Real-Time or Left Behind: The New Reality of AI in Enterprise
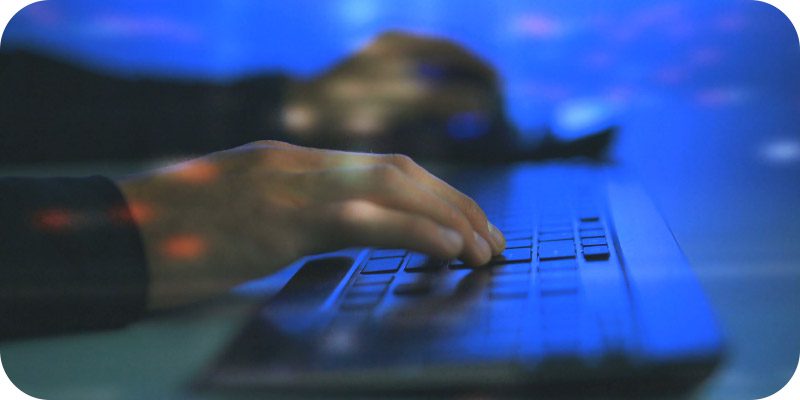
Redpanda Data’s Tristan Stevens offers insights on real-time or left behind and the new reality of AI in the enterprise. This article originally appeared on Solutions Review’s Insight Jam, an enterprise IT community enabling the human conversation on AI.
When a cargo ship struck Baltimore’s Francis Scott Key Bridge in the spring of 2024, the impact rippled far beyond the physical destruction. Within minutes, logistics companies needed to reroute shipments, insurers had to assess risks, and traders had to reevaluate positions in affected companies. For businesses relying on AI systems trained on historical data, this sudden change, in reality, created a dangerous disconnect – their algorithms were still operating in a world where the bridge existed.
This scenario illustrates a critical challenge facing organizations today: building AI systems that don’t just learn from the past, but also understand and adapt to the present. While many companies have successfully experimented with AI in controlled environments, they now face the complex challenge of deploying these systems in production environments where decisions must be made on constantly changing, real-world data.
The Data Currency Challenge
Keeping an AI model current and productive is challenging in the face of a crucial reality: data becomes stale really fast, and the businesses that thrive are going to be the ones with the most up-to-date information. According to Gartner’s “Top Strategic Technology Trends for 2024” report, in 2025, over 75 percent of enterprise-generated data will be created and processed outside traditional centralized data centers or clouds, highlighting the growing importance of edge computing and real-time data processing.
We’ve seen how a major disaster like a bridge collapse can impact real-world scenarios, but these incidents and extreme outliers are becoming more common, showing how data currency is becoming ever more important when dealing with:
- Contextual information about current geopolitical events
- Weather events affecting business operations
- Infrastructure changes impacting supply chains
- Market-moving announcements affecting trading decisions
Training models on yesterday’s data are no longer sufficient. By the time one region wakes up, the business landscape may have already shifted dramatically on the other side of the world.
The Convergence of Traditional and Real-Time Systems
Historically, organizations operated in two distinct worlds: batch processing for historical analysis and real-time streaming for immediate insights. Users had to navigate different tools and technologies depending on their data needs – data warehouses for historical analysis, specialized query languages like Apache Kafka’s KSQL for stream processing, or solutions like ClickHouse for real-time analytics.
Today’s users don’t think in these bifurcated terms. They expect data to be current and accessible through natural language queries, regardless of its source or processing method. This shift has driven a massive convergence of previously separate IT infrastructure components.
- A modern data architecture requires several key components working in harmony:
- Legacy information and batch data that forms the business’s historical foundation
- Contextual and reference data specific to the industry
- Real-time data providing current situational awareness
- AI and language processing capabilities that can integrate all these sources
Data Sovereignty and Security Considerations
As organizations integrate AI into their core processes, data sovereignty is emerging as a strategic choice that balances the opportunities to utilize AI while minimizing the risks of external AI vendors. While large language models from providers like OpenAI offer powerful capabilities, they also raise serious questions about data security and intellectual property protection.
“You only need to leak once,” as the saying goes. We know that whatever gets on to the internet is probably out there forever. Recent incidents highlight these risks. Beyond the widely reported cases of engineers accidentally exposing code through ChatGPT, organizations have faced challenges with:
- Employees uploading sensitive financial data to public AI tools
- Competitors gaining insights through public AI model training data
- Regulatory violations from cross-border data transfers through AI services
The concept of sovereign AI is one solution to this security challenge. Rather than sending sensitive data across the internet to third-party services, organizations can bring AI capabilities within their security boundaries. This approach allows companies to:
- Run AI models within their own infrastructure
- Combine proprietary data with general language models
- Maintain complete control over data lineage and access
- Ensure compliance with regulatory requirements
Best Practices and Implementation Strategies
Prompt Engineering and Quality Control
Asking the right questions of AI systems is crucial for success. Organizations should develop systematic approaches to creating prompts that include sufficient context, direction, and examples. Teams should maintain documentation of successful prompt patterns and implement iterative feedback processes to improve results.
Democratized Access and Integration
Modern AI implementations must balance accessibility with control. Business users should be able to embed AI into their processes with minimal technical overhead while maintaining appropriate safety guardrails. Systems should integrate seamlessly with existing workflows rather than requiring users to switch between multiple applications.
Real-Time Data Quality Management
As data volumes and velocities increase, organizations must implement automated validation checks and monitor data freshness in real-time. Clear data ownership and governance structures ensure accountability throughout the system.
Security and Compliance Integration
Security cannot be an afterthought in real-time AI systems. Organizations should implement comprehensive monitoring of AI interactions and establish clear policies for data usage and retention. Regular security assessments protect sensitive information and maintain compliance.
Continuous Monitoring and Optimization
Systems must adapt as business needs evolve. Organizations should track both technical performance metrics and business impact, using these insights to guide optimization efforts and ensure systems meet business objectives effectively.
Looking Ahead
The Baltimore bridge collapse highlighted a critical truth about modern AI systems: processing historical data alone isn’t enough. Organizations that thrived during the incident were those whose systems could rapidly incorporate and act on real-time information, from rerouting shipments to reassessing market positions. As AI becomes more deeply embedded in critical business processes, this ability to adapt to sudden changes while maintaining security and reliability at scale isn’t just advantageous – it’s essential for business survival and success.