Why DataOps Will Set Enterprises up for Success
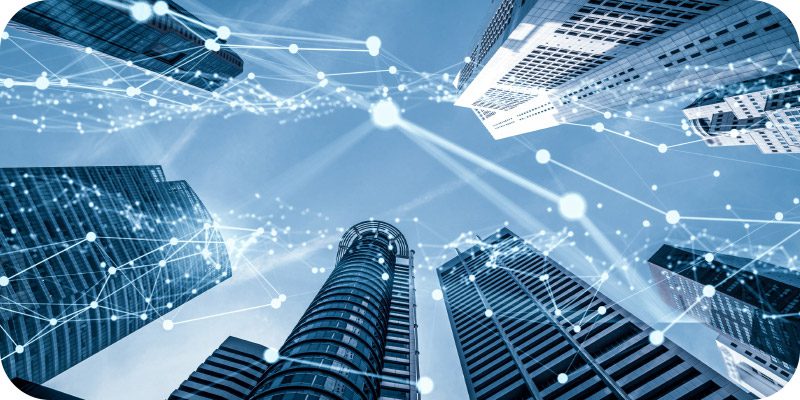
BMC’s Ram Chakravarti offers insights on why DataOps will set enterprises up for success. This article originally appeared on Solutions Review’s Insight Jam, an enterprise IT community enabling the human conversation on AI.
In recent years, companies have increased their investments in data quality, management, compliance, and regulatory adherence. This has set up organizations to take off with DataOps—the practice of applying agile methods to data in motion.
This investment has paid off. About 38 percent of North American companies employ a chief data officer (CDO), according to research from PwC, and those companies have grown faster than peers that do not employ a CDO. Similarly, companies with higher data management maturity tend to be linked to higher reported rates of success in other data-driven activities. In these organizations, gone are the days when executives would outline their informational needs, only for data analysts to retreat and reemerge 18 months later with insights.
But those successes aren’t the end of the story. To continue to thrive, organizations on the DataOps journey need to scale the results of their initial data-driven processes across the organization. To do that, they’ll need to maintain consistency, accuracy, and reliability in data handling. And deploying generative artificial intelligence (AI) can help.
Better Governance: Accuracy, Consistency, and Reliability
In practice, data orchestration boils down to something like this: Information is gathered, organized, and flows to the people who need it when they need it, whether that’s part of a self-service effort, or a data pipeline for new workflows and processes. However, operationalizing at scale, at the speed and level of sophistication that stakeholders are increasingly expecting, is an ongoing obstacle.
Organizations face several challenges. Data privacy and security requirements often limit access to data because breaches can have severe repercussions. Inaccurate, incomplete, or inconsistent data can lead to misguided insights and decisions, undermining the trust in data-driven strategies. Teams that produce and manage data may be siloed from the teams that analyze it.
Many challenges can be overcome by automating data pipelines, which makes it easier to collect, apply, and secure data for effective use. By reducing manual interventions and enhancing data processing speed and accuracy, automation significantly improves efficiency, especially in environments with increasing data volume, variety, and velocity. Automated data validation checks, detection and correction of errors in real time, minimizing the risk of inaccurate data entering the analysis pipeline. This is essential for maintaining trust in data-driven decision-making.
Implementing automated compliance checks and access controls helps organizations safeguard sensitive data and adhere to regulations, reducing the risk of breaches and ensuring consistent application of data governance policies.
In addition to technical advantages, automation fosters closer collaboration between IT and data teams. Automating routine tasks allows IT professionals to focus on strategic initiatives, while data analysts can
access high-quality data more efficiently. This alignment is crucial for operationalizing data pipelines and ensuring that both teams work towards common goals with a shared understanding of the data landscape. It also leads to faster insights and improved experiences for the organization.
Harnessing the Power of Generative AI in DataOps
Generative AI is transforming how organizations manage and use data and realize increased value from their technology investments. While many organizations are frustrated by the very real limitations of currently available public models, which are prone to errors and hallucinations, they have the power to improve it.
Most experts believe that the leading models were trained on data sets comprised of the entire internet. Because of that, there’s no easy source of written material for next-generation models. For AI to have value, it needs to be trained on high-quality data sets. The logical next step is to continue AI training with proprietary data in the enterprise, and this requires more reliance on enterprise systems.
The integration of generative AI tools into automated data workflows represents the next frontier in DataOps. These tools can augment human capabilities, providing deeper insights and identifying patterns that might be missed by traditional analysis methods. Generative AI enhances DataOps by providing expert system insights, real-time issue summaries, natural language event correlation, issue clustering, improved chatbot interactions, streamlined knowledge sharing, accelerated development and DevOps processes, and predictive insights for proactive management.
The Path Forward
Data and analytics have become key players in the enterprise, but there is more room to grow, and the changing technology landscape offers even more opportunity. Despite a significant increase in data maturity at many organizations, vast amounts of data are underutilized for the purposes of insights because companies do not have a way to operationalize it. Simultaneously, few organizations have done more than scratch the surface of generative AI.
For technology executives, the message is clear: organizations can’t unlock value from their data assets without AI. To stay ahead in the competitive landscape, it’s essential to invest in automation, AI, and specifically, generative AI, and to integrate them seamlessly into a DataOps strategy. This approach will not only help organizations overcome current challenges but also position them for long-term success.