Cloud Data Warehouse Types, Benefits and Limitations: A User’s Guide
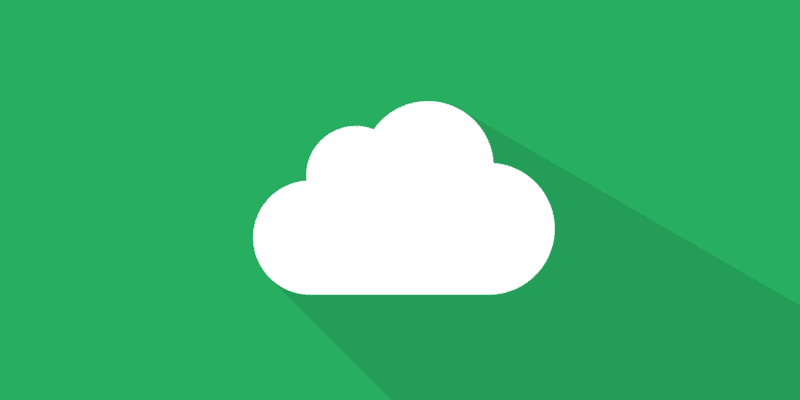
Enterprises across every industry are going through a massive digital transformation aimed at increasing their efficiency and their ability to innovate. This is achieved, in part, by moving workloads to the cloud – and data infrastructure, including cloud data warehouse types, are no exception. If data is the new oil, data warehouses are the refineries that enable them to refine that crude data and transform it into something usable and valuable with broad applicability.
Since their inception almost 50 years ago, the way in which data warehouses are used has changed drastically and many enterprises are looking to the data warehouse to maximize their data’s potential by hosting data from a wide variety of sources, and by answering complex queries in real-time for business and technical users. Today’s users of the data warehouse expect to be able to do their own data discovery and ad-hoc querying and are no longer satisfied by a set of pre-defined reports and dashboards provided to them by IT. In following this seismic industry shift, data warehouses have been re-imagined from their original function as on-premise workhorses, to agile, scalable, flexible solutions in the cloud.
The data management and analytics market has experienced three notable generations of cloud data warehouses: data warehouse software that leverages cloud infrastructure; cloud-native, fully managed services; and now, hybrid, multi-cloud solutions. But what defines each of these generations, and what does this mean for the industry today?
Where have we been? Data warehousing deployed in the cloud
The first generation of cloud data warehouses, typified by Amazon Redshift, was little more than data warehousing software delivered in the cloud. It simplified the deployment model for data warehousing software and provided a better value equation than on-premise solutions by leveraging cloud infrastructure, but there was little innovation in what was delivered.
Benefits: The first-generation provided many benefits beyond the simplicity of deployment – they could, in theory, scale up and down as business needs changed, they were part of an ecosystem of data integration and application development which enabled building new classes of applications, and they were built on a platform designed for resiliency and security. These early data warehouses removed the complexities of setting up sophisticated infrastructure required to support a clustered MPP data warehouse, and not only was the hardware and operating system environment pre-configured, but so was the data warehouse itself. Technologies such as Amazon Redshift were heralded as changing the way data warehouses would be deployed in the future and adoption grew rapidly – however, noticeable limitations surfaced.
Limitations: While this first generation was a trailblazer for its time, these data warehouses were descended from on-premise technologies, not designed to perform at cloud scale, and were inherently limited. The first generation couldn’t handle the demanding queries and diverse workloads of modern IT, which resulted in slow performance and high maintenance – requiring the same teams of people to manage, monitor and maintain the environment as was required for their on-premise cousins.
First-generation cloud data warehouse deployments were typically limited to the vendor’s own cloud platform (e.g. RedShift is limited to AWS and BigQuery is limited to Google Cloud Platform). Since the first-generation cloud data warehouses provided only a cloud version, adopters of this technology needed to find an alternative technology solution for data that resided on-premise. The promise of integration to other related services came with a price and plumbing them together proved to be difficult and time consuming.
These cloud data warehouses were typically restricted in elasticity and didn’t provide true cloud economics, such as the ability to only pay for what you needed. Once you subscribed to the service, the underlying cloud infrastructure was typically always on, so the cost added up quickly whether the solution was in use or not. While pricing started low with these solutions, production workloads at scale could get expensive quickly.
Where are we now? Cloud-native, fully managed services
The second generation of data warehouses are cloud-native, fully managed services that are architected for cloud infrastructure and are typified by technologies like Snowflake. They address many of the limitations of the first generation and introduce flexibility and improved functionality to the market.
Benefits: This era of cloud data warehouses have all the benefits of cloud infrastructure such as scalability, elasticity, and security, but they’re purpose-built for the cloud and are not tied to a single provider in the same way that the first-generation warehouses were. Snowflake’s core offering for example, provides a cloud-native, fully managed cloud data warehouse, which has improved on-demand elasticity that cloud consumers expect, as well as the ability to pay only for the resources being used. With this solution, the underlying cloud infrastructure is inherently hidden from the user. These cloud data warehouses, a generation defined by Snowflake and similar services, has changed the economics of enterprise data warehouses forever.
Limitations: Second-generation data warehouses address some of the shortcomings of the first generation, e.g. costly infrastructure, limited elasticity/inability to scale, but are still fraught with limitations – underscoring the need to move modern enterprises away from past and present and toward the future of cloud data warehouse solutions. Cloud-native, as the name suggests, means that a second technology needs to be selected to meet on-premise analytics needs. Additionally, their costs start low but rise quickly as additional compute clusters are spun up to meet growing user needs.
Where are we going? Hybrid, multi-cloud solutions
Third-generation cloud data warehouses are the future of data management and analytics, and enterprises are beginning to make the transition to these hybrid, multi-cloud solutions to harness all the capabilities from past generations, with a host of added benefits.
Benefits: One of the primary differentiators of the third-generation cloud data warehouse is its hybrid capability – allowing data to be simultaneously managed and analyzed on-premise and in public clouds, connecting all data to the broader data ecosystem regardless of location, and allowing organizations to leverage the real-time insights provided by incorporating all of their data.
Hybrid capabilities address one critical requirement for many organizations that was not met with past generations – an on-premise equivalent to the cloud solution that enables the same technologies, skills, and applications to be run in the cloud and on-premise for sensitive data management and analytics. This addresses the demand that industries with regulatory compliance requirements, such as financial services, healthcare and pharma have around their sensitive data. These enterprises want to leverage the same technologies for their on-premises and cloud analytics needs and be able to write applications that seamlessly join on-premise and cloud-resident data. The third generation is designed to be a component in a broader cloud strategy and is delivered with integration with hundreds of data sources including popular SaaS solutions like Salesforce, NetSuite, Workday and ServiceNow, so data from those services can be seamlessly blended to provide 360-degree insights.
Another key benefit of the hybrid, multi-cloud data warehouse is that it allows for superior scalability and can handle larger data volumes, greater query complexity and substantial user concurrency. This means organizations can truly harness the data across every business function at scale with enterprise-grade reliability and security. The third-generation solutions were designed for top tier enterprises that need to accommodate hundreds of users querying data in parallel and require real-time insights. Having concurrency capabilities, without costs scaling as users are provided with access to the data, means that organizations can truly harness the data across every business function at scale to provide actionable insights.
One of the most inconvenient qualities of previous data warehouse generations was the cost associated with managing and maintaining two separate data warehousing environments. Future solutions will allow use of the same skills, technology, and applications for both cloud and on-premise deployment, while greatly reducing the staff required to administer hybrid deployments. In addition, they are designed for simplicity and incorporate things like automated indexing which negates the need for expensive database tuning experts to create materialized views, cubes, and other complex database mechanisms that need to be managed and maintained.
The onset of new generations of data warehouses underscores how quickly and constantly the industry is evolving in response to market demands, and how enterprises are changing the way they operate. Third-generation hybrid, multi-cloud data warehouses go above and beyond the call to allow for a seamless, autonomous, economical, secure, and scalable environment that lets organizations focus less on administration and maintenance and more on innovation and business differentiation.
By Emma McGrattan
Emma McGrattan is the SVP of Engineering at Actian, and leads research and development for the company’s Hybrid Data Analytics portfolio. A recognized authority in DBMS and big data technologies, Emma is a sought-after speaker at industry conferences. She has recently celebrated 25 years in Ingres and Actian Engineering as well. Emma was educated in Ireland and holds a Bachelors of Electrical Engineering degree from Dublin City University.