The Universe is a Graph: It’s Time for our Data to Start Acting Like it
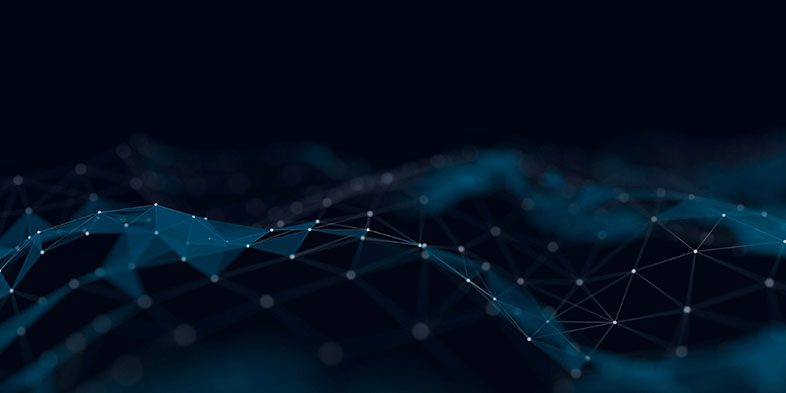
Altair’s Christian Buckner offers insights on how the universe is a graph and why it’s time our data started acting like it. This article originally appeared on Solutions Review’s Insight Jam, an enterprise IT community enabling the human conversation on AI.
Reality is not a collection of isolated entities, neatly boxed and categorized. It is a web—vast, intricate, and endlessly interconnected. From the movements of galaxies to the subtleties of human relationships, the universe reveals itself as a tapestry of connections, each thread influencing and entwining with countless others. Yet, in day to day business, when we attempt to model the complexity of our business universe, we usually rely on rigid relational data structures—rows and columns that impose artificial order on an inherently fluid world. And to accommodate this unnatural model, for decades we have built increasingly convoluted and expensive systems that shoehorn real world intricacies into the rows and columns today’s analytics tools understand.
But the world is changing, and so are the tools we use to understand it. Generative AI, with its astonishing ability to create, predict, and understand, demands a different perspective. Generative AI models generate meaningful outputs by capturing patterns, learning from rich contextual data, and leveraging complex interdependencies. Unlike traditional analytics and machine learning, generative AI doesn’t merely process datasets in isolation—it thrives on the relationships between ideas, concepts, and patterns. It begs for data that exposes the complexity of the real world, allowing it to traverse idea to idea and piece together meaning from the natural interconnectedness of reality.
In other words, generative AI needs a graph. With nodes representing entities and edges capturing the relationships between them, a graph can represent massive networks of information and provide a data structure that mirrors the interconnectedness of the real world. Unlike rigid relational models, graphs allow for dynamic connections, enabling generative AI to explore context, infer meaning, and uncover hidden patterns. Graphs can represent hierarchies, peer-to-peer relationships, temporal changes, and multi-dimensional dependencies, all in a single framework. This flexibility makes them uniquely suited to capture the complexity of reality—whether it’s mapping customer interactions, modeling supply chains, connecting field data to manufacturing data and designs, or understanding the intricate cause and effect links in life sciences research. For generative AI, a graph is not just a database; it is a living model of knowledge that it can navigate, learn from, and act upon, unlocking insights and automation that rigid data structures could never achieve.
At the same time, pragmatism dictates that we must meet reality where it stands. Today, the data ecosystems of most organizations are deeply entrenched in relational paradigms. Relational databases, document stores, enterprise applications, and other business systems form the backbone of how businesses store and manage information. Overhauling these systems entirely in favor of a graph-based architecture is not a realistic or immediate solution.
Instead, the path forward lies in building a bridge between what exists and what is needed. By overlaying a representative, knowledge graph-backed data fabric—an abstraction layer that unifies all of an organization’s data, regardless of its source—we can create a cohesive, graph-driven model without dismantling existing infrastructure. When anchored by a knowledge graph, data fabrics serve as the connective tissue in businesses, transforming disparate silos into a seamless web of relationships. They enable organizations to work with their relational systems while simultaneously presenting data in a way that aligns with the interconnected nature of reality, unlocking the potential for generative AI and other advanced analytics to thrive. It harnesses past investments while setting a foundation for the future.
Graph-based models become even more essential when we consider the role of generative AI agents in the evolving landscape of organizations. These agents are not just tools for static analysis or passive insights—they are active participants in the systems they inhabit. To operate effectively, generative AI agents will need the full context of an organization’s collective knowledge: every piece of data, every relationship, every dependency. They must have visibility into the full scope of tools and processes they can interact with to enact operational changes, automate workflows, and inform decision-making. In essence, these agents must not simply read from the graph—they must become a part of it, acting as dynamic nodes that continuously read context, update their understanding, and take actions. A graph-powered data fabric allows these agents to seamlessly navigate the complexities of organizational ecosystems, enabling them to not only analyze and predict but also orchestrate processes and collaborate with humans in a way that mirrors the interconnected nature of the organization itself.
The introduction of generative AI agents into a knowledge graph-powered data fabric transforms the very nature of what a data fabric can be. No longer just an abstraction layer to unify and present data, it evolves into something far more powerful: an AI Fabric. This AI Fabric is not simply a repository or a conduit for information—it becomes an active, intelligent network where AI agents, humans, and systems work in concert. Within this paradigm, AI agents are not just observers or analysts; they are collaborators, orchestrators, and drivers of automation. They leverage the full depth of the knowledge graph to understand context, anticipate needs, and act with precision. The AI fabric enables a new level of operational intelligence, where processes are dynamically optimized, decisions are continuously informed by real-time insights, and automation becomes not just reactive but proactive. It represents a fundamental shift in how organizations operate, bridging the divide between static data infrastructure and living, adaptive business intelligence.
Importantly, this AI Fabric not only enables extraordinary new possibilities but also provides a critical framework for managing risk. When all data, actions, and actors are part of the graph, the single governance model that controls the graph also controls all the entities. Every actor—whether a human user or a generative AI agent—is tightly regulated by one universal governance model, ensuring that only authorized entities can access or act upon specific data or processes. Moreover, the AI Fabric builds traceability and observability into its very foundation. Every action taken within the AI Fabric—whether it’s a decision by an agent, a process execution, or a human intervention—is stored as an entity within the graph itself. These action entities not only provide additional context to inform future decisions by AI agents but also give organizations complete, real-time oversight of what the system is doing at any given moment. This level of transparency ensures accountability, fosters trust, and allows organizations to harness the transformative power of the AI Fabric without losing control over the systems and processes that drive their operations.
The AI fabric represents a profound leap forward—a shift from static, siloed systems to a living, dynamic network that mirrors the complexity of modern organizations. By unifying data, actions, and actors within a single, graph-powered ecosystem, it enables a new era of operational intelligence. Generative AI agents and human users alike can seamlessly collaborate, informed by the full context of an organization’s knowledge and empowered to make real-time decisions that drive meaningful change. At the same time, the AI Fabric ensures control and accountability through universal governance, precise access controls, and comprehensive traceability. Every action is logged, every decision observable, creating an environment where innovation thrives without compromising oversight. The AI fabric isn’t just the next step in data evolution—it’s the operating system for the future.
The alternative – retrofitting generative AI capabilities onto relational data lakehouses and warehouses – unfortunately introduces inefficiencies and artificial complexity, forcing cutting-edge models to work against their nature. Instead of embracing data representations that reflect reality’s interconnectedness, these solutions continue to build on foundations that fracture it, creating brittle systems that fall short of unlocking AI’s true potential. And by doing so, they not only limit the capabilities of generative AI but also constrain the transformative possibilities it holds for businesses and society alike.
The message is clear: the future won’t wait. Graph-powered AI Fabric is the bridge to that future—dynamic, intelligent, and transformative. It’s time to stop forcing innovation into rigid boxes and start embracing the interconnected reality that defines our world. The universe is a graph. Your data should be too.