Spatial Data Types
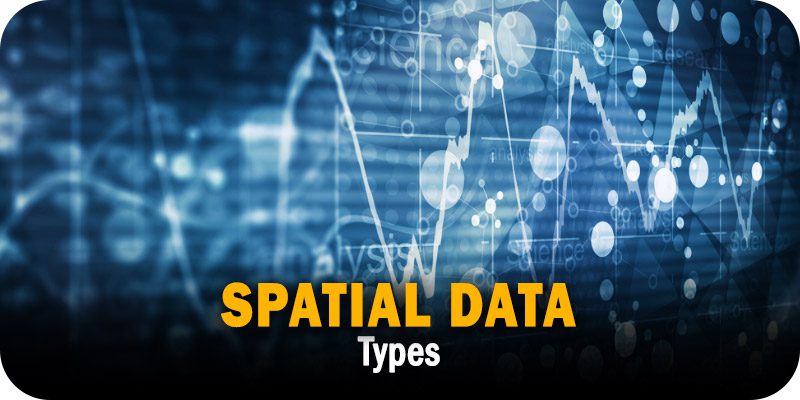
In today’s data-driven world, spatial data plays a crucial role in various industries, from urban planning and transportation to environmental analysis and marketing. Spatial data types enable organizations to represent and analyze geographic information, uncovering valuable insights and driving informed decision-making. In this comprehensive guide, we will explore different spatial data types, their significance, and how they can be leveraged to unlock the full potential of location-based data. Understanding these spatial data types will empower businesses to harness the power of geospatial analysis and gain a competitive edge in their respective industries.
Points
Points are the most basic spatial data type and represent individual locations in a two-dimensional space. They are defined by their X and Y coordinates and can be used to represent various entities, such as customer addresses, landmarks, or sensor locations. Points are the building blocks for other spatial data types and serve as reference points for spatial analysis, distance calculations, and spatial joins.
Lines
Lines, or line strings, represent connected sets of points, forming a continuous path or route. They can be used to represent transportation networks, roadways, river networks, or any linear feature. Lines are commonly used for route planning, network analysis, and measuring distances or lengths along a specific path.
Polygons
Polygons are closed shapes formed by a series of connected lines, with the last point connecting to the first. They represent areas or regions on a map and can be used to delineate boundaries, administrative units, or land parcels. Polygons are essential for spatial analysis tasks such as area calculations, spatial clustering, and overlay analysis.
Multi-Points, Multi-Lines, and Multi-Polygons
Multi-geometry types allow for the representation of multiple points, lines, or polygons within a single object. For example, a multi-point can represent a collection of customer locations, while a multi-polygon can represent a complex region made up of multiple disjoint polygons. These data types are useful when dealing with multiple spatial entities that are related or need to be treated as a group.
Geometry Collections
Geometry collections are versatile spatial data types that can contain a combination of points, lines, polygons, and other geometric objects. They are useful when dealing with diverse or heterogeneous spatial data that cannot be easily represented by a single geometry type. Geometry collections enable the storage and analysis of complex spatial relationships within a single object.
Raster Data
While the previous spatial data types focus on vector-based representations, raster data is a grid-based format used to represent continuous surfaces or images. Raster data is commonly used in remote sensing, satellite imagery, digital elevation models, and land cover classification. Raster data allows for analysis and visualization of continuous phenomena, such as temperature, elevation, or vegetation density, across large areas.
Spatial Indexing
In addition to the various spatial data types, it’s essential to highlight the significance of spatial indexing techniques. Spatial indexing structures, such as R-trees, quad trees, or k-d trees, enable efficient spatial queries and spatial joins by organizing and optimizing the storage and retrieval of spatial data. Spatial indexing improves query performance and accelerates spatial analysis tasks, especially when dealing with large datasets.
The Bottom Line
Spatial data types form the foundation for capturing, analyzing, and visualizing location-based information. By understanding the different spatial data types available and their applications, businesses can harness the power of spatial analysis to gain insights, optimize operations, and make informed decisions. Whether it’s analyzing customer locations, optimizing supply chains, or understanding environmental patterns, leveraging spatial data types is vital in today’s data-driven landscape.
By incorporating these spatial data types and spatial indexing techniques into their workflows, organizations can unlock the true potential of spatial data and drive innovation in their respective industries. Embracing spatial data and its diverse types will enable businesses to navigate the complexities of location-based information and gain a competitive edge by harnessing the power of geospatial analysis.