The Link Between Customer Data Platforms, Machine Learning, and Scaling Customer Experiences
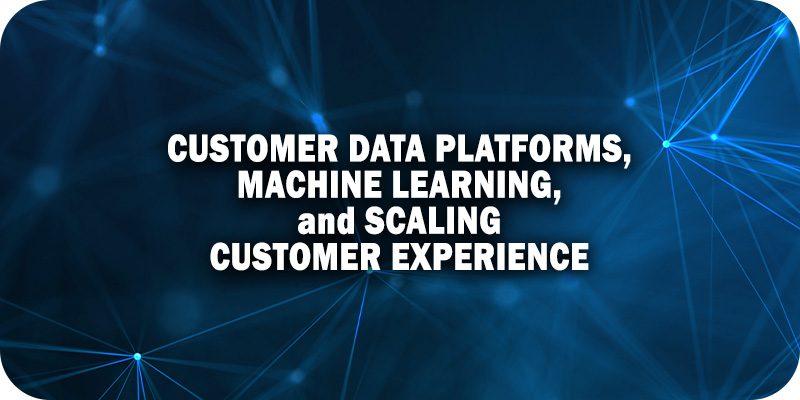
As part of Solutions Review’s Contributed Content Series—a collection of contributed columns written by industry experts in maturing software categories—Arnaud Prades, the Director of Machine Learning at Acquia, outlines the links between customer data platforms (CDPs), machine learning, and scaling customer experiences.
Over the past decade, customer data platforms (CPDs) and machine learning (ML) have become integral components of creating customer experiences at scale. A CDP is the starting point of orchestrating the entire customer journey, and at its core, it should be the right data to activate the right content for the right customer through the right channel at the right time. Providing an exceptional experience at scale relies on a lot of information about a customer and being able to identify “what, where, and why” outcomes—that is where machine learning comes in. It provides the capability to see thousands of data points about a single individual’s engagement. It enables predictive analytics, personalization, and the ability to create more unique customer experiences at scale.
How Machine Learning Works Within a CDP
There are three main steps to how machine learning works within a CDP. The first step is to capture and collect data points that will be inputted into the CDP. Once the data collection is configured, set the goals of the machine learning algorithm. The algorithm will then analyze the data points it has collected, leveraging different techniques to predict customer behavior outcomes. It can predict customer behavior patterns, such as their likelihood to buy, pay full price, or engage with particular content, giving marketers an advantage in acquiring and retaining customers.
When considering the various use cases of a CDP, a range of machine learning techniques is needed to be efficient, especially at scale. For example, suppose the goal is to recommend products, create customer segments based on demographics, or determine when to email a customer. In that case, each requires a different machine-learning model.
Enabling Customer Experience at Scale
Think about it this way. The CDP is the proverbial brain of your customer experience; the data points you input are the signals it receives to make decisions. A CDP relies heavily on data to craft a complex and unique customer experience, increasing customer trust, and fostering customer loyalty. When we further prioritize the customer experience, we promote accuracy through data cleanliness and dispel any inconsistencies that can adversely affect the CX. Furthermore, the CDP needs to be connected to other integrated components—the so-called arms and legs—to act on decisions optimized by machine learning.
CDPs range in capabilities, and the size of your operation should determine the type of CDP you need. Look at the benefits and disadvantages of a “1-click” solution versus a more flexible/configurable one. An out-of-the-box solution may be easier for a marketer to manage but can also have drawbacks. One of them is the chance of ending up with a “black box” with no transparency into how the predictive analytics were derived. Without the technical expertise to challenge the result, your action may be misleading. Ultimately, a complete CDP should address every component.
Beyond predicting consumer behaviors, machine learning capabilities within CDPs allow for automating and streamlining workflows and routine processes. With the power to analyze numerous data points, machine learning can provide real-time recommendations through a CDP that enables teams to modify their go-to-market approach.
Furthermore, machine learning drives marketing teams to work efficiently, craft data-driven insights, and create unique customer experiences. Over time, more regulations will be placed at the forefront, implementing stricter requirements for CDPs. Features like composability and machine learning technologies will continue to advance, enabling more personalized customer experiences while balancing data governance and privacy.