AI & the IT Data Problem: 3 Key Things to Know for 2024
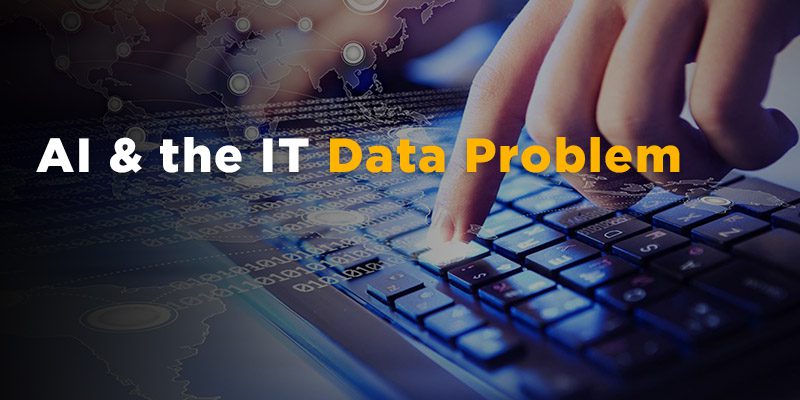
Solutions Review’s Contributed Content Series is a collection of contributed articles written by thought leaders in enterprise tech. In this feature, Semarchy‘s Brett Hansen offers commentary on AI and the IT data problem, featuring keys to know for success.
IT leaders are all too familiar with the complications of our modern data landscape. Data silos and general disarray lead to misinformed decision-making, and the direct costs of disorganized data can stack up quickly. For example, a single duplicative record costs its hosting organization $100 to store. Now, imagine those costs multiplied across an enterprise’s vast footprint.
Organizational data problems are especially poignant right now because they’ll only get worse with time. By 2025, the IDC anticipates global data will swell to 175 zettabytes, a significant leap from where we stand today. At that time, organizations will need a coordinated data storage and management strategy. Otherwise, they stand to lose out on lucrative market opportunities — or worse, fall behind their data-savvy competitors.
Which technologies, procedures and attitudes can data leaders adopt today to understand, categorize and organize their organizations’ vast data stores? Contrary to certain hype cycles, the answer does not lie in burgeoning AI applications like generative AI (GenAI) — at least, not in isolation.
Good Data Practices Will be Imperative in 2024
Modern businesses produce massive amounts of data. Why? Because every digital activity generates data, from everyday interactions like customer purchases and social media engagements to intra team organization tools and workflows. According to Statista, 328.77 terabytes of data are generated, captured, copied and consumed daily, with organizations leading the charge. This degree of data overload can be genuinely disastrous without a proper management and storage strategy.
For example, imagine a global food distribution company with 500 facilities. Tracking and maintaining inventory across so many locations can be challenging, especially when facilities are geographically diverse and the organization has no central data repository. Without the right data management strategy, top executives from this enterprise may not know when facilities are failing to meet their service thresholds. Worse — but equally common — executives may not even know the location of all of their facilities. Understandably, both of these scenarios make strategic decision-making difficult.
This is just one simplified example of the danger posed by data disorganization at scale. However, a lack of data hygiene is problematic for organizations of all sizes. For instance, while larger enterprises may face the additional challenge of synthesizing data from different offices or regions, smaller organizations grapple with data silos created by disparate team workflows. And in many cases, smaller teams may currently lack the resources to cleanse and understand their data.
For many non-technical leaders, GenAI is an attractive “solution” to bad data. But, word to the wise: Look before you leap into advanced analytics and large-language models (LLMs). Although these tools are fruitful, they alone cannot tame data disorganization.
GenAI is an Effective Data Management Partner — but Not a Leader
GenAI promises to reshape how we work. Even in early applications, GenAI integrations have contributed to a 14 percent uptick in productivity among customer service representatives, according to a study by the National Bureau of Economic Research (NBER). Similar results have been replicated by other studies and in other industries.
However, leaders won’t see similar results in their organization unless they set AI integrations up for success.
The truth is that GenAI is not magic but rather an advanced set of algorithms capable of parsing, extracting and understanding data at scale. In other words, while capable of creating compelling outputs, AI technologies are fundamentally constrained by the information they access. If that information is disorganized or outdated, GenAI may produce incorrect, potentially harmful outputs.
We’ll use another example to illustrate. Let’s say a financial institution (FI) relies on AI to identify promising loan candidates. To be a good candidate, banking clients must have a strong credit score and lending history. But this FI has sprawling data reserves that haven’t been verified in over a year. Resultingly, the system has erroneous, outdated records about John Doe that indicate he is a strong candidate — when, in fact, he is three months overdue on his current loan repayments. AI systems cannot understand or identify this discrepancy, and therefore, these systems may replicate the incorrect information, leading to an ill-informed loan strategy on the bank’s behalf.
This example evidences why it’s critical to adopt a cohesive data management strategy before rolling out GenAI integrations. Are GenAI and its attendant benefits still a priority for your organization? Then it’s likely time to consider the utility of a master data management (MDM) platform.
The Power of MDM at an Enterprise Level
MDM platforms complement GenAI by reinstating data’s trustworthiness and equipping AI with the foundational understanding necessary for accurate suggestions. These platforms compile, interpret and sort data from various sources into one cohesive platform (aka a single source of truth).
Additionally, MDMs maximize the benefits of AI by encouraging:
- Quality — MDMs ensure AI models have access to accurate, consistent and updated data, allowing for more reliable training.
- Governance — MDMs enforce data governance policies, ensuring that data used in AI applications complies with regulations and ethical standards.
- Context — MDMs provide context that is essential for AI systems to understand the relationships and hierarchies within data, enabling more sophisticated and accurate insights.
- Stewardship — MDMs promote data stewardship by managing data throughout its lifecycle. AI applications require ongoing data curation and management, so stewardship is vital.
- Interoperability — MDMs facilitate interoperability among different systems and data formats. This seamless integration allows AI applications to draw upon diverse data sources without compatibility issues.
- Scalability — As AI initiatives scale, MDM systems can manage the increasing complexity and volume of data.
When your organization’s data is organized, high-quality, well-governed, contextualized, stewarded, interoperable and scalable, your GenAI integration’s outputs will be, too. However, these benefits cannot arise in a vacuum.
Leaders must ask themselves if their systems and data are prepared to operate alongside advanced AI technologies. Without an MDM or a comprehensive data management strategy, the answer is almost certainly no. And if the answer is no, deploying GenAI throughout your organization will probably do more harm than good.