Predictions 2016: On-demand Big Data Virtual Clusters Go Mainstream
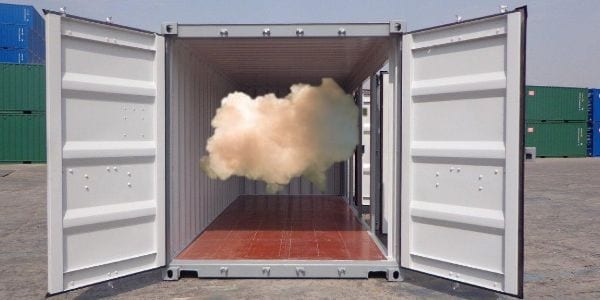
by Sujatha Kashyup, VP of Technology at Robin Systems
The aim of virtualization is to deliver rapid time to value for the end user. The biggest value virtualization has provided to date has been to decouple the application deployment cycle from the hardware procurement cycle. The first wave of virtualization did a remarkable job of consolidating legacy applications. However, the new class of applications making its way from early adopters to mainstream use has largely eschewed virtualization so far. This, of course, is the class of Big Data applications.
They came of age in web-scale giants like Google, Facebook and Twitter where large swaths of scale-out servers were dedicated to a monolithic application. In these extreme-scale deployments, economies of scale could be applied to optimize a single framework by highly-skilled administrators. This justified bare metal deployments.
We predict that 2016 will be the year where a paradigm for virtualizing Big Data infrastructures will become established as part of the journey towards adoption by mainstream enterprises.
There are several drivers for this. Firstly, mainstream enterprises are unlikely to achieve the kind of scale that justifies the use of dedicated hardware clusters for each Big Data framework. Instead, they are more likely to want to experiment with different Big Data frameworks, and construct data pipelines that comprise of multiple such frameworks. Providing hardware multi-tenancy for multiple Big Data frameworks is, therefore, a basic requirement.
Secondly, mainstream enterprises have existing investments in storage systems, and these storage systems and the data contained within need to be integrated into their Big Data applications. This requires storage virtualization and a decoupling of compute from storage.
Thirdly, enterprise users expect to be able to procure on-demand Big Data clusters just as they procure on-demand virtual machines from a centralized IT infrastructure today. This requires compute orchestration frameworks for Big Data applications that insulate the end user from the mundane tasks of installing, deploying and maintaining their virtual clusters.
Finally, because these are inherently distributed scale-out applications, they are expected to be highly elastic. A static binding between creation-time capacity specifications and runtime performance is not a viable strategy. Furthermore, many of these frameworks complete entire jobs within minutes. So, an elasticity strategy based on virtual machines is a non-starter, since virtual machines take several minutes to install, configure and deploy. Containers, which take seconds to deploy, are the natural choice for providing the elasticity required by these applications.
While many enterprises are exploring Docker and other container technologies, production use cases are extremely limited in number. There is much that is lacking in existing container frameworks to make them enterprise-ready. Foremost among these lacking features is a robust storage framework for container-based deployments.
We expect activity to heat up significantly in 2016 in the space of container-native storage technologies that provide enterprise-class storage features such as reliability, scalability, and high performance.
Overall, in 2016, we expect the IT infrastructure landscape to see a paradigm shift where Big Data applications become a “regular” part of the catalog of centralized IT PaaS offerings in mainstream enterprises, just as legacy applications are today.
About the Author: Sujatha Kashyup has led industry leadership benchmark publications for several generations of IBM servers. Resolved critical performance problems at Fortune 500 companies across the globe and created high-performance solutions and proofs of concept to win new customers and/or ecosystem partners. She has spent the past five years working with marquee names in the financial industry to create extreme-performance solutions for high-frequency trading. Sujatha Kashyup holds 11 patents, a Bachelor’s degree in Computer Engineering from the National Institute of Technology Karnataka and a Doctorate from the University of Texas, Austin in Computer Engineering.
Widget not in any sidebars