The Data Governance Hub and Spoke Model: Why it Works
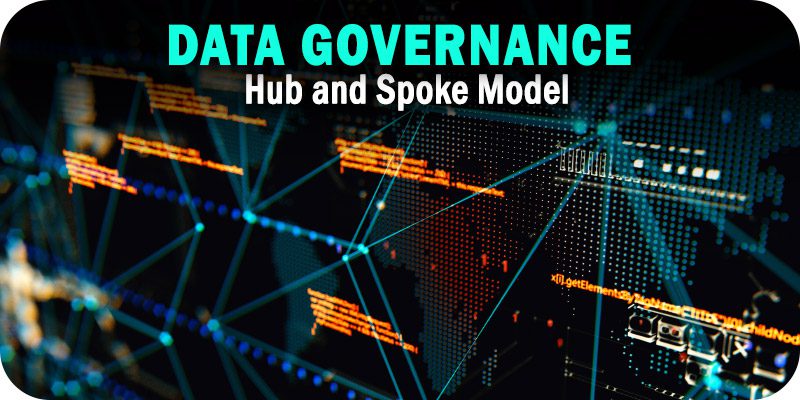
This is part of Solutions Review’s Premium Content Series, a collection of contributed columns written by industry experts in maturing software categories. In this submission, Dataddo Founder and CEO Petr Nemeth offers commentary on why the hub and spoke model of data governance strikes an ideal balance between control and democratization of data.
With data and analytics becoming a core function in today’s business culture, the issue of data governance is more prominent than ever.
Data governance refers to the processes and practices within a company that formally determine how data is stored, distributed, and kept consistent across channels.
While governance policies vary highly from organization to organization, at the heart of every policy is a balance between centralization and decentralization.
Before we introduce the hub and spoke model of data governance, we need to take a closer look at what centralized and decentralized data governance mean.
Centralized vs. Decentralized Data Governance
In traditional models of data governance, data is almost entirely centralized. It’s kept by data teams that feed dashboards to non-technical professionals, who are powerless to modify the source data (or data pipelines) on their own for quick insights. Requests for new information can take anywhere from hours to months to fulfill.
For the most part, these governance models are no longer sustainable, because marketers, salespeople, recruiters, finance experts, and other business-oriented professionals require more analytics flexibility for faster time to insights.
Hence the trend toward decentralization. This is being accelerated by the development of self-service data integration and business intelligence (BI) tools that eliminate the need for data departments to engineer every step of the analytics process. (The market for self-service BI tools is expected to grow at an annual rate of 15.5 percent per year through 2026.)
On one hand, decentralization of analytics competencies gives non-technical actors the quick insights they need to yield better business outcomes.
On the other hand, it brings a storm of questions around data quality and data security. How to ensure that data stays consistent and accurate across all systems? Who is responsible for which data sets? Who should have access to them? Using what tools? And when?
Just like extreme centralization is not practical, neither is extreme decentralization. Best for any organization today will be to find some happy medium between the two. More centralization means more control over data quality and security, but less power from insights and less usability of data at the operational level; more decentralization means the opposite.
The Data Governance Hub and Spoke Model
The optimal data governance policy should strike a balance between centralization and decentralization. But what this looks like in practice is not obvious. One answer is the hub and spoke model.
In the hub and spoke model, data teams can remain in control of data quality and storage, while end users can flexibly manipulate data without breaching security. Here is a diagram to illustrate how this model of governance might be implemented:
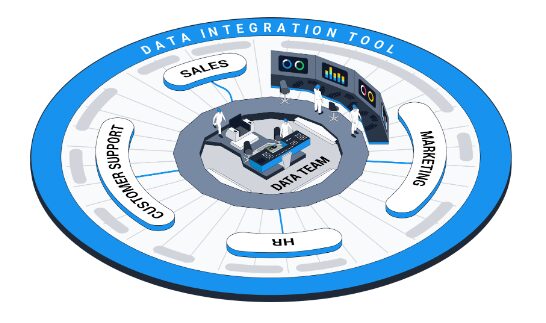
Source: Dataddo
This may look like a diagram of some kind of data architecture. But it’s very important to point out that in a data architecture, hubs and spokes are virtual locations, like data warehouses (hubs) and BI tools or other operational tools (spokes). In data governance, hubs and spokes are competencies.
As you might guess, hub teams (i.e. data teams) have advanced competencies to understand and work with data. These teams include data engineers, data scientists, and sometimes data analysts. They build and maintain data architectures, develop data models, build pipelines, cleanse data, set rights and accountabilities, and—in organizations where governance is more centralized—build dashboards.
Spoke teams (i.e. sales, HR, customer support, marketing) are composed of non-technical professionals like marketers, salespeople, recruiters, and accountants. Depending on the style of data governance, their competencies could range from determining metrics, to viewing dashboards created by hub teams, to actually building and managing pipelines. In a bank, for instance, where data security is paramount, the competencies of spoke teams would be very limited. But in an e-commerce or retail business, their competencies may even overlap with those of the hub teams.
It’s crucial to note that the hub and spoke model of data governance can only be fully achieved with modern, cloud-based data integration tools that support employees at all levels, whether at the center of the hub or at the end of a spoke.
Modern, Cloud-Based Data Integration Tools Enable Spokes
Traditional data integration tools disable spoke teams without technical know-how because they can only be manipulated by engineers and they only feed data to warehouses, which also require technical know-how to access. Plus, they are often on-premise, which puts them at a growing disadvantage in the face of the cloud technology boom (cloud solutions are more flexible, scalable, and cost-effective).
Most modern data integration tools, on the other hand, are friendly to non-technical spoke teams and offer hub teams the full range of integration capabilities. They are cloud-based, making them easily connectable to any data-generating app, data warehouse, or dashboarding application. The capabilities they offer are:
- They feed data to both warehouses and self-service BI platforms (a process known as ETL—extract, transform, load)
This means that they support organizations with stricter governance policies by enabling hub teams to skip the warehouse and send data directly from online services to the dashboards of spoke teams. They also support organizations with more relaxed governance policies by enabling spoke teams to send data from services to dashboards themselves; this is extremely useful in situations that call for quick insights.
During the ETL process, these tools automatically perform light transformations, which is what makes extracted data immediately analyzable in dashboards. Many such tools also allow exclusion of personally identifiable information (PII) from extractions, ensuring an essential standard of security.
- For cases where spoke teams do have sufficient rights and technical know-how, they allow engineers to easily batch replicate high volumes of data from hub storages to secondary spoke storages.
This essentially gives each spoke team its own data sandbox.
- There are now a few tools on the market that enable data to be “activated,” i.e. sent from storages back into the applications that employees at the operational level actually spend most of their time in (data activation is also referred to as “reverse ETL”).
This helps marketing teams automate personalized advertising, and gives departments like sales and customer service a 360-degree view of prospects and customers—without them accessing the central data hub.
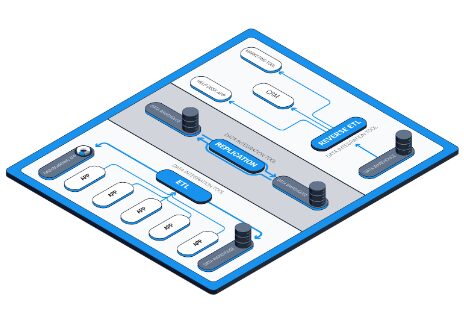
Source: Dataddo
All the above capabilities let hub teams securely democratize access to data, while maintaining a vital standard of quality. They also let non-technical spoke teams manipulate data to the maximum extent within any policy of governance.
Empowering All Organizational Units
Where a company needs to be on the axis between complete centralization and complete decentralization depends on industry regulations and company goals, but in the post-pandemic era of business, every company that uses modern data integration and BI platforms can benefit from the hub and spoke model.
It gives hub teams control where control is necessary, and keeps spoke teams as flexible and self-sufficient as possible.