Simplifying Data Access with Generative AI: Paving the Path to Data Democratization
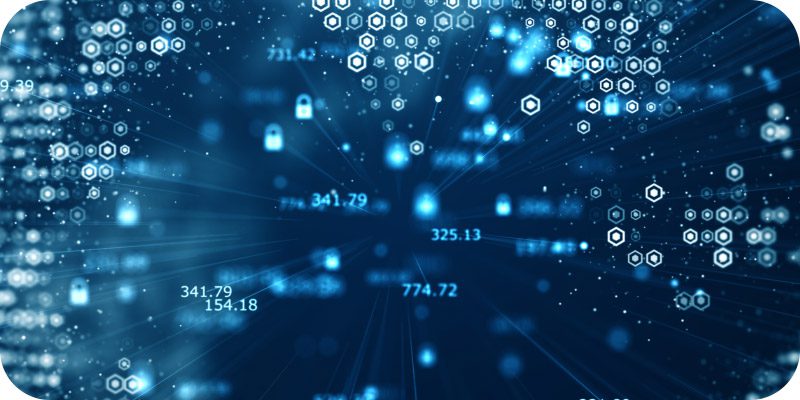
Denodo’s Paul Moxon offers insights on simplifying data access with GenAI and paving the path to data democratization. This article originally appeared on Solutions Review’s Insight Jam, an enterprise IT community enabling the human conversation on AI.
Data-driven information is the lifeblood of innovation. As artificial intelligence (AI) and generative AI (GenAI) continue to reshape how organizations interact with data, one fundamental question arises: Does GenAI have the potential to democratize data?
The short answer: Yes. However, GenAI relies on the quality of the data it’s fed. With trustworthy data, GenAI can democratize data access, empowering business users as well as their technical teams – so that everyone can leverage AI’s potential to drive smarter decisions and innovation.
Data Democratization Complexities
Traditionally, businesses have depended heavily on their IT departments for data retrieval and simplification. This reliance has led to bottlenecks that can have widespread negative impacts, including missed opportunities, inefficiencies in operations and significant constraints on timely, accurate decision-making.
Data self-service capabilities have the potential to empower business users to independently access, query and analyze data without relying on constant IT intervention. Such capabilities could break down data silos and foster simplified data exploration while reducing the burden on IT teams.
Unfortunately, true data self-service requires organizations to seamlessly unify such silos, and depending on the system and the application, this can be complex. Can GenAI streamline this process? It can, but GenAI faces a few of its own challenges regarding data, and these need to be addressed first.
Challenges to Scaling
According to Statista, the GenAI market in North America is projected to grow at an annual rate of 46.46% from 2024 to 2030, reaching more than $130 billion by the end of the decade. Despite the hype around GenAI, many companies struggle to move beyond pilot projects and achieve impactful return on investment (ROI). For GenAI to perform optimally, it requires a secure, trusted, high-quality data foundation, and one of the biggest challenges is dealing with the fragmentation of data sources.
Key concerns include:
- Preventing AI Hallucinations: Inaccurate or incomplete data can lead to GenAI “hallucinations,” or false, misleading information.
- Protecting Sensitive Information: Data democratization should not come at the expense of security, so GenAI needs to be implemented with strong security in place. Organizations must strike a balance between data accessibility and the protection of sensitive information, particularly in industries with stringent data privacy regulations.
- Meeting Regulatory Compliance: As AI regulations evolve, companies need to maintain control over their data management practices, enabling compliance with emerging standards.
Addressing these challenges requires a well-architected data management strategy, supported by technologies like data fabric and advanced data governance frameworks.
Building a Solid Foundation with Logical Data Management
Most organizations manage a diverse ecosystem of applications and systems tailored to various functions, from supply chain management to marketing automation. Businesses may also handle multiple data systems, including data warehouses, data marts, and data lakes.
A common strategy for managing this complexity involves physically consolidating data from multiple systems into a single repository. While this approach is relatively straightforward to implement initially, it becomes increasingly impractical and inflexible as the number of data sources and the volume of data continue to grow.
Conversely, organizations can establish a logical data management platform that serves as a unified control point for managing data across the organization. This approach brings disparate data sources into a cohesive view, forming the basis for a comprehensive data fabric. A data fabric simplifies data management by connecting data sources through metadata, enabling seamless data integration while also providing strong data security and governance.
By leveraging a data fabric, organizations can provide their AI systems with accurate, up-to-date information, regardless of where the data resides. This is crucial for AI use cases in which real-time insights are needed, such as dynamic customer interactions, predictive maintenance, or rapid decision support.
Teachable Moments
Retrieval augmented generation (RAG) is a powerful technique that enhances large language models (LLMs) with contextually relevant data, improving the precision of GenAI outputs and how they are used. While RAG is often used with static information, such as product documents or policy guidelines, its potential extends to real-time data integration.
Without RAG, the AI responds to user questions based only on the information it was trained on—essentially, what it already “knows.” With RAG, the AI can go a step further. RAG includes a process to look up additional information based on the user’s question. The AI then combines this new information with what it already knows to provide more accurate, complete answers. The responses can include references or citations to the sources of the information, giving users the option to check the original documents for more details.
When organizations combine RAG with a strong data fabric, they can feed GenAI models with relevant, up-to-the-minute information so all decisions are based on the latest insights. The transparency of this approach improves data quality and builds trust and confidence in the AI’s answers.
Don’t Get Left Behind
Employees can now “query data” as naturally as asking a question, because GenAI bridges the gap between complex datasets and the people who need insights from them. By pairing logical data management with GenAI, organizations can unlock:
- Optimizing IT: Non-technical teams can quickly extract insights without relying on technical support, freeing up in-house resources.
- Greater Agility: With easier access and understanding of data, employees can respond more quickly to changing market conditions.
- Increased Innovation: Teams are empowered to experiment and innovate using AI-generated insights.
Breaking down data silos and providing a unified virtual data access layer that operates seamlessly across a variety of data locations and formats simplifies data retrieval, enabling business users to access essential information swiftly and efficiently.
Remember, AI is only as good as the data it’s fed—and with good data, GenAI can lead organizations on the path to data democratization, where information is always actionable. Instead of being outpaced by competitors, organizations that invest in foundational data management systems will be able to utilize AI and GenAI in an impactful way to foster greater innovation, agility, and inclusivity.