Driving Business Agility with Self Service Data Management
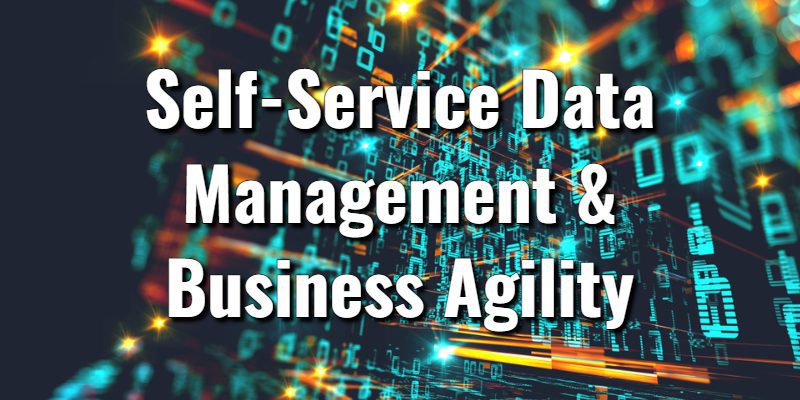
Solutions Review’s Contributed Content Series is a collection of contributed articles written by thought leaders in enterprise technology. In this feature, Modak Head of Product Management Mayank Mehra offers commentary on how to drive business agility with self-service data management.
Organizations continue to face a pressing challenge of harnessing the power of data swiftly and effectively, as traditional data management approaches no longer suffice in the face of increasing volumes and complexity. Compounding the problem is that most data consumers rely solely on IT teams for data-related tasks which can lead to bottlenecks, delays, and limited agility. While there are many technology options that can help minimize the impact, it’s clear that enterprises need a solution that empowers business users to access, analyze, and utilize data themselves. While self-service data management is not a new approach, it remains a game-changing alternative that empowers decision-makers at all levels with direct access to data and enables them to make informed choices in real-time.
Understanding Self-Service Data Management
Self-service data management is driven by several factors that have transformed the way organizations handle and leverage their data. These drivers have emerged because of the rising volume, diversity, and speed at which data is generated, along with the growing demand for agility and effectiveness in making data-driven decisions.
One of the key drivers is data democratization. With self-service data management, organizations empower business users to access and analyze data without heavy reliance on IT departments. This transition helps eliminate obstacles, facilitates quicker decision-making, and fosters a data-driven culture across the organization.
Another crucial element of self-service data management is the growing complexity of today’s data ecosystems. As data sources multiply and data formats diversify, self-service tools provide necessary capabilities for tasks like data preparation. They streamline the integration, transformation, and analysis of data from various sources in a unified and user-friendly manner. This reduces the dependence on specialized technical skills and simplifies data operations.
The demand for agility and responsiveness also drives the adoption of self-service data management. Traditional approaches often involve lengthy requests and development cycles, hindering timely insights. Self-service tools on the other hand empower users to explore and analyze data at their own pace, enabling quick experimentation, discovery, and iterative decision-making.
Data Management
How Self-Service Data Management Addresses Existing Business Challenges:
Data Integration and Data Silos
Self-service data management tools are instrumental in enhancing data integration and dismantling data silos within organizations. It is challenging to integrate and thoroughly evaluate data because of these silos, leading to fragmented insights and inefficient decision-making. Fragmented data silos across organizations hinder data integration and sharing across systems, making it difficult to gain a comprehensive view of the data.
Also, self-service data management tools provide a no-code/low-code, UI-driven approach for all data integration tasks. Users can transform and prepare data according to their requirements, ensuring its consistency and usability. Data governance features enforce security and compliance policies, safeguarding sensitive information during integration and sharing processes.
By empowering users to create and manage their own data pipelines, self-service tools reduce dependency on IT teams and enable faster integration cycles. Collaboration features promote cross-functional teamwork and knowledge sharing, breaking down data silos across departments. Additionally, real-time data integration capabilities ensure that organizations can leverage the most up-to-date information for timely decision-making.
Augmented Data Cataloging
Self-service data management tools greatly enhance the process of data cataloging within organizations. These tools used machine learning to automate the discovery of data assets by analyzing metadata and content, expediting the cataloging of large volumes of data. Users can enrich metadata with contextual information, such as descriptions and tags, improving the understanding and searchability of data assets.
Furthermore, self-service tools capture data lineage, providing insights into data dependencies and impact analysis. They also assess data quality, identifying and prioritizing assets for improvement. Integration with data governance frameworks ensures that cataloged data aligns with established policies, standards, and compliance requirements.
Ultimately, self-service data management tools streamline data cataloging by automating discovery, facilitating user-driven annotation, capturing lineage, assessing data quality, and integrating with data governance. This leads to comprehensive and accurate data catalogs that enhance data understanding and utilization.
Enhanced Data Quality and Observability
Self-service data management tools play a vital role in augmenting data quality and observability within organizations. These tools provide capabilities that enable users to assess, monitor, and improve data quality.
Users can embed data quality and observability checks to automate the identification of data anomalies, schema drift, data change, inconsistencies, and inaccuracies in the data. By highlighting data quality issues and observability alerts, self-service tools empower users to take corrective actions, such as data cleansing and enrichment, to improve the overall quality of the data.
Best Practices for Self-service Data Management
Organizations can follow these best practices to efficiently adopt self-service data management and avoid any barriers to foster successful implementation:
- Data Governance: Establish a strong data governance framework that addresses data security and privacy concerns. This involves implementing robust access controls, encryption mechanisms, and monitoring systems.
- Data Quality Checks: Prioritize data quality by defining data standards, conducting regular data quality assessments, and providing training on data integration best practices. Establish clear guidelines and validation mechanisms to ensure data consistency and accuracy.
- Skill Development: Invest in user training and support programs as this is crucial to enhancing technical expertise and ensuring users are proficient in using self-service data management tools. This can include providing training resources, conducting workshops, and offering ongoing assistance. Additionally, fostering a culture of collaboration and knowledge sharing can encourage adoption and maximize the benefits of self-service data management.
Self-service data management presents a powerful solution for organizations seeking to drive business agility in today’s fast-paced environment. By empowering business users with direct access to data, self-service tools enable informed decision-making. Moreover, self-service data management enhances data integration, dismantles data silos, augments data cataloging, and improves data quality and observability.
By adopting best practices in data governance, quality assessment, skill development, and fostering a collaborative culture, organizations can successfully implement self-service data management and unlock the full potential of their data assets.