligning with corporate values such as sustainability and ethical governance to build long-term trust.
Unified Data Observability Platforms Will Emerge as Essential Tool for Large Enterprises
In 2025, unified data observability platforms will emerge as essential tools for large enterprises, enabling comprehensive visibility into data quality, pipeline health, infrastructure performance, cost management, and user behavior to address complex governance and integration challenges. By automating anomaly detection and enabling real-time insights, these platforms will support data reliability and streamline compliance efforts across industries.
Rohit Choudhary, Acceldata
High-quality data will drive successful AI across enterprises
In 2025, the success of AI and ML operations will hinge on the foundation of high-quality data, robust enterprise infrastructure, and well-trained models. Reliable, accurate data will be essential for scaling AI-driven decision-making, allowing organizations to deploy AI as a core strategic asset. Enterprises equipped with consistent, high-quality data will respond swiftly to market changes, leveraging AI to fuel continuous innovation and maintain a competitive edge.
Operational insight from data will drive business decisions and enable strategic workflows
By 2025, data-driven insights will extend beyond technical teams, empowering C-suite executives and non-technical leaders with the information needed for informed decision-making. Organizations will leverage accessible, actionable dashboards that provide operational visibility across data workflows, transforming data insights into a critical resource for guiding strategic priorities and fostering business success.
Hybrid multi-cloud infrastructure will be standard, supported by data observability
In 2025, hybrid multi-cloud environments will become the standard for data-driven enterprises, optimizing security, privacy, and cost management. Data observability will be essential for ensuring seamless operations and unified visibility across these diverse infrastructures, helping organizations manage multi-cloud and on-premises data assets with enhanced resilience.
Structured data will become the backbone of AI-driven insights
In 2025, structured and semi-structured data sources will form the foundation for robust AI-driven insights. By leveraging data lakes, data warehouses, and data streams, organizations will enhance AI model training, improve data quality, and generate more accurate, actionable intelligence. This strategic approach will empower businesses to unlock the full potential of AI, driving continuous innovation and maintaining a competitive edge.
Jeremy Kelway, EDB
Data governance and quality will be the biggest barriers to successful and ethical AI adoption
In 2025, data governance, accuracy, and privacy will emerge as the most significant barriers to effective AI adoption. As organizations look to scale AI, the realization will occur that successful AI outcomes are entirely dependent on trustworthy data. Managing and preparing massive amounts of data, ensuring compliance, and maintaining accuracy will provide complex challenges. Enterprises will need to overcome these hurdles by investing in foundational data platforms that include robust governance controls. As a result, we’ll see a stronger emphasis on data stewardship roles and governance frameworks that align with AI initiatives, as businesses recognize that unreliable data directly impacts AI effectiveness.
Seamless integration of AI and data will redefine core business functions
Moving into 2025, AI capabilities will no longer be siloed as a separate technology, we will start to see these technologies woven seamlessly into core business applications, enhancing traditional business functions and customer experiences. In the next phase of AI, we anticipate a graduation from Proof of Concept and narrowly scoped AI initiatives. Instead, organizations are aiming to incorporate AI into the main architecture of their business platforms, treating data and AI as unified capabilities. This transformation will enable businesses to increase productivity and decision-making power by incorporating AI as a foundational component rather than an add-on.
Jozef de Vries, EDB
Data and AI sovereignty will drive strategic differentiation for enterprises
In 2025, enterprises that prioritize data and AI sovereignty will gain a strategic edge by building secure, compliant AI platforms aligned with regulatory expectations. With increasing focus on data protection laws, companies will treat data sovereignty as a core principle. Open-source solutions like PostgreSQL will see rising adoption, supporting flexible, compliant AI environments that enable innovation within regulatory boundaries.
Data quality will become a foundational priority for AI-driven enterprises
As businesses adopt AI at scale, they will recognize that their outcomes are only as strong as the quality of their data. This understanding will drive increased investment in established data management technologies—like PostgreSQL—known for reliability, stability, and robust community support. Trusted vendors and open-source technologies will be favored to build resilient AI platforms, as these are seen as essential in managing data effectively while mitigating the complexities of data governance and privacy.
Joe Regensburger, Immuta
Model security—specifically data security, data lifecycle management, and data telemetry—will be a top priority as Commercial-off-the-shelf (COTS) foundational models drive quicker adoption of Generative AI functionality across multiple industries
Enterprises can now build applications around COTS AI models, reducing the need to acquire and maintain specialized hardware, and affording Generative AI companies the opportunity to amortize astronomical training costs across multiple users. This has been a revolution in machine learning, but it carries a cost to security. The fact that there are a relatively small number of models serving a broad number of users, makes these foundational models tempting targets for adversaries both in terms of training and avoidance. We are applying generative AI to more tasks, and empowering generative AI with a degree of autonomy. This increases the responsibility for AI developers to demonstrate that the data they use to train and refine model predictions is clean, timely, and has provable lineage. We will see a greater need for tools which automate the track data usage throughout its lifecycle.
