GenAI & Data Quality: Strategy, Planning & Success
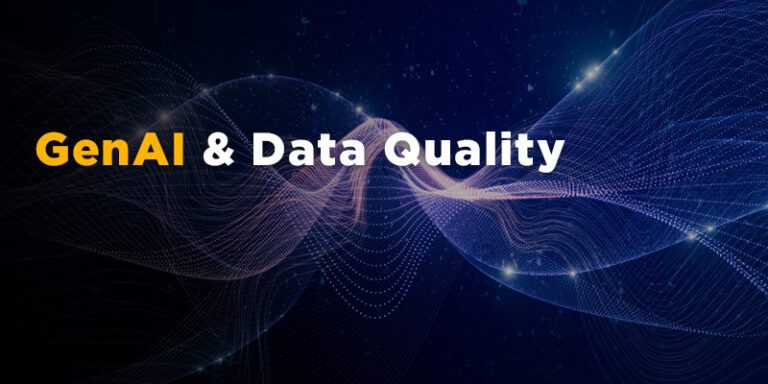
Solutions Review’s Contributed Content Series is a collection of contributed articles written by thought leaders in enterprise tech. In this feature, Syniti‘s Rex Ahlstrom offers a quick commentary on GenAI and data quality, and how to deploy a successful data strategy.
The latest shiny object of the tech industry is generative AI. ChatGPT racked up 100 million monthly active users in just two months – the fastest-growing consumer app ever. And in a Forbes Advisor study, 97% of surveyed business owners think ChatGPT will benefit their businesses. More than 30% of those businesses intend to create website content using ChatGPT, and 44% of them intend to translate that content in multiple languages.
Businesses from all industries are considering how they might use this technology to get the competitive upper hand. But organizations need clarity about the purpose and potential benefits of implementing generative AI before they begin. If not built on a firm foundation, generative AI can quickly become an expensive house of cards.
That firm foundation starts with data. Successful implementation and use of generative AI is rooted in your organization’s data strategy. Quality data is what underpins this and can make or break an organization’s AI investments.
Generative AI and Data Strategy: Making the connection
Carefully consider the goal for using generative AI. If it’s to address specific business challenges, it’s crucial to consider how you’re going to train the models and look at the data that you’re delivering in order to reach the outcome you want.
Generative AI models are trained on data. Quality data is the essential ingredient for getting results that are in line with your desired business goals.
It’s the classic “garbage in, garbage out” situation. If the quality of the data sets you intend to use to train a model or obtain insights from is poor, you’ll have a poor outcome. Issues like erroneous recommendations and off-base insights will likely result from using low-quality data.
Creating a solid data strategy involves two things: quality and context.
Organizations must start collecting and documenting data, metadata, procedures, business processes and business rules as part of their data quality programs. These essential elements are necessary for AI models to produce accurate and insightful results. By investing in initiatives to enhance data quality, businesses can build a solid foundation for the application of AI.
The context of the data is important, too. How can you be sure you’re selecting the right data sets and inputs? Your results will be useless if you have high-quality data but the wrong data. To make sure you can make the most of generative AI, you must combine good data curation with high-quality data.
Prepare for Training
As previously stated, you have to begin with clarity about the purpose of your generative AI project. Do you intend to use these systems to supplement human workers? Do you require generative AI to assist with laborious tasks? For example, creating data quality criteria for your ERP system or learning more about the data in your ERP or other system often requires a lot of backend effort. Could a model be taught to perform this task? The majority of the time, what you want to do is automate a process.
Before you can determine what actions to take, you must explicitly specify what your success criteria are. What must your company do to prevent spreading incorrect information or generating biases, which would ultimately lead to unfavorable outcomes? It all boils down to the data you’re providing and the caliber of that data when it was provided.
There are two main factors involved in training generative AI:
- The initial training process: Generative AI can and should be used to streamline rule formulation and enhancing data quality. The objective is to train the AI engine to comprehend and develop these rules more effectively by using your organization’s professional knowledge and carefully chosen content.
- Expert tweaking: Next, the results of the AI are refined. After a result has been generated based on a set of inputs, bring in experts who can review and verify the outcome. The expert validates the accuracy of the output produced by the AI. If it’s not accurate, the specialist can supply further information to lead the AI down the right path. When used on a broader scale across numerous businesses or anonymized data, this iterative method accelerates the AI’s learning and enhances the speed and accuracy of its outputs.
Planning for success
Understanding generative AI’s promise and drawbacks is crucial in a digital industry ablaze with its possibilities. This technology has great potential to transform a wide range of industries. But rushing headlong into generative AI without a solid data approach could result in expensive errors and delays. The keys to success are data quality and context. Iteratively improving AI models and creating a solid data foundation are crucial. Enterprises will realize the potential of generative AI with the help of a strategy based on data – and in turn, achieve their business goals.