A Beginner’s Guide to Advanced Analytics
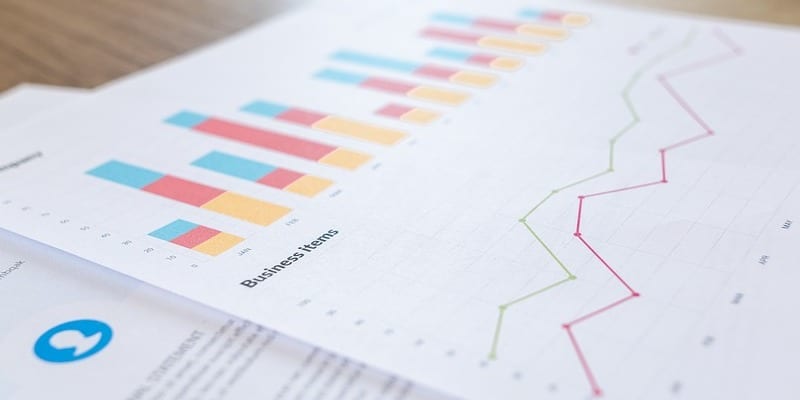
Analytics acts as the bridge between data and business intelligence. A data science, analytics is the practice of studying data assets to identify trends and make informed business decisions. With increased adoption of analytics in the enterprise, the playing field among digital businesses is leveling. As a result, organizations are looking for new ways to regain a competitive advantage. To do this, more sophisticated measures are needed. These tactics are referred to as advanced analytics. How is the ‘advanced’ in advanced analytics defined?
Data mining and discovery
Making predictions requires relevant data, and oftentimes, this requires manual digging. A lot has been made in recent years about the “volume and velocity” of big data that organizations want to analyze, and advanced BI tools provide a way of sifting through enterprise data in a way that is conducive to prediction. In order for data analysts to have any faith that their predictions are correct, they first have to get granular in choosing which pieces they analyze.
Before the data can be mined and prepared for analysis, it has to be located. Data science tools that provide search-based discovery capabilities can uncover data that was once too buried to see. Data analysts use this technology to develop and refine views of different types of data so that only relevant data is grouped together. This ensures that the data being analyzed is the data that will help a business answer a specific question.
It’s all about prediction
Predictive analytics takes the process one step further. Where traditional analytics is the process of using historical data to make more informed decisions in the future, predictive models attempt to predict the future. In effect, organizations that use predictive tools wind up creating a simulation of future conditions in order to run through different scenarios in hopes of coming to conclusions before their competitors.
The accuracy and usability of predictive analytics is dependent on how granular the analysis is and the type of assumptions that are being made. It could be argued then that advanced analytics are a product of the success businesses have with traditional BI. Predictive modeling is especially useful in a real-time environment where the data that is being analyzed is created in the present time.
The future lies in automation and machine learning
Machine learning is a type of artificial intelligence that allows digital interfaces the ability to learn without being programmed. Machine learning is a major focus in many fields, and zoning in on the development of applications that can change when exposed to new data. Similar to data mining, smart machines may wind up being an integral part of the prediction process given the proliferation in data volumes.
Automation and machine learning go hand-in-hand. Automating the analytics process with smart machines frees up time for data analysts to focus on the questions they want answered, not the day-to-day grind of discovering, cleaning, prepping and analyzing data assets.