Empowered by Security Log Data: A Guide to Combatting Cyber Fatigue
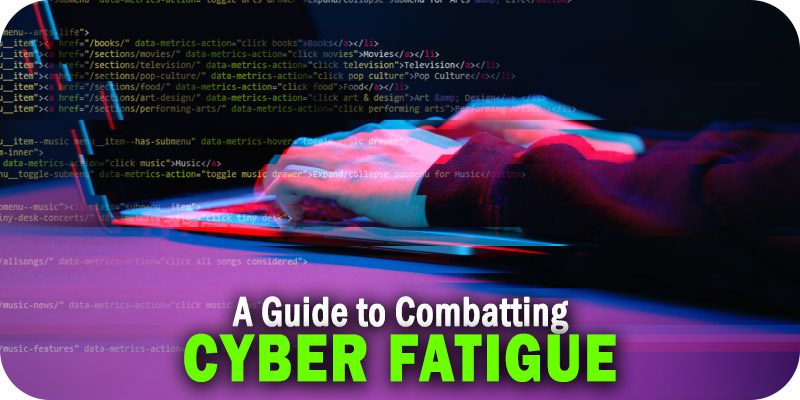
As part of Solutions Review’s Premium Content Series—a collection of contributed columns written by industry experts in maturing software categories— Ozan Unlu of Edge Delta breaks down how security log data can help combat cyber fatigue, and get security teams back into the fight.
A fundamental problem with security log data management at many organizations is effectively balancing a growing avalanche of data coming from a wide range of sources – such as antivirus software, firewalls, intrusion detection, and prevention systems; server operating systems, applications, and more – with a limited quantity of both people and log management resources available to manage it all.
One of the problems with cyber threats is that these threats can lurk anywhere. Organizations understandably want to harness and leverage as much security log data as possible to ensure they’ve got “eyes in all places” However, with all the data being generated, this task is more overwhelming than ever before. In fact, humans (and machines, for that matter) can barely keep their heads above water as the onslaught continues. Combine this with the fact that today’s attackers are more sophisticated and moving faster than ever before, and you have a perfect scenario for security ops teams to feel as if they are shoveling sand against the tide. In fact, it’s estimated that up to 42 percent of companies report growing cyber fatigue (defined as apathy in proactively defending against attacks). We’ve got to empower them to harness and leverage the full breadth of their security log data. But how?
Widget not in any sidebars
Security Log Data: 3 Key Points to Empowering Security Ops Teams
Break Datasets Down into Manageable Chunks All Processing at Once
The way the process has traditionally worked is known as “centralize and analyze,” where all security log data is amassed into a central SIEM and retained as one true copy in one highly secure location, often completely siloed from production environments. By ingesting all this data, a security ops team could ensure they had no blind spots, and the data (all pooled together) became contextually richer. However, in recent years, massively growing data volumes mean this approach is no longer viable from either a time or cost perspective. A better approach involves breaking data sets down into smaller clusters and processing all of them simultaneously and in parallel. This is akin to taking a big, overwhelming project, breaking it into manageable bits, and assigning the pieces to various workers who can work independently and contribute meaningfully to the whole.
Apply Machine Learning at the Source
As noted earlier, one drawback of the “centralize and analyze” approach is the latency it introduces. With hackers moving faster than ever before, most organizations just can’t afford such latency anymore. According to recent statistics, the average “break out” time (the time it takes for an adversary to move laterally from an initially compromised host to another host within the victim environment) is only about an hour and a half, with the fastest hackers moving much more quickly than that, often requiring only a matter of minutes. Given this accelerated speed, it becomes critical to identify suspicious activity as quickly as possible, and applying machine learning simultaneously across the growing number of security log data sources is an absolute must. The benefits to doing this are twofold: One, when security ops teams analyze security log data at its source, they can identify anomalies much faster than forcing various datasets to “wait in line” to enter the SIEM for analysis. Second, when data is analyzed at the source, security ops teams know immediately and automatically the exact source of the anomaly, helping to reduce cyber response times dramatically.
Intelligently Filter Security Log Data
Another drawback of “centralize and analyze” is the fact that it does not acknowledge that all data is not created equal. Security ops teams find themselves running up against data limits and, in many instances exceeding them, hiking up storage costs substantially and often unexpectedly. Keeping an eye on all data is essential. However, a lot of this data will be of lower value, so where it is ultimately relegated for ongoing analysis and retention needs to be carefully considered. For instance, some data can afford to go in lower-cost “cold” retention tiers, while others may belong in a hotter SIEM tier. Intelligently filtering data helps security ops teams avoid the need to make unnecessary compromises. In addition, due to ongoing cost challenges, some security ops teams are forced to make arbitrary decisions on what security log data to include and what to omit, leaving them with obstructed views. With today’s technology, they don’t need to do this– they can have their cake and eat it too.
Conclusion
Every year, cybersecurity threats are escalating both in terms of volume and variety. This, plus the ongoing skills shortage, places a significant strain on cybersecurity teams. It is therefore not surprising that as many as 66 percent of CISOs report feeling unprepared. As part of an overarching cybersecurity strategy, comprehensive security log data can be a handy weapon in the arsenal. Unfortunately, the data is currently being generated at a pace that exceeds both natural human and machine limitations. We need new techniques enabling security ops teams and the resources at their disposal to “work smarter, not harder”– effectively harnessing and leveraging the full breadth of their data stores, and finding novel ways to accomplish this are fast becoming an organizational imperative.
Widget not in any sidebars