The Critical Importance of Data Quality: A Deep Dive
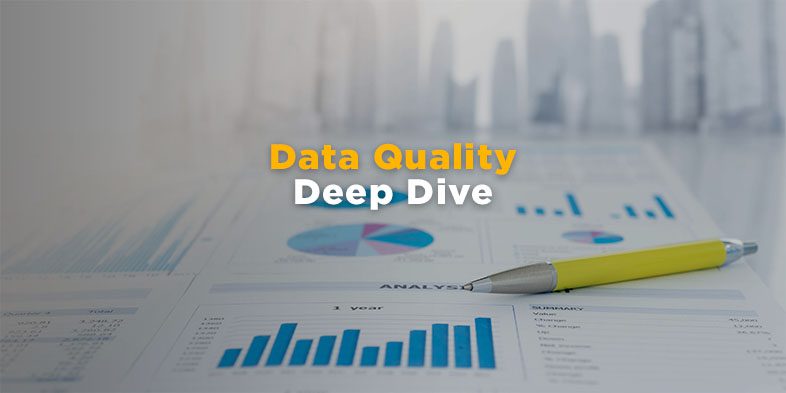
Bigeye’s Kyle Kirwan offers insights on the critical importance of data quality in this deep dive resource. This article originally appeared on Solutions Review’s Insight Jam, an enterprise IT community enabling the human conversation on AI.
Data quality is a critical factor that can make or break organizations. Consider the impact of data errors: a data mishap once led to the accidental demolition of the wrong house in Texas.
In another instance, NASA suffered a $125 million loss when different teams used incompatible measurement systems, causing the failure of a spacecraft. These examples underscore the profound impact that data quality can have on our lives and businesses.
Data quality is not just about accuracy; it’s about trust, efficiency, and effective decision-making. In this article, we’ll delve into the depths of data quality, exploring its definition, importance, and practical tips for ensuring high-quality data in your organization.
What is Data Quality?
Data quality refers to the reliability, accuracy, and completeness of data for its intended purpose. Essentially, it ensures that data is good enough to support the tasks and processes it’s used for. Data quality encompasses various aspects, including:
- Accuracy: The data must correctly reflect the real-world values it represents.
- Completeness: All required data must be present.
- Consistency: The data should be uniform within the dataset and across different datasets.
- Timeliness: Data should be current and relevant for its intended use. 5. Formatting: The data must have consistent formatting.
- Uniqueness: The dataset should not have duplicate records
- Accessibility: The data should be accessible for users in a way that balances security and usability.
Data Quality vs. Data Integrity
Data quality and data integrity are related but distinct concepts. Data quality focuses on accuracy, completeness, and consistency, among other aspects. In contrast, data integrity is about maintaining data accuracy and consistency throughout its entire lifecycle, involving processes and technologies that protect data from unauthorized changes. While data quality ensures that data is suitable for its purpose, data integrity ensures that data remains intact and unaltered.
Data Quality vs. Data Observability
Data quality is a traditional, all-encompassing term focused on fixing data issues reactively. It refers to the general state of your data: how healthy is it?
On the other hand, data observability constantly surveys the state of the data pipeline and proactively diagnoses issues. Data observability platforms can help ensure data quality by providing continuous monitoring and immediate alerts for any anomalies or issues.
Prevention Before Mitigation
When it comes to data quality, prevention is often more effective and efficient than mitigation. Preventing data quality issues at the source is far less costly and time-consuming than trying to clean and correct data after it has already entered the system. Moreover, if data quality issues are not identified promptly, they can lead to misguided decisions and actions.
Here are some of the first steps to start preventing data quality issues:
Implementing Clear and Consistent Data Entry Standards
Ensure that data is recorded accurately and uniformly from the beginning by establishing clear data entry standards. This involves defining standard formats, units of measurement, and acceptable value ranges for all data entries. Consistent data entry standards help minimize errors and discrepancies, making the data more reliable and easier to work with.
Using Data Validation Rules
Data validation rules are essential for preventing the entry of invalid or inconsistent data. For instance, using regular expressions or predefined value ranges to validate data entries can help ensure that only valid data is entered into the system. These rules act as a second line of defense against data quality issues.
Establishing Data Governance Policies
Data governance policies and practices define roles, responsibilities, and processes for maintaining data quality. Effective data governance involves assigning specific roles to individuals or teams responsible for data quality, defining data stewardship practices, and establishing protocols for data management. Clear governance policies help ensure accountability and consistency in data management practices.
Training Employees
Employees play a crucial role in maintaining data quality. Training employees on the importance of data quality and providing them with the tools and knowledge needed to enter data accurately can significantly reduce errors. Regular training sessions and workshops can help reinforce the importance of data quality and keep employees updated on best practices.
Implementing Automated Checks and Validations
Automated checks and validations within data entry forms and systems can catch and prevent data quality issues in real-time. These automated processes can include format checks, range checks, and consistency checks to ensure that data entered into the system meets the required standards. Automation reduces the likelihood of human error and increases the efficiency of data entry processes.
Conclusion
Data quality is a critical aspect of modern business operations, impacting everything from daily decisions to long-term strategic planning. By understanding the importance of data quality and implementing preventive measures, organizations can ensure that their data is reliable, accurate, and fit for purpose. High-quality data not only supports efficient and effective decision-making but also builds trust and confidence among stakeholders. As the saying goes, “garbage in, garbage out.” Ensuring data quality from the outset can prevent costly errors and pave the way for successful data-driven initiatives.