Future-Proofing Clinical Data Infrastructure: The Evolution Towards a Data Lakehouse Architecture
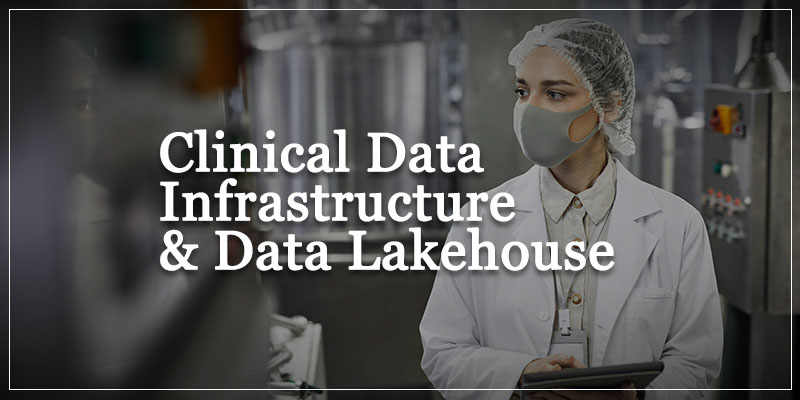
Solutions Review’s Contributed Content Series is a collection of contributed articles written by thought leaders in enterprise technology. In this feature, eClinical Solutions’ VP of Global Strategy Venu Mallarapu offers commentary on the evolution towards a data lakehouse architecture in clinical data infrastructure.
The life sciences industry faces intense pressure to accelerate clinical research timelines to keep pace with the speed of innovation in science and medicine and above all, deliver therapies to patients in need. Personalized medicine, digital trials and decentralization increase data volume from external data sources such as biomarkers, labs, wearables and more. Trials routinely use at least six external data sources, with many incorporating over 10. With increasing trial complexity comes increased data challenges. Today’s clinical trials demand innovative approaches to handle the exponential growth in data volume and diversity. Accurate and timely data insights are necessary for faster and better decision-making to reduce cycle times, improve productivity, scale operations, and develop new breakthroughs.
Driven by the need for speed and efficiency, the clinical data technology landscape is also transforming. Removing fragmentation and silos is very critical to accelerate this transformation. End users must have access to data and tools that facilitate risk-based approaches, collaboration, and automation in place of inefficient, manual methods for interacting with data. In addition to addressing the data challenges of today, modern research demands modern, scalable technology that supports the science and data needs to come. The ways in which trials are conducted, including how data is managed, must advance accordingly. As decentralized clinical trials (DCTs) and novel trial approaches become more widely adopted, traditional approaches to data acquisition, aggregation, cleaning, and analysis must be re-evaluated.
Clinical Data Infrastructure & Data Lakehouse
Data is the currency of the life sciences industry, and streamlining data and analytics pipelines is critical to reducing cycle times in research overall. Accordingly, data technology architectures within research continue to evolve to address current challenges and pave the way for future requirements. While traditional “Data Warehouse” and “Data Lake” architectures have historically played an important role in the industry to enable data aggregation and analytics, they have their shortcomings in data accessibility, quality, scalability, and cost.
Data Warehouses, an information storage architecture that stores structured and processed data, aim to centralize data but can create data silos. This can make it challenging to ensure the data in the warehouse is up-to-date and consistent, which in turn makes it difficult to adapt to changing requirements.
On the other end, the Data Lake architecture is a centralized space for raw and unprocessed data, which is ingested “as is” from various data sources. This data is not validated, cleansed, or checked for accuracy. Therefore, insights generated from such data cannot always give an accurate picture for reliable decision-making. While Data Lakes can improve capabilities by integrating cloud technologies and are cost-effective for handling large data sets, they still lack strict schema enforcement for types and formats of data, and finding data can become problematic.
Due to these disadvantages, it’s time to herald in a new architecture that takes the best from both worlds: the “Clinical Data Lakehouse.” Data Lakehouses aim to address the limitations and challenges associated with existing data architectures and create a unified architecture that combines capabilities from both. What makes Data Lakehouses unique is their ability to store both structured and unstructured data in its raw form, compared to previous structures, which relied on either structured and processed data (Data Warehouse) or raw and unprocessed data (Data Lake). This provides clinical researchers with a single repository for diverse data types, including relational, semi-structured, and unstructured data. By leveraging a Data Lakehouse, researchers can access the most complete and up-to-date data.
In adopting the Clinical Data Lakehouse, life sciences organizations are equipped with a more comprehensive and flexible data infrastructure – one designed for data democratization and accessibility in a regulated environment. This blueprint enables companies to derive critical decision-making insights from diverse data sources and also address existing challenges with data storage, processing, and analytics. Additionally, Data Lakehouses support the use of emerging techniques, including Artificial Intelligence (AI) and Machine Learning (ML), which is pivotal for the acceleration of data science.
AI adoption has become a priority within the life sciences space. As AI has matured, it offers the potential to significantly accelerate drug development and discovery and enhance quality while reducing costs, ultimately improving patient outcomes. ML’s advanced algorithms enable researchers to detect and predict adverse events, which can help them identify potential safety concerns at a faster rate so they can intervene and take appropriate action. Using AI and ML techniques, researchers can also learn from more complex sets of data and uncover patterns in that data that would be challenging to identify otherwise. AI/ML models require large quantities of high-quality data for training and validation. As AI continues to advance and becomes more heavily relied upon, having a data foundation that can keep pace is key. A Clinical Data Lakehouse architecture supports the continued rapid evolution of life sciences R&D with a unified clinical data repository complimented by novel technology capabilities for real-time and advanced analytic workloads.
A Clinical Data Lakehouse architecture supports the use of AI/ML, reduces data silos, and increases performance, flexibility, and scalability, making it the ideal technology blueprint for continued data digitization in support of modern clinical trials.