The Data Engineer’s Dilemma: Turning Investment Into Innovation
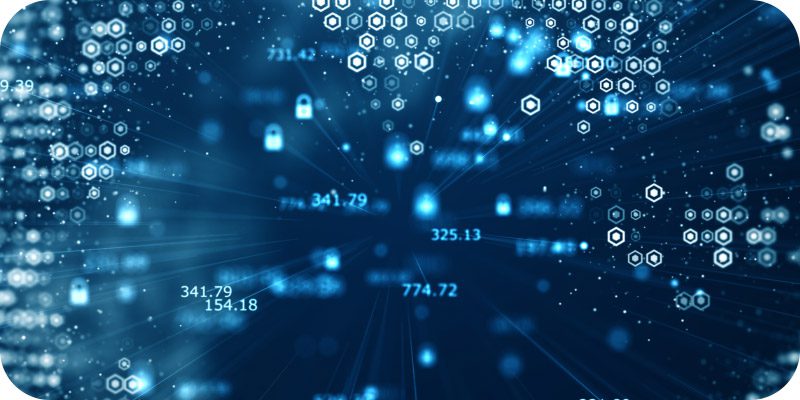
The Modern Data Company’s Srujan Akula offers insights into the data engineer’s dilemma and turning investment into innovation. This article originally appeared on Solutions Review’s Insight Jam, an enterprise IT community enabling the human conversation on AI.
With data and AI budgets at all-time highs, there’s an unprecedented focus on enterprise data management. This attention exposes a critical challenge: despite massive investments, data engineers, whose role should center on enabling data-driven innovation, are increasingly buried in basic data management tasks. They’re spending 70-80 percent of their time maintaining pipelines, cleaning data, and handling governance rather than creating business value. It’s like building a chef’s kitchen and spending all your time organizing ingredients rather than cooking.
The misalignment between investment and impact is particularly evident in AI initiatives, where organizations continue to struggle with ROI. The challenge isn’t a lack of data or technology–it’s that our approach to data engineering hasn’t evolved to meet the demands of the AI era. We need to fundamentally shift from merely managing data to activating it for business value.
The pressure to transform the data engineer’s role has never been higher. As AI shifts from a “nice to have” to a business necessity, companies can’t afford to have their data engineers stuck in an endless cycle of data management and cleanup. However, freeing them from these tasks requires a fundamental rethinking of how we work with data.
The strategic shift to data products represents one of the most promising solutions to this challenge. By treating data as a product rather than a resource to be endlessly processed, we can automate and standardize many of the tasks that currently consume data engineers’ time. Each data product combines the data itself with built-in governance controls, quality metrics, and clear context about its meaning and use, turning data into assets that can be applied to plug-and-play solutions that business teams can activate immediately – whether for dashboards, AI models, or new applications.
Accelerating AI Development
This transformation is particularly crucial for AI initiatives. Traditional data bottlenecks have consistently held back AI scaling efforts, with data engineers spending more time preparing data for models than actually building and optimizing AI solutions. When data products provide consistently high-quality, governed data ready for AI model training, everything changes. Data engineers can shift their focus from data preparation to AI innovation, working on model architecture, optimization, and strategic implementation.
The other AI challenge data products can help address is governance. Questions like: “Can I trust this data? If I run the model, will it try to access things it shouldn’t? Is it compliant and secure?” Providing clean, trusted data for model training and rapid iteration is particularly important for AI initiatives, and data products remove that guesswork. They provide a foundation that maintains consistent data quality, accelerates model deployment, and supports monitoring – which in turn, will help companies scale AI implementation.
Learning from Software Engineering
This evolution mirrors the transformation that happened in software development. While software engineering has fully embraced agile methodologies, robust collaboration tools, and reusable components, data engineering often remains stuck in a project-by-project mindset. By treating data more like software – with reusable, well-documented, and maintained data products – we can free data engineers to focus on innovation rather than maintenance.
A Role In Transition
The impact of this transformation is already becoming clear: organizations implementing data products are seeing up to 90 percent faster deployment of new data use cases, according to McKinsey. But the real transformation goes beyond speed. It’s about fundamentally changing the role of data engineers from maintainers to innovators.
The data engineer should be able to focus on higher-value tasks, such as data architecture, AI strategy and building data products for business use. With the increase in data product adoption, we’ll start to see more data engineers acting in a new role–the “Data Product Owner.” Data engineers can support defining data products, as well as building and launching them into areas business users can then manipulate. In the same way a mobile application is not a one-and-done, and still requires management and software updates, data products are also living, breathing assets that will need to be supported like any other software product.
We’ll start to see data engineers take over that lifecycle management, adding new elements, data or governance considerations as needs arise. Data engineers will also take on a larger leadership role in driving the GenAI strategy, collaborating between business and technical counterparts, to design the right data products that empower business self-service around AI.
As AI and machine learning become central to business strategy, this evolution couldn’t come at a more critical time. Organizations desperately need their data engineers to lead AI innovation, not just maintain infrastructure. Data products are finally giving them the tools to do exactly that – to shift from data operations to data value creation, from pipeline builders to business transformers.
This isn’t just another technological shift; it’s a fundamental reimagining of the data engineer’s role. In a world where AI innovation is increasingly crucial to business success, we need to free our data engineers to focus on what matters most: turning data into business value. The future of data engineering isn’t in managing data – it’s in activating it to transform the business.