Forrester: The Top 6 Steps to ‘Do’ Analytics
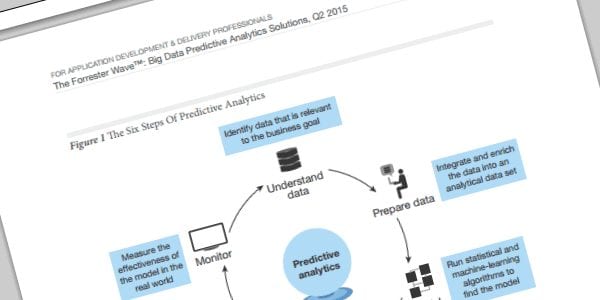
In their most recent Wave study on big data and predictive analytics, Forrester outlines the six steps organizations need to take in order to “do” predictive analytics. This may be important to companies who are looking to get serious about pulling insights from the data they generate.
By nature, using the power of predictive analytics in your organization is not a one-time operation. Over and over again, businesses must confront the challenge of making something out of their data. As new data and updated solutions become available, companies must be willing to re-evaluate on the fly if they are to get every last drop of utility out of their BI solution.
Identify data from a variety of sources
Data can exist in locations that are hard to access, both within organizations and outside of them. Given that any scrap of data has the potential to add valuable insight, locating this information should be important. Advanced data visualization tools can help businesses explore data from the various sources to determine what might be of worth and should be used in a predictive analytics project.
Wrangle the data
Preparing data for analytics is an important challenge. According to Forrester, many users of these solutions spend more than three-quarters of their time prepping the data for use. This includes calculating aggregate fields, removing unwanted characters, filling in missing data, or merging data sources together.
Build a predictive model
The solutions outlined in the report all include various forms of statistical and machine-learning algorithms that data scientists can choose to run within their organization, depending on need. The best algorithms to run depend on the type and completeness of the data and the type of prediction the user wishes to come to. Analysts typically run analysis on a subset of data called “training data” and set aside “test data” that they plan to use in evaluation of the model.
Evaluate the model’s effectiveness and accuracy
Predictive analytics is about probabilities. To evaluate the predictive power of a model, data analysts use it to predict a “test data” set. If the model can handle predicting the test data set in way that is satisfactory, then it is a candidate for deployment.
Use the model to deliver actionable prescriptions to your business peers
Predictions have little value if they cannot seize a predictive opportunity and help an organization to avoid a negative event. If predictive analytics is the chosen path of a company, then the predictions the model creates need to be trusted. In that, those who create the models need to learn from their peers when it comes to implementing actionable insights.
Monitor and improve the effectiveness of the model
Predictive models can only be as accurate in their findings as the information that is fed into them are. In order to keep these models relevant, the data that is being input should also be closely watched over time. By adjusting the model or seeking additional data, the model should continue to function as a source of business insight as time goes on.