What is a Data Lake and How Can it Improve Your Business?
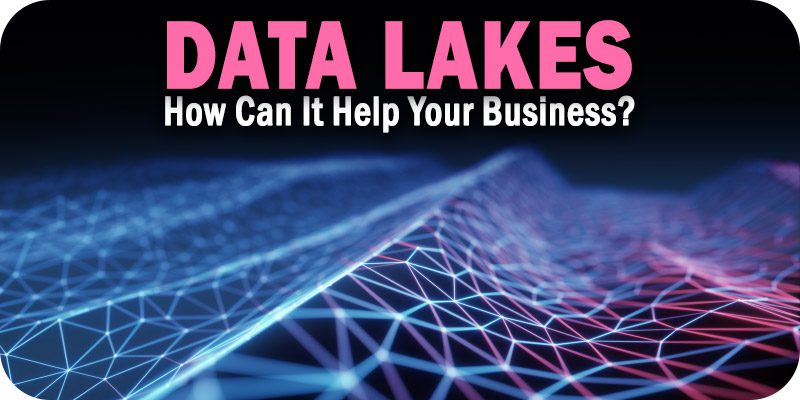
As part of Solutions Review’s Contributed Content Series—a collection of articles written by industry thought leaders in maturing software categories—Craig Kelly, the Vice President of Analytics at Syntax, shares some insights on data lake implementation and the value it can add to an organization’s existing enterprise technologies.
The term “data lake” was first coined over a decade ago. Since then, industry-leading research consultancies from PwC to McKinsey & Co. have championed the concept as a means for better data-based decision-making. At a high level, a data lake is a cloud-based centralized data repository. The term “lake” refers to how data is stored—raw and unrefined information populates a data lake, making for an extensive (but not necessarily clear) body of water. As organizations’ data volume expands, data lakes help improve information quality, access, and usability.
Despite the splash data lakes have already made, there’s still quite a bit of confusion about how the technology compares to traditional data stores, the benefits, and what’s involved in implementation. Fifteen years in enterprise data and analytics has given me more clarity than most to wade through the waters of data lakes. Let’s dive in.
More Data Doesn’t Always Equal Clearer Insights
As of 2021, the world produces five exabytes of data every day. That number is expected to skyrocket to 463 exabytes per day in just four years. For context, the most recent iPhone’s maximum storage option is one terabyte. One terabyte is the same as 1000 gigabytes (most iPhones only have 64GB), which is .000001 of an exabyte.
That’s more than a 9,000-percent increase in data growth in just four years. Imagine the amount of data your organization produces today and multiply that by 92.6. That number is roughly how much information you can expect to generate by 2025. All of this data growth must be a good thing, right? After all, data fuels an enterprise’s Business Intelligence (BI) and business analytics (BA) programs which helps companies make better, faster, and more informed decisions.
While more data can be beneficial, it’s not a given. Organizations’ data volume and sources are growing faster than most know what to do with it, meaning most organizations aren’t getting the total value out of their data. In fact, between 60-percent and 73-percent of all data collected by enterprises is never analyzed and remains untouched.
Without a convenient way to collect, store, and access data, organizations miss out on opportunities to drive profitability, operational efficiency, and innovation. But with more data than ever coming from dozens of distinct sources, enterprises need a more strategic place to flow their data into.
Data Lakes vs. Traditional Solutions
As data grows, so must an organization’s data store maturity. Otherwise, you won’t be able to take advantage of the data you already produce and the benefits that come with data-driven decision-making.
Before diving into what a data lake is and its advantages, let’s first discuss the two most common data store methods and how these traditional solutions aren’t measuring up.
- Excel Spreadsheets: Good old-fashioned spreadsheets are useful for many things but not necessarily a best-in-breed data and analytics program. While analysts can use spreadsheets to run business analytics, their capabilities are limited. Spreadsheets only provide a fixed and backward-looking review of your data, are prone to inaccuracies and user errors, and require manual maintenance. They’re also limited to the processing power of wherever the spreadsheet software is running. Plus, because of how easily spreadsheets can be compromised, spreadsheets shouldn’t be used as a primary method of storing data or running business-critical analytics.
- Data Warehouses: Data warehouses are a centralized repository of data collected from one or more disparate sources. This technology was highly innovative when first introduced in the late 1980s/early 1990s. However, it has a few limitations considering the amount of data we produce today. Namely, warehousing requires data to be defined upfront, which can be time-consuming and frustrating for organizations that want to store large amounts of unstructured data. It’s also not the most cost-efficient solution since ongoing RAM, memory consumption, hardware, and maintenance can add up quickly.
Unlike these dated models, data lakes offer a more modern path for storing large datasets for enterprise-wide analysis. The primary benefits are that it improves data accuracy and access, produces more mature insights, reduces manual efforts, and has a relatively low total cost of ownership.
A data lake is a centralized cloud store that can house large amounts of structured and unstructured data from on-premises or cloud-based systems (ERPs, CRMs, HR systems, or IoT devices). It uses a flat architecture that allows data to be stored in its native format, making the solution cost-efficient, easy to implement, and maintain.
The centralized structure improves data democratization across the entire organization and allows organizations to apply machine learning (ML) and artificial intelligence (AI) algorithms to produce mature insights. In fact, of organizations currently using a data lake, 87-percent have experienced improved decision-making ability.
Finally, data lakes are highly cost-efficient and allow organizations to scale as needed. For example, with a long-term data archiving plan from AWS, storage only costs organizations $0.00099 per GB.
Vendor Evaluation and Implementation Considerations
If you’re ready to embrace data lakes, you should know a few things about the adoption process. If data lake implementation goes successfully, it should only take a few weeks to complete your project and have your lake start producing actionable insights. By comparison, a data warehouse project typically takes months to set up servers, install the software, and ensure compliance with the operating system, including ongoing maintenance and upgrades.
When looking at data lake cloud vendors, due diligence about the security, management, and search capabilities vendors offer is essential. Otherwise, you’ll have to build out that functionality yourself or outsource it to a third party. For example, AWS provides AWS Lake Formation, which helps organizations operationalize their data lakes. The service enables you to collect and catalog data from databases and object storage, move the data into your data lake, clean and classify your data using ML algorithms, and secure access to sensitive information via hyper-granular controls.
Once you’ve made your vendor decision, you’ll want to work with a trusted cloud and analytics partner who can help with planning, mapping, and implementation to ensure your data lake solution is optimized for your existing tech environment. Check if they’re certified for your specific ERP system, database environment, and data lake solution cloud vendor.
Let’s Go Swimming
Data lakes have been around for long enough that the results now speak for themselves. Organizations with solid data lake practices outperform data lake followers by 9-percent in organic revenue growth. Imagine the difference compared to organizations that have no investment in data lakes.
Ultimately, the value of a data lake lives in its ability to access high-quality data without the manual effort or cost involved in traditional solutions. Instead of collecting data for it to lay around collecting metaphorical dust, you can put that information to use, driving profit, innovation, and competitive advantage for your company and its utilization of ERP technologies.