Demystifying AI: Bridging the Explainability Gap
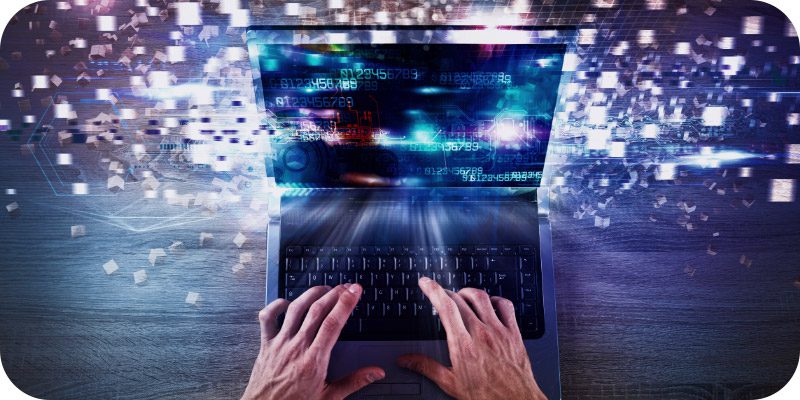
Solutions Review’s Contributed Content Series is a collection of contributed articles written by thought leaders in enterprise tech. In this feature, Neo4j Chief Product Officer Sudhir Hasbe offers commentary on demystifying AI by bridging the explainability gap.
The era of Artificial Intelligence (AI) has heralded remarkable advancements in technology and business transformation. However, it has also ushered in a new era of skepticism and concerns regarding the reliability of AI-driven decisions. How can we bridge the gap between the seemingly inscrutable nature of AI and the trust it demands from users? In this article, we delve into the need for AI explainability and explore how graph technology might hold the key to shattering the enigmatic façade that obscures AI’s decision-making processes.
For those deeply immersed in the practical applications of AI, the issue of explainability continues to be a question mark. It’s a challenge that extends far beyond curiosity and is a matter of trust. Understanding why AI makes a specific decision or recommendation is pivotal, not just for transparency but also for user acceptance. To tackle this challenge effectively, we must break down the concept into three core facets.
3 core facets of “Explainability”
- Data: What data was employed to train the AI model, and what was the rationale behind this choice of data?
2. Predictions: What features and weighting factors influenced a particular prediction or decision?
3. Algorithms: What are the inner workings of the algorithms, including the various layers and decision thresholds that drive predictions?
Data Integrity
Even with the most cutting-edge AI systems at our disposal, data integrity remains the linchpin of trust. If the data utilized in AI model training has been tampered with or manipulated in any way, it casts doubt on the AI’s competence and fairness. Therefore, understanding the lineage of data, its transformations, and the identities of those who have interacted with it is imperative. This is not merely an academic exercise; it’s necessary in numerous domains where AI is increasingly becoming a decision-making partner. Fields like healthcare, credit risk assessment, and more, increasingly rely on AI to augment human decision-making. To ensure the responsible application of AI in these fields, we must be able to explain and defend AI-driven decisions.
The Role of Graph
This is where graph database technology emerges as a potential game-changer. Graph databases offer an ideal framework for tracking data lineage, changes, and usage patterns. This capability is not just theoretical; it has already found practical application in ensuring data compliance with regulations like the General Data Protection Regulation (GDPR) and the California Consumer Privacy Act (CCPA). By extending similar principles of data lineage to AI applications, we could potentially unravel the question of AI decision-making. Graph technology inherently incorporates context and connections, making it indispensable for enhancing the applicability of AI in complex scenarios. Furthermore, it acts as a sentinel against the manipulation of input data, often a nefarious tactic employed in corporate fraud and other malicious endeavors.
Consider an energy supply system or a voting application. In these critical domains, we might have unwavering confidence in the monitoring software, but if the input data is unreliable or has been tampered with, the entire system becomes suspect. Access to explainable data, where the history and integrity of data are verifiable, is paramount. It assures us what data was used to train the AI model and why it was chosen. Achieving this level of transparency requires adopting a graph database approach. This approach not only facilitates the tracking of data changes and usage but also provides ‘explainable data’ across all dimensions: data, predictions, and algorithms.
Graph databases come to the fore in another significant aspect of AI explainability: understanding the chain of data changes and their subsequent ripple effects. They excel in helping us trace how data is transformed and the implications of these transformations. Additionally, graph technology holds immense potential in the realm of explainable predictions. For AI to gain widespread acceptance and fulfill its transformative potential, it must be more than a black box. People are naturally inclined to trust what they can understand and explain. If AI remains inscrutable, users will be hesitant to embrace its recommendations and insights, even if they could prove to be highly valuable.
Conclusion
The potential of AI is vast, and unlocking it necessitates pragmatic solutions to the explainability conundrum. Employing graph technology as a powerful ally to enhance AI explainability aligns with this objective. Whether you are embarking on an AI initiative or seeking to harness the full potential of AI in your organization, consider graph technology as a strategic tool to provide the context and clarity required to break through the ‘hidden glass ceiling’ of AI. In doing so, we can pave the way for AI to become not just a powerful tool but a trusted partner in our decision-making processes.