What’s the Difference Between Data Quality and Data Governance?
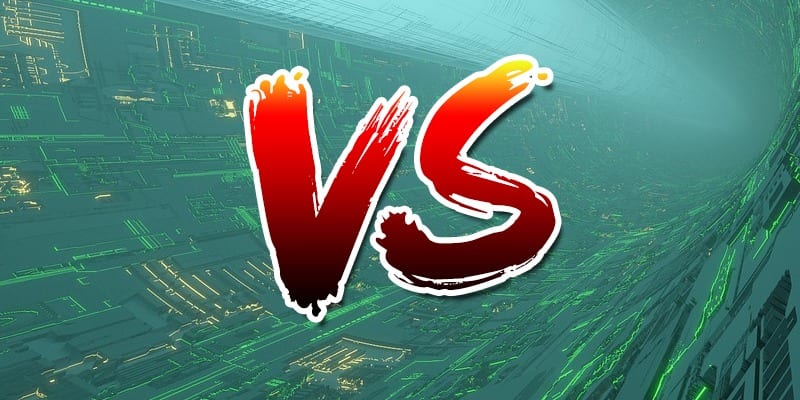
Keeping up with the endless of barrage of technology jargon can be a difficult task. Loosely-defined terms and industry-specific vernacular muddy the waters even further. When it comes to data management in particular, it seems like many words are used interchangeably as well, with data quality and data governance a prime example. It’s not the only one, and it sure won’t be the last, but properly setting the boundaries where on term begins and another ends has real-world implications.
That’s where Solutions Review comes in. Our editors scour the web on a daily basis to bring you the leading library of content. This concept comparison post is one in a long line we encourage you to read, and it is our hope that these resources help you gain a better understand of the space. In this post we feature data quality and data governance in an attempt to remove the signal from the noise. Data management is a broad umbrella term that we see often, but it encompasses many key concept, and the market is expanding in such a way that explainers like this can be of assistance.
The data management industry has spawned several brand new technology categories in recent years. Data quality and data governance are perhaps the two most prominent. Although not linear in functional use, the topics are closely related and play major roles during the analytic process. The newfound importance of these topics has seen the rise of dedicated technology vendors looking to help solve these problems, which is a recent phenomenon as well.
An Introduction to Data Quality Tools
Data quality tools aim to help businesses keep their data clean and uncorrupted, so that a data warehouse or data analytics tool can properly analyze it. A company’s data quality can degenerate if the data is not regularly monitored over time.
Data quality pertains to the overall utility of data inside an organization, and is an essential characteristic that determines whether data can be used in the decision-making process. Data quality solutions are typically built atop features that allow businesses to match, clean, correct, validate, and transform data so that it can be analyzed by a database, data warehouse, or analytics system.
Depending on data use, keeping enterprise data clean and healthy is necessary to boost an analyst’s reporting or help with a new product release. However, to be sustainable in the long-term, data quality tools need to be able to support data management beyond standard data cleansing methods. Data quality tools need to extend sophisticated data processing across a company’s entire data management system.
As regulations grow stricter and compliance frameworks become more common, organizations will grow increasingly interested in dedicated data quality software, ensuring that they are keeping their data in a way that safeguards it from internal and external threats. Perhaps the most important use case for data management in the next five years will be Europe’s new General Data Protection Regulation (GDPR).
What is Data Governance?
Not only is data governance one of the most common data management use cases, it’s also the most difficult to solve for. Data governance is perhaps the most important factor in modern data management, and bridges the gap between data quality and democratization. In order for organizations to enable cross-enterprise data access (which is a major pain point in and of itself), data needs to be overseen in the correct fashion using industry-standard best practices.
While many of the industry’s best data management platforms offer capabilities that support data governance, the process really is up to the organization. Data governance is commonly made up of a set of frameworks developed to ensure consistent and quality data. Implementing a governance procedure often involves defining data stewardship roles as well. Those in these positions then decide how data will be stored and protected, and are to follow a strict set of guidelines to do so
More than 50 percent of organizations claim data governance is their top cloud data management challenge, according to new research. The overall theme seems be that cloud data warehousing is enabling organizations to achieve better performance, but trouble implementing new technologies and protocols (governance) remains a real challenge to organizations that don’t utilize all the best practices at their disposal.
Complexity too remains a major issue, as organizations are increasingly expressing an interest for in-memory processing to support both structured and unstructured data. Data access too has to be governed, and represents yet another pain point for modern organizations. Integrating data across multiple sources brings both data quality and data governance pain points, as well as getting data into the data warehouse. These needs can often be mitigated by deploying data integration middleware, but also bring to light the fact that cloud data warehouses must accommodate a variety of data types and serve a range of use cases.
The Bottom Line
Organizations should utilize data quality techniques as part a larger data governance strategy. Clean, actionable and ready-to-use data is a key part to the analytic process, especially if you are executing accurate and meaningful results. Users can take advantage of dedicated (or data preparation) software to help them during the data quality process, but data governance is a strategic methodology for executing authority over a collection of data.