Mark Cusack, Yellowbrick Data
Hybrid Cloud Will Become the Standard
Hybrid cloud deployments will become the norm, driven by the philosophy of “own the base, rent the spike.” This approach offers the best balance of cost and flexibility, enabled by Kubernetes-powered solutions that make portability seamless across on-premises and multi-cloud environments.
- Own the base, rent the spike: Businesses will keep core workloads on-premises and scale up with the cloud during peak demand.
- Cost and flexibility: A hybrid cloud will provide optimal cost efficiency while maintaining flexibility.
- Kubernetes-enabled agility: Organizations will find that Kubernetes will make it easy to move workloads between cloud and on-premises while supporting agile operations.
This shift will give companies the freedom to scale as needed without sacrificing control or cost-efficiency.
Data Sovereignty will Force Companies to Rethink Cloud Strategies
As data privacy laws tighten, companies will face increasing legal pressure to keep data within national borders. This shift will be driven by:
- Weaponization of tax laws: Governments will use tax policies to enforce data sovereignty.
- Risk aversion: Companies will avoid even tokenized data crossing borders to mitigate legal and security risks.
- Rise of colocation: In regions without cloud service provider (CSP) presence, businesses will move to run cloud-native solutions in local data centers.
The proliferation of data sovereignty regulations will force companies to rethink their cloud strategies, prioritizing in-country data storage and processing solutions to stay compliant and secure.
Molly Presley, Hammerspace
GPU Centric Data Orchestration Becomes Top Priority
As we head into 2025, one of the challenges in AI and machine learning (ML) architectures continues to be the efficient movement of data to and between GPUs, particularly remote GPUs. GPU access is becoming a critical architectural concern as companies scale their AI/ML workloads across distributed systems. Traditional data orchestration solutions, while valuable, are increasingly inadequate for the demands of GPU-accelerated computing.
The bottleneck isn’t just about managing data flow—it’s specifically about optimizing the transport of data to GPUs, often to remote locations, to support high-performance computing (HPC) and advanced AI models. As a result, the industry will see a surge in innovation around GPU-centric data orchestration solutions. These new systems will focus on minimizing latency, maximizing bandwidth, and ensuring that data can seamlessly move across local and remote GPUs.
Companies already recognize this as a key issue and are pushing for a rethinking of how they handle data pipelines in GPU-heavy architectures. Expect to see increasing investment in technologies that streamline data movement, prioritize hardware efficiency, and enable scalable AI models that can thrive in distributed and GPU-driven environments.
Breaking Down Data Silos Will Become a Central Focus for AI and Data Architects
In 2025, breaking down data silos will emerge as a critical architectural concern for data engineers and AI architects. The ability to aggregate and unify disparate data sets across organizations will be essential for driving advanced analytics, AI, and machine learning initiatives. As the volume and diversity of data sources continue to grow, overcoming these silos will be crucial for enabling the holistic insights and decision-making that modern AI systems demand.
The focus will shift from the infrastructure toward the seamless integration of data across various platforms, teams, and geographies. The goal will be to create an ecosystem where data is easily accessible, shareable, and actionable across all domains. Expect to see new tools and frameworks aimed at simplifying data integration and fostering greater collaboration across traditionally siloed environments.
Enterprise HPC Must Align with Standardized Technologies for Unstructured Data Processing
By 2025, medium to large enterprises will face a pivotal challenge: integrating high-performance computing (HPC) for unstructured data processing while adhering to enterprise standards. As organizations increasingly rely on AI and data analytics to gain a competitive edge, the need to process vast amounts of unstructured data—like text, images, and video—will be unavoidable. However, enterprises have long struggled to adopt HPC at scale due to the complexities of reconciling specialized HPC technologies with enterprise requirements for security, compliance, and operational standards.
The solution lies in the development of HPC technologies designed to work within enterprise-standard environments. In 2025, we expect to see the rise of enterprise-ready HPC solutions that seamlessly integrate with standard clients, operating systems, networks, and security frameworks. This convergence will enable organizations to finally leverage HPC for large-scale unstructured data processing without compromising on enterprise security, compliance, or performance standards.
Haseeb Budhani, Rafay Systems
GenAI Will Transform Data Graveyards Into AI Goldmines
- Organizations are sitting on “data graveyards” — repositories of historical information that became too resource-intensive to maintain or analyze.
- This is largely because it can be expensive to tag data and keep track of it. Many companies defaulted to “store everything, analyze little” approaches due to the complexity and high costs related to data management.
- Yet valuable insights remain buried in emails, documents, customer interactions and operational data from years past.
- With GenAI tooling, there’s an opportunity to efficiently process and analyze unstructured data at unprecedented scale.
- Organizations can uncover historical trends, customer behaviors and business patterns that were too complex to analyze before.
- Previously unusable unstructured data will become a valuable asset for training domain-specific AI models.
Mohan Varthakavi, Couchbase
Businesses will adopt hybrid AI models, combining LLMs and smaller, domain-specific models, to safeguard data while maximizing results
- Enterprises will embrace a hybrid approach to AI deployment that combines large language models with smaller, more specialized, domain-specific models to meet customers’ demands for AI solutions that are private, secure and specific to them.
- While large language models provide powerful general capabilities, they are not equipped to answer every question that pertains to a company’s specific business domain. The proliferation of specialized models, trained on domain-specific data, will help ensure that companies can maintain data privacy and security while accessing the broad knowledge and capabilities of LLMs.
- Uses of these LLMs will force a shift in technical complexity from data architectures to language model architectures. Enterprises will need to simplify their data architectures and finish their application modernization projects.
Data architectures will be redesigned to support AI integration and ensure transparency
- As AI becomes more integrated into applications, data architectures will be fundamentally redesigned to support AI workloads. Companies will implement new data architectures that go beyond simple record storage to capture the “intelligence history” and thought processes of AI systems. They will need to simplify complex architectures, including consolidation of platforms, and eliminate data silos to create trustworthy data.
- These evolved architectures will incorporate robust security measures for both data and AI communications. They will prioritize transparency and governance, enabling organizations to track how their data was used in AI training, monitor the decision-making processes of AI systems, and maintain detailed records of AI-generated insights and their underlying reasoning.
Moshie Weis, Check Point Software
Cloud-Native Solutions to Shape the Future of Data Security
With data spread across diverse cloud-native architectures, adaptive, data-centric security is essential. Cloud-native solutions now provide dynamic protection across data lifecycles, securing data at rest, in motion, and in use. This will be critical in 2025 as stricter compliance standards and more data-centric attacks demand robust, consistent security for data everywhere. In 2025, cloud-native solutions will be crucial for staying resilient, adapting to new regulations, and navigating an ever-evolving threat landscape.
Tom Keuten, Rightpoint
Data Governance will Become the Backbone of AI-Powered EX
As AI takes center stage in improving employee experience, the spotlight will increasingly fall on the integrity of data. Trust will be the key differentiator in successful AI implementations, and technologies related to data governance, quality, and explainability will be critical. With AI automating decisions and providing insights, employees and companies must trust the outputs. Building this trust will require robust data foundations that ensure accuracy, privacy, and transparency, making data governance essential for the future of AI-driven employee experience.
Rajan Goyal, DataPelago
Enterprise Open Source Investments Grow
Contrary to the notion that open-source is dying in the enterprise, 2025 will mark a resurgence in its adoption, driven by the rising demand for cost-efficient solutions amidst growing IT and AI budgets. Gartner predicts that software spending will grow by 14 percent in 2025 to $1.24 trillion, driven by several factories including AI investments. Overall, Gartner projects global IT investments will near $6 trillion in 2025, up 34 percent from 2024.
At DataPelago, we see our enterprise customers, particularly larger organizations, increasingly investing in open source to lower total cost of ownership (TCO). For these companies, open source offers flexibility and ROI potential, but it also comes with the burden of complex management – a challenge they are equipped to handle as they typically have dedicated open source teams. This trend highlights a circular dynamic: by improving performance, enterprises can optimize costs, reinforcing the appeal of open source in mission-critical, cost-conscious environments. As open source tools continue to mature, particularly for managing the complexities of AI workloads, open source will remain central to enterprise innovation.
Data Quality Supersedes Quantity, Placing a Greater Onus on AI Customers
We’re seeing growing reports that LLM providers are struggling with model slowdown, and AI’s scaling law is increasingly being questioned. As this trend continues, it will become accepted knowledge next year that the key to developing, training and fine-tuning more effective AI models is no longer more data but better data. In particular, high-quality contextual data that aligns with a model’s intended use case will be key. Beyond just the model developers, this trend will place a greater onus on the end customers who possess most of this data to modernize their data management architectures for today’s AI requirements so they can effectively fine-tune models and fuel RAG workloads.
Francois Ajenstat, Amplitude
2025 will mark the shift from “big data” to “small data” as organizations focus on quality over quantity
The past few years have seen a rise in data volumes, but 2025 will bring the focus from “big data” to “small data.” We’re already seeing this mindset shift with large language models giving way to small language models. Organizations are realizing they don’t need to bring all their data to solve a problem or complete an initiative – they need to bring the right data. The overwhelming abundance of data, often referred to as the “data swamp,” has made it harder to extract meaningful insights. By focusing on more targeted, higher-quality data– or the “data pond”– organizations can ensure data trust and precision. This shift towards smaller, more relevant data will help speed up analysis timelines, get more people using data, and drive greater ROI from data investments.
Bipin Singh, Redpanda
Streaming Data Platforms will Become Indispensable
In 2025, streaming data platforms will become indispensable for managing the exponential growth of observability and security data. Organizations will increasingly adopt streaming data platforms to process vast volumes of logs, metrics, and events in real-time, enabling faster threat detection, anomaly resolution, and system optimization to meet the demands of ever-evolving infrastructure and cyber threats.
Streaming Data and Agentic AI
In 2025, streaming data platforms will serve as the backbone for agentic AI, RAG AI and sovereign AI applications, providing the low-latency, high-throughput capabilities required to power autonomous decision-making systems and ensuring compliance with data sovereignty requirements.