Unstructured Data Fuels GenAI, But Firms Need Help Managing
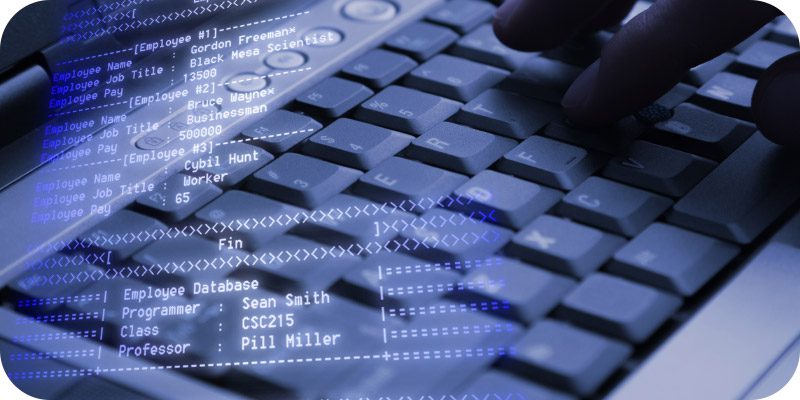
Datadobi’s Carl D’Halluin offers insights on how unstructured data fuels GenAI, but organizations don’t know how to manage it. This article originally appeared on Solutions Review’s Insight Jam, an enterprise IT community enabling the human conversation on AI.
Arguably the most important tech trend in decades, AI has soared in popularity and adoption over the past 12 months. Having hovered around the 50 percent mark between 2018-2023, implementation rates increased dramatically to 72 percent last year, according to a study by McKinsey. Expectations are also high, with three-quarters of organizations believing GenAI will lead to significant or disruptive change in their industries.
This is being translated into tangible performance improvement, and as McKinsey points out, organisations are “already seeing material benefits from gen AI use, reporting both cost decreases and revenue jumps in the business units deploying the technology.”
Despite this positive backdrop, however, there are also a range of significant risks potentially standing in the way of successful implementation. For instance, nearly three-quarters of participants in the McKinsey study have experienced data management challenges, “including defining processes for data governance, developing the ability to quickly integrate data into AI models, and an insufficient amount of training data, highlighting the essential role that data play in capturing value.”
Garbage In, Garbage Out
Given the fact that the vast majority of enterprise data is unstructured, including everything from videos and images to emails and social media content, the successful implementation of GenAI is heavily dependent on the way organizations manage these vast datasets.
The challenge is analogous to the classic computing principle of “garbage in, garbage out”, particularly because GenAI models don’t always work well with unstructured data, particularly if it has been poorly managed. As a result, organizations can easily find AI outputs skewed by poorly managed data, producing unreliable performance.
So, where does that leave businesses who see massive potential in AI, have access to unstructured data but struggle to turn objectives into tangible outcomes? The first step is to establish an enterprise-wide view of all unstructured datasets so leaders can make informed decisions about what data has potential value and, crucially, where it currently resides.
These files, which can exist in their billions, need to be identified, organized and visualized in a manner that can keep pace with rapid developments in AI systems. This can potentially be a highly complex and resource-intensive task, but choosing the correct data is critical for producing accurate, actionable and unbiased outputs.
Armed with these capabilities, organizations can empower their data scientists and accelerate the identification of the correct data to train GenAI models that address their performance improvement priorities.
This all has to take place in the context of effective data governance and a set of policies and processes that control how data is stored, documented and maintained in line with internal and regulatory requirements. Good governance also requires an ongoing commitment to data audits and continual improvement, particularly as additional datasets are added to AI systems. Done well, organizations can go a long way to minimizing the risks associated with unstructured data, from security problems and compliance breaches to poor operational efficiency.
A Change of Mindset
What’s been lacking until relatively recently are the tools to help manage unstructured data. Instead, organizations have found it much easier just to add extra storage. Given the sharp rise in data accumulation rates – in part due to the demands of GenAI systems – this approach is no longer viable. Instead, businesses need data management technology to help them stay ahead of demand. In other words, instead of focusing on the device where unstructured data is stored, IT leaders should turn their attention to how it is managed.
Effective data management technology can bridge the capability gap between raw unstructured data that contains latent value to a situation where teams can train GenAI models with high-quality data. Ideally, the data management solution used with GenAI systems will deliver seamless, reliable and efficient data migration, management and protection across heterogeneous storage environments. For organizations looking to a future where everything from strategic planning to tactical decision-making is augmented and improved by GenAI, getting the data management foundations right is crucial for success.