The Benefits of On-Premises AI: Regaining Control in the Era of Data Sovereignty
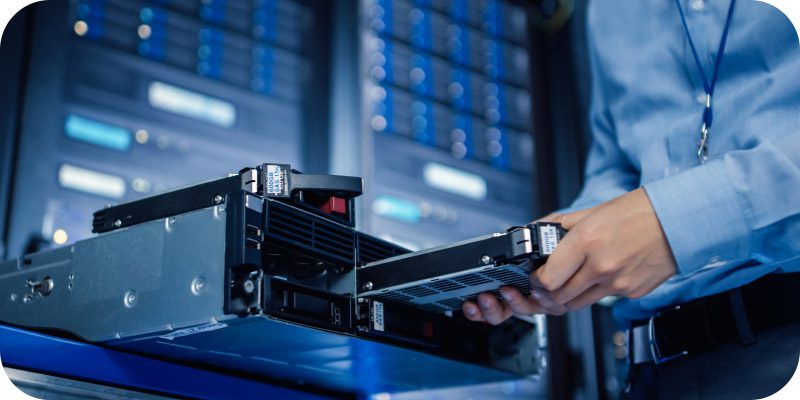
Praveen Jain, the SVP/GM of AI Clusters and Data Center at Juniper Networks, outlines how on-premises AI can help companies regain control in this era of data sovereignty. This article originally appeared in Insight Jam, an enterprise IT community that enables human conversation on AI.
A decade ago, the public cloud promised enterprises greater flexibility and lower costs. Today, many realize the reality is far more complex, and we are witnessing a significant shift back to on-premises solutions, especially for enterprises deploying AI workloads. This shift stems from mounting challenges with public cloud deployments, from unpredictable GPU costs and security vulnerabilities to vendor lock-in concerns. Organizations are increasingly recognizing that the promise of simplified cloud deployments often comes with hidden complexities and costs that can impact long-term success.
To illustrate the optionality, a recent survey found that nearly 50 percent of IT decision-makers are now equally considering both on-premises and public cloud solutions for new applications in 2025, marking a significant departure from the “cloud-first” mindset.
Data Sovereignty and Security: Bringing AI Workloads Home
In today’s digital landscape, where data breaches can easily cost organizations millions, security cannot be an afterthought.
The challenge becomes particularly acute when training large language models (LLMs) using private data in public cloud environments. On-premises AI infrastructure provides organizations with complete control over their security protocols and data governance—a crucial advantage for complying with regulations such as the General Data Protection Regulation (GDPR) and the California Consumer Privacy Act (CCPA). This control extends beyond mere compliance. However, it enables organizations to implement custom security measures that align precisely with risk tolerance and operational requirements.
Consider the financial services sector, where institutions process millions of customer transactions daily. When AI models are trained and deployed on-premises, these organizations maintain full data sovereignty while significantly reducing breach risks due to the more direct visibility into all hardware, software, and in-house security measures. There’s no guesswork, no hoping a third-party provider has things locked down. This autonomy becomes even more critical when considering that GDPR non-compliance fines, for example, typically range from $10M to $22M.
The ability to maintain complete control over sensitive data while running sophisticated AI workloads has become a competitive necessity in heavily regulated industries. However, it’s important to note that on-premises benefits extend beyond data sovereignty alone.
The Economics and Technical Advantages of AI: Cost Efficiency and Control
While short-term projects—like a specific research study or business analysis—might find temporary solace in the lower cost of entry offered by public cloud solutions, the long-term cost implications for AI are often overlooked. The truth is, the substantial recurring costs associated with running resource-intensive GPUs in the cloud quickly add up.
In contrast, private AI data centers, while requiring a more significant upfront investment, ultimately deliver substantial savings in terms of total cost of ownership (TCO) and operational expenditures (OpEx). This economic advantage is further compounded by the technical control gained from on-premises deployments.
In the automotive industry, for instance, companies developing autonomous vehicles are producing massive data volumes, presenting a unique challenge. Original Equipment Manufacturers (OEMs) and their suppliers find that the bandwidth costs alone for moving massive datasets to and from the cloud can be prohibitive. Moreover, these software and interoperable hardware developers require real-time processing capabilities to support critical functions like over-the-air updates and rapid iteration in AI model development. Latency introduced by cloud data transfers can severely hinder these operations.
By deploying on-premises AI infrastructure, automotive companies and OEMs reduce bandwidth costs and gain the necessary control to fine-tune their infrastructure for specific workload requirements. This leads to better cost predictability and often results in lower TCO for sustained AI workloads. Recent analysis finds a 35 percent TCO savings and 70 percent OpEx savings over five years for private AI data centers compared to public cloud offerings, primarily due to the high recurring costs associated with public cloud services.
These advantages extend beyond pure economics, however, as organizations also gain the ability to fine-tune their infrastructure for specific workload requirements, optimize performance for certain AI models, and maintain complete visibility into their entire AI stack.
The Future of AI Infrastructure: Automation and Optimization
Looking ahead, there is little doubt that AI and machine learning are crucial for modern, reliable, and secure end-user experiences, underscoring the importance of optimizing the underlying infrastructure. Modern on-premises solutions are evolving to incorporate advanced capabilities in high-performance networking and GPU clusters, specifically designed for complex tasks like LLM training. The focus is shifting toward automation that directly enhances control and efficiency.
To that end, advancements in automation are being adopted to directly address the need for greater efficiency:
- Automated Resource Scaling: Systems can automatically adjust computing resources based on real-time demand, ensuring optimal performance without manual intervention.
- Intelligent Workload Placement: AI-driven tools can analyze workload requirements and dynamically allocate them to the most efficient resources, maximizing utilization.
- Proactive Performance Maintenance: Automated monitoring and optimization tools maintain consistent performance levels, minimizing downtime and ensuring smooth operations.
Organizations can achieve cloud-like flexibility by focusing on these key automation capabilities while retaining the essential control and security benefits of on-premises AI infrastructure.
The Path to Efficient AI Operations
While cloud services will continue to play a role, on-premises AI infrastructure remains essential for organizations serious about building sustainable, scalable capabilities, particularly those requiring fully optimized data and computing resources. The decision between cloud and on-premises AI infrastructure isn’t just about hardware—it’s all about aligning IT priorities with long-term business objectives and operational realities.
As organizations mature in their AI journey, many are searching for the optimal balance of control, security, and cost predictability to launch large-scale AI deployments efficiently. By opting for on-premises AI infrastructure, organizations can build a strong foundation that keeps their data and workloads secure, compliant, and cost-effective in the long term.