Synthetic Data Definition: Key Opportunities and Pitfalls Explained
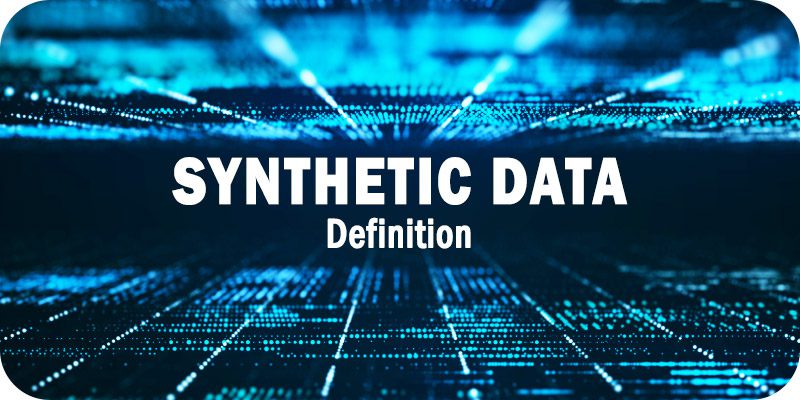
This is part of Solutions Review’s Premium Content Series, a collection of contributed columns written by industry experts in maturing software categories. In this submission, Integrate.ai VP of Machine Learning Products Roshanak Houmanfar offers a synthetic data definition and keys to consider.
Last year, Gartner predicted that “by 2024, 60 percent of the data used for the development of AI and analytics projects will be synthetically generated.” Since then, synthetic data has grown in popularity as a solution to a lack of access to high-quality, real-world data for training machine learning algorithms. But as synthetic data is increasingly used in place of inaccessible distributed data, it’s essential to ask: Is synthetic data really the right solution?
Synthetic Data Definition
When to Use Synthetic Data & When Not to
Before knowing whether or not synthetic data is the best solution for training algorithms, it’s important to understand overall when synthetic data is useful and when it isn’t.
Despite the trillions of data humans generate every day, there is still a lack of available real data. Synthetic data is best used as a solution when the modeling target has either a small amount of real data available or none at all. For example, it’s a helpful resource for cold-start problems and text and image-based model training. Additionally, synthetic data has shown value in datasets that already have standardized data between different problems– such as the presence of words and grammar in test use cases and the existence of pixels, which allow for models to abstract the essence of the data.
Synthetic data however is not suitable for use cases where real data already exists but is siloed due to privacy regulations, centralization costs, or interoperability roadblocks. Further, most of these use cases are not datasets that benefit from that unit of input in the first place, making it difficult to determine the right level of abstraction for synthetic data creation. Thus, challenges arise because the nature of the problem is fundamentally different from what synthetic data is suitable to solve.
Problems with Synthetic Data
Because there are inherently unknown aspects to most source data, generating high-quality synthetic data will always be a challenge. Think of synthetic data as a blurred image. It’s unclear how it affects the training and the results of the models learning from it, which makes debugging any issues down the line difficult.
Synthetic data also suffers from the same problem most machine learning projects suffer from— connecting the wrong questions to the wrong tools and then concluding that the model was ineffective when it could be the case that the synthetic data was simply not up to par.
Unknown bias is also a cause of concern with the use of synthetic data, as users can’t guarantee the quality of the representation it has learned. When developers can’t access the real data, and can only see a narrow view of what the real data could be, the added layer of abstraction can open opportunities for innate bias.
Finally, the use of synthetic data in machine learning training models is costly. With synthetic data, teams often need to run computations and adjust models hundreds if not thousands of times to achieve the most accurate results. Coupled with the added cost of transferring the massive amounts of data required for comprehensive training, synthetic data ultimately becomes more expensive from a time and investment standpoint compared to utilizing other methods, like privacy-enhancing technologies, that capitalize on real data from the source.
The Future is Federated
In instances where the real data exists but is siloed, solving data access challenges with synthetic data will always produce a subpar result in comparison to that which a federated learning solution can produce. Federated learning allows for superior training of AI models by sending versions of the model(s) to the data in the environment where it lives instead of requiring the data to move to the model. Because federated learning enables secure access to real high-quality data while simultaneously allowing data custodians to retain full control and security over said data, it eliminates the need for the generation and use of synthetic data in use cases where interoperability, privacy regulations, or centralization costs are the roadblocks.
Where federated learning really shines is in use cases within highly regulated industries like healthcare and finance where confidential, protected data sets are largely inaccessible to developers. Imagine a world with better cancer diagnostics, virus outbreak predictions, and fraud detection because access to data that was once unobtainable due to its sensitive nature is unlocked. Breakthrough AI advances can make such a world become reality, but that reality is reliant on access to huge amounts of data currently spread across business units, organizations, and countries– each with different privacy regulations. The ability to securely capture the value of this data exists, but it is not currently accessible beyond the elite ranks of big tech, thanks to their all but limitless resources.
While synthetic data solves the problem of a lack of access to data to train models, for most other use cases, it will be inferior in comparison to model training on data accessed with federated learning tools, which can better preserve privacy; produce more accurate results by enabling work with granular, quality source data; and avoid the inherent, added layer of abstraction that is unavoidable with synthetic data.
With federated learning, democratized access to privacy-enhancing technology breaks down collaboration barriers within and between organizations and increases access to quality data. Innovative data scientists and engineers can seamlessly build the best AI systems, with the best data, regardless of where it sits, while maintaining the highest standards of trust and security for organizations and the individuals from which said data were produced.