The Secret to More Accurate, Intelligent LLMs: Metadata
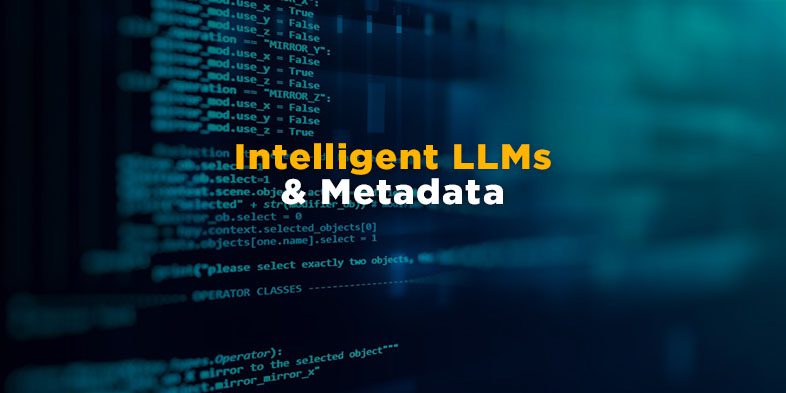
Coalesce’s Satish Jayanthi offers insights on how the secret to more accurate and intelligent LLMs is metadata. This article originally appeared on Solutions Review’s Insight Jam, an enterprise IT community enabling the human conversation on AI.
In today’s business technology landscape, artificial intelligence (AI) and machine learning (ML) are at the forefront of innovation. Generative AI and sophisticated large language models (LLMs) promise to revolutionize how businesses operate. While the possibilities for AI are vast, the risks are equally significant due to unreliable outputs and the high costs of making decisions based on poor data. Before diving into gen AI and LLMs, it’s crucial to have a strong data foundation, including comprehensive use of metadata.
What is Metadata?
Metadata is the contextual information that describes your data and is typically generated automatically. For example, when you take a photo with your smartphone, it generates a timestamp, location coordinates, device type, and file size. This metadata allows you to search your photo library effectively, turning it into a valuable database of memories.
Similarly, businesses generate metadata while building and using their data platforms. Data catalogs and glossaries are rich sources of metadata, providing context about data tables and columns. This contextual information is vital for training accurate and useful LLMs.
Enhancing LLM Training with Metadata
The initial excitement around consumer LLMs like ChatGPT led to misconceptions about their capabilities. It soon became clear that LLMs could produce unreliable outputs, or “hallucinate.” Simply feeding more data into an LLM would be one way to improve output, but that isn’t scalable or cost-effective. Instead, providing clean data with rich context—metadata—can significantly improve LLM accuracy, while keeping costs in check.
For example, to train an LLM to generate SQL, you would need to include metadata about table and column definitions, relationships, the timeliness and freshness of the data, and so on. Domain-specific LLMs, tailored to a company’s data and context, can offer more accurate and valuable insights than general-purpose models like OpenAI’s ChatGPT or Google’s Gemini.
Practical Examples of Metadata in Use
- Contextual Understanding: Metadata can include definitions and usage context for different data columns. For example, in a retail database, metadata might explain that a column labeled “Q1 Sales” refers specifically to sales figures for the first quarter of the fiscal year. This helps the LLM understand the context and provide more accurate insights or predictions.
- Data Relationships: Defining relationships between business entities–for example, customers and sales, or products and inventory–is essential for conducting complex analyses. Understanding how customer data connects with sales and marketing, and how products are linked to inventory, provides valuable insights.
- Data Quality Assessment: Tagging data according to its relevance and importance, or timeliness and freshness, and training an LLM with that additional context, will help produce more accurate outputs. For instance, a financial firm could label transaction data with metadata indicating the freshness of each data source, helping the LLM distinguish between relevant and stale data.
- Data Lineage: Tracking the origin of data through metadata allows businesses to maintain a clear audit trail. For example, a healthcare company might use metadata to track the source of patient records, ensuring compliance with data governance policies and enabling precise data lineage tracking.
- Optimized Query Performance: Metadata can be used to optimize query performance by providing additional context for query planning and execution. Engineers can use metadata to indicate which columns are indexed or frequently queried, enabling the LLM to generate more efficient SQL queries.
Building a Solid Data Foundation
A robust data foundation harnesses metadata to enhance data management and project efficiency. Modern data tools that integrate column-level metadata into the data platform architecture are key to delivering business-ready data consistently. Metadata-driven architectures can optimize query performance and reduce compute costs by providing contextual clues that simplify data processing.
When building out a data platform, it’s essential to focus on tools that capture and utilize rich metadata. This includes data cataloging tools, data quality assessment frameworks, and metadata management solutions that provide a comprehensive view of your data landscape. By leveraging these tools, businesses can create a solid data foundation that supports advanced AI and ML initiatives.
Embracing a Metadata-Driven Approach
Adopting a metadata-driven approach by selecting tools that capture extensive metadata and leveraging it to add context to your data, can shift your team’s focus from troubleshooting and firefighting to fine-tuning LLMs, launching new data projects, and driving business value.
Additionally, metadata can enhance collaboration across teams by providing a shared understanding of data assets. Data engineers, analysts, and business users can all access and interpret metadata to gain insights into data sources, definitions, and relationships. This shared understanding fosters collaboration and enables more effective data-driven decision-making.
Unlocking the Potential of AI and ML Initiatives
In conclusion, while AI and LLMs hold transformative potential, their success depends on a solid data foundation enriched with metadata. This will enable businesses to build more accurate, intelligent LLMs that provide reliable insights and drive meaningful innovation. Metadata is not just an ancillary component of data management—it is the key to unlocking the full potential of your AI and ML initiatives. Embrace a metadata-driven approach to ensure your data is well-contextualized, high-quality, and ready to power the next generation of intelligent systems.