A Five-Minute Guide on Augmented Analytics for CDOs
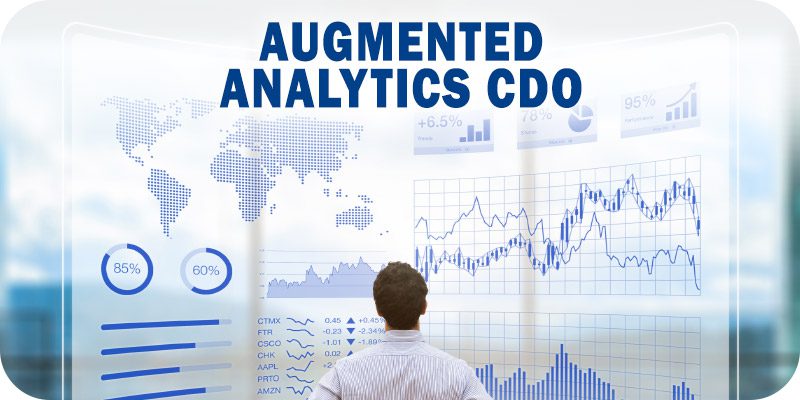
This is part of Solutions Review’s Premium Content Series, a collection of contributed columns written by industry experts in maturing software categories. In this submission, Exasol Senior Product Manager Eswar Nagireddy offers tips on how CDOs can best utilize augmented analytics to drive business value.
Rapid digital transformation has shifted company culture towards a more data-driven approach to business. Foundry’s 2022 Data & Analytics report identified that 88 percent of IT decision-makers believe data collection and analysis will likely transform business operations for years to come. This shift is fueled primarily by the proliferation and democratization of data, as well as the speed at which it is created and consumed. Subsequently, the amount of data analyzed is also expected to increase as organizations grapple with larger, more complex data sets.
While many realize the value of data for business, data management remains a key challenge. As businesses of all sizes continue to become more data-driven, business leaders will expect rapidly paced data-driven outcomes that improve the bottom line, despite business processes that lag behind.
Chief Data Officers (CDOs) are often the lynchpin between data-driven success and failure, but the reality is, technological or logistical challenges tend to hold them back. As the time to data continues to be a key differentiator, CDOs must leverage all the available tools and resources in their current tech stack, to help streamline operations, especially data analysis. This is where Artificial Intelligence (AI) and Machine Learning (ML) play an integral role in working smarter, not harder – enabling data teams to get more value from data in possession, and in a faster amount of time.
Why waste any more time or resources? Here’s how CDOs can better leverage current AI and ML capabilities to improve business performance right now:
Augmented Analytics for CDOs
Accessing Data Quality and the Business Impact
There is no one size fits all approach to working with data. Still, the more data accessed, the better-informed organizations can be to mitigate risks, drive growth or achieve other business objectives.
Presently, the responsibility lies with the CDO to implement a robust data strategy. This strategy should bring awareness to the context, risks, and opportunities surrounding the data. An optimal data strategy must first take into account that every organization manages, distributes, and consumes data differently. Simultaneously, data quality should also be factored into the strategy – is it continuous? Is it missing any critical details that could best support their decision-making process? Are there duplicates?
One key factor inhibiting an effective data strategy is that real-world data is often not clean. In fact, according to Deloitte’s Analytics Advantage report, more than half (66 percent) of a business’s data is considered low-quality. If the information is not high quality, teams can easily get bogged down, spending most of their time cleaning and prepping the data for analysis. Since data is often created and shared across multiple systems, external data can significantly impact the internal decision-making process, leading to additional time spent cleaning and accessing the data quality.
Understanding the Role of AI/ML in Analytics
Automation can play a critical role by reducing human involvement in data analysis. As a result, data teams have newfound time to focus on actionable challenges, instead of preparing the data for these insights. This then allows organizations to improve data access flows and gain commonality across various points of view at scale in a timely manner.
When the data quality is considered to be good and reliable, automated machine learning (AutoML) can help accelerate insights and automate specific processes. This automation saves time for various teams, thereby saving significant revenue. One known downside to AutoML is that every possible model is tried before selecting the best model for a given problem.
Most AutoML platforms are black-box models – model explainability is difficult, which is a crucial part of many ML projects. While using ML, the outcomes or predictions are not the only criteria to look at, but also the details of the logical approach. Such details include: feature derivation, feature importance, the confidence of the prediction, etc. If an AutoML platform could provide such intelligent insights, it would accelerate the time to insights process.
Using AI/ML to Improve Data Access Flows & Establish a Single Source of Truth
To get data-driven actionable insights, the data must go through a multitude of transformations. Some of these transformations can be handled manually, while others are automated. Manual processes require domain knowledge and understanding of the data. Intelligent manual processes can be automated with AI/ML, with examples including data extraction from PDFs, data labeling, or classification. These ML applications can accelerate data flows, saving a considerable amount of organizational time.
Improving data access flows can help organizations reach their target audiences, including prospects, partners, and customers while making the best decisions possible to support and optimize business processes. A robust data flow can boost an organization’s data maturity level, thereby driving them toward a data-driven culture.
AI/ML can not only help clean and resolve the data but also create a single source of truth for the entire business. Once that’s established, various points of view can be built on top of a single source of truth. To achieve this, organizations can isolate a single source of resolved truth, with everyone working towards the same mission or goal.
Ultimately, understanding and utilizing AI/ML to its full potential in data analysis can be the difference between a data-driven organization and one that is lagging behind the competition. Automation is the key to a robust data strategy, one that includes self-service data analytics tools, flexible data flows, and access designs. As CDOs leverage AI/ML to their full advantage, a greater number of individual teams within an organization can help contribute to the data journey, creating that data-driven culture that so many strive for, but are yet to accomplish.
What’s stopping you